Yang Gao
Paper download is intended for registered attendees only, and is
subjected to the IEEE Copyright Policy. Any other use is strongly forbidden.
Papers from this author
Hierarchical Routing Mixture of Experts
Wenbo Zhao, Yang Gao, Shahan Ali Memon, Bhiksha Raj, Rita Singh
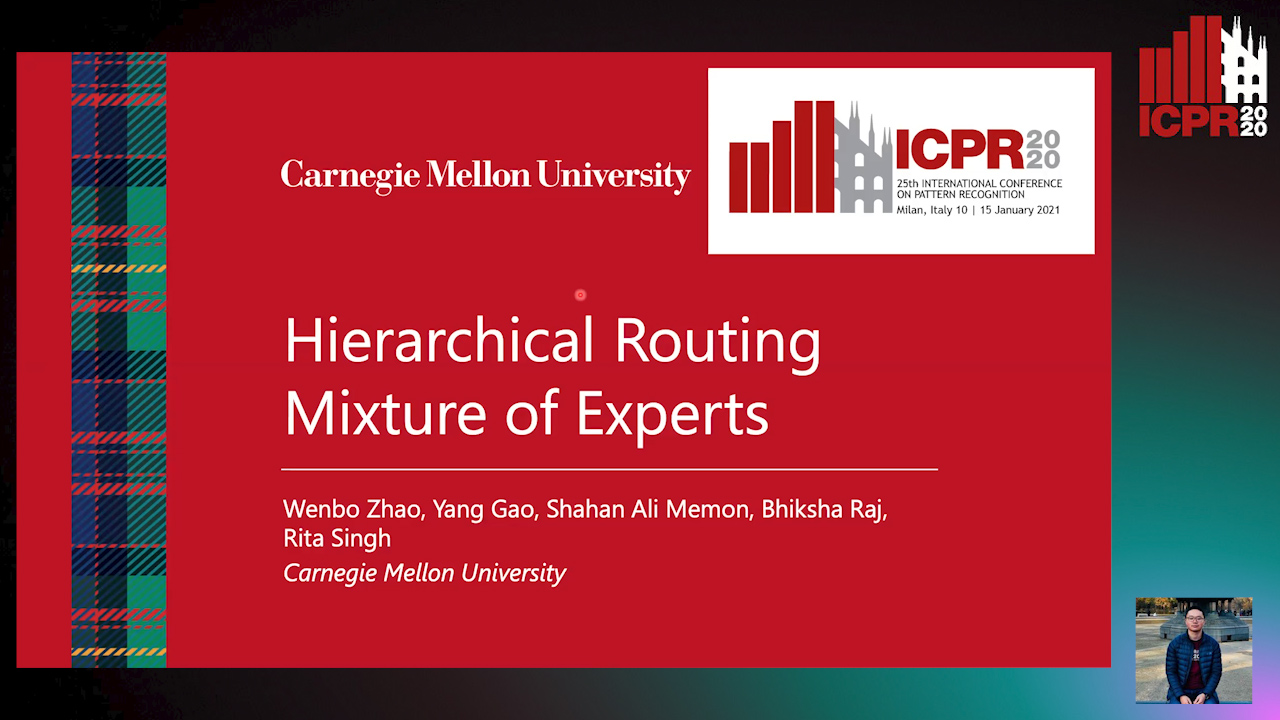
Auto-TLDR; A Binary Tree-structured Hierarchical Routing Mixture of Experts for Regression
Abstract Slides Poster Similar
In regression tasks the distribution of the data is often too complex to be fitted by a single model. In contrast, partition-based models are developed where data is divided and fitted by local models. These models partition the input space and do not leverage the input-output dependency of multimodal-distributed data, and strong local models are needed to make good predictions. Addressing these problems, we propose a binary tree-structured hierarchical routing mixture of experts (HRME) model that has classifiers as non-leaf node experts and simple regression models as leaf node experts. The classifier nodes jointly soft-partition the input-output space based on the natural separateness of multimodal data. This enables simple leaf experts to be effective for prediction. Further, we develop a probabilistic framework for the HRME model, and propose a recursive Expectation-Maximization (EM) based algorithm to learn both the tree structure and the expert models. Experiments on a collection of regression tasks validate the effectiveness of our method compared to a variety of other regression models.