Shuai Yin
Paper download is intended for registered attendees only, and is
subjected to the IEEE Copyright Policy. Any other use is strongly forbidden.
Papers from this author
Double Manifolds Regularized Non-Negative Matrix Factorization for Data Representation
Jipeng Guo, Shuai Yin, Yanfeng Sun, Yongli Hu
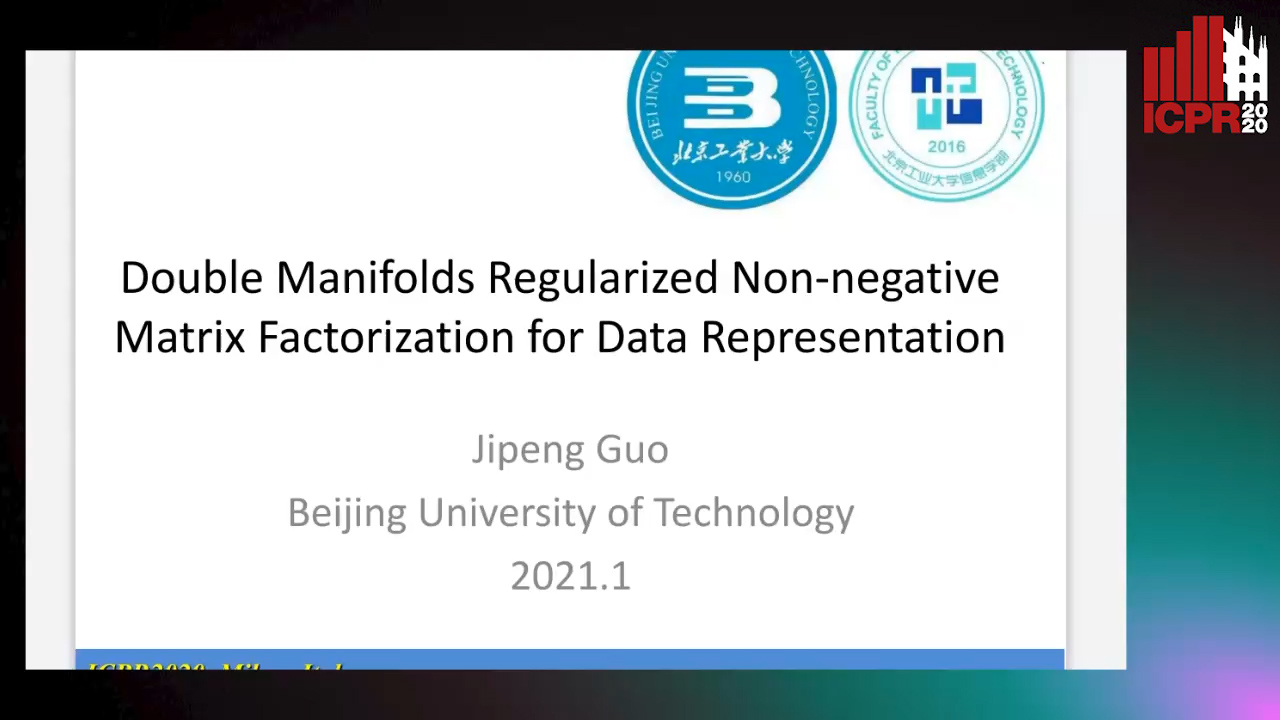
Auto-TLDR; Double Manifolds Regularized Non-negative Matrix Factorization for Clustering
Abstract Slides Poster Similar
Non-negative matrix factorization (NMF) is an important method in learning latent data representation. The local geometrical structure can make the learned representation more effectively and significantly improve the performance of NMF. However, most of existing graph-based learning methods are determined by a predefined similarity graph which may be not optimal for specific tasks. To solve the above the problem, we propose the Double Manifolds Regularized NMF (DMR-NMF) model which jointly learns an adaptive affinity matrix with the non-negative matrix factorization. The learned affinity matrix can guide the NMF to fit the clustering task. Moreover, we develop the iterative updating optimization schemes for DMR-NMF, and provide the strict convergence proof of our optimization strategy. Empirical experiments on four different real-world data sets demonstrate the state-of-the-art performance of DMR-NMF in comparison with the other related algorithms.