Xia Zhu
Paper download is intended for registered attendees only, and is
subjected to the IEEE Copyright Policy. Any other use is strongly forbidden.
Papers from this author
MFPP: Morphological Fragmental Perturbation Pyramid for Black-Box Model Explanations
Qing Yang, Xia Zhu, Jong-Kae Fwu, Yun Ye, Ganmei You, Yuan Zhu
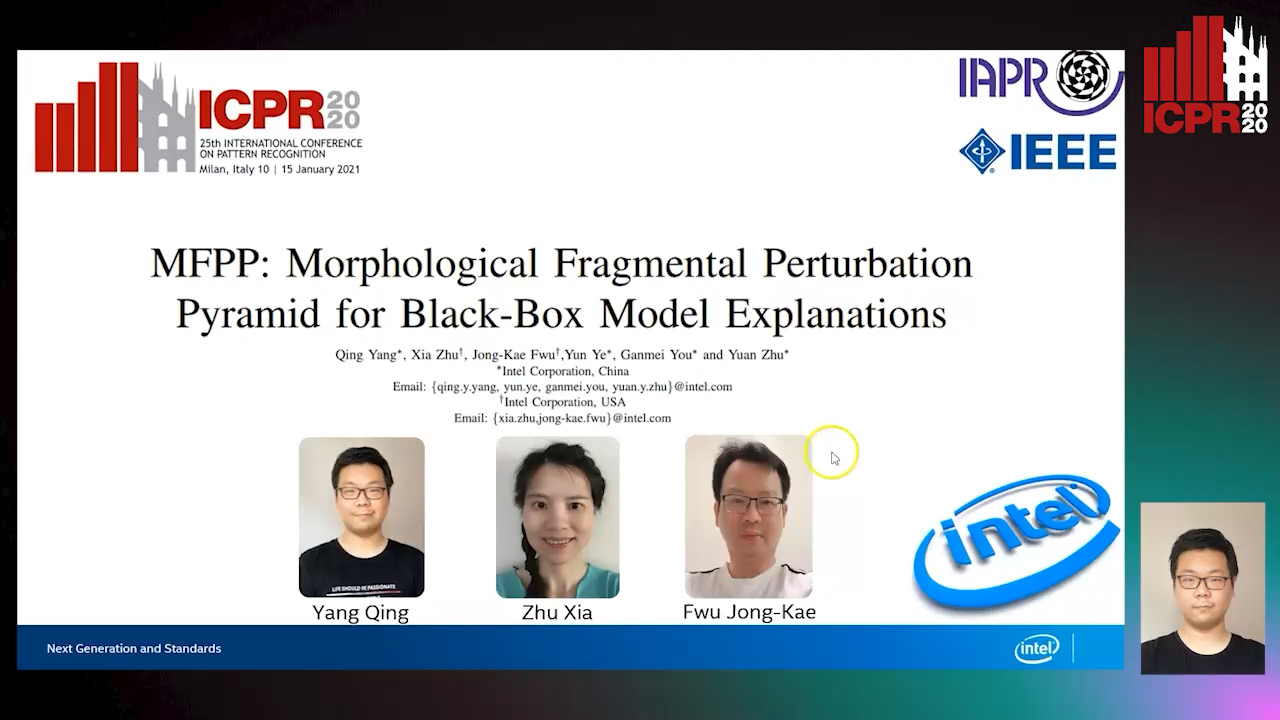
Auto-TLDR; Morphological Fragmental Perturbation Pyramid for Explainable Deep Neural Network
Abstract Slides Poster Similar
Deep neural networks (DNNs) have recently been applied and used in many advanced and diverse tasks, such as medical diagnosis, automatic driving, etc. Due to the lack of transparency of the deep models, DNNs are often criticized for their prediction that cannot be explainable by human. In this paper, we propose a novel Morphological Fragmental Perturbation Pyramid (MFPP) method to solve the Explainable AI problem. In particular, we focus on the black-box scheme, which can identify the input area responsible for the output of the DNN without having to understand the internal architecture of the DNN. In the MFPP method, we divide the input image into multi-scale fragments and randomly mask out fragments as perturbation to generate a saliency map, which indicates the significance of each pixel for the prediction result of the black box model. Compared with the existing input sampling perturbation method, the pyramid structure fragment has proved to be more effective. It can better explore the morphological information of the input image to match its semantic information, and does not need any value inside the DNN. We qualitatively and quantitatively prove that MFPP meets and exceeds the performance of state-of-the-art (SOTA) black-box interpretation method on multiple DNN models and datasets.