Tijl De Bie
Paper download is intended for registered attendees only, and is
subjected to the IEEE Copyright Policy. Any other use is strongly forbidden.
Papers from this author
Graph Approximations to Geodesics on Metric Graphs
Robin Vandaele, Yvan Saeys, Tijl De Bie
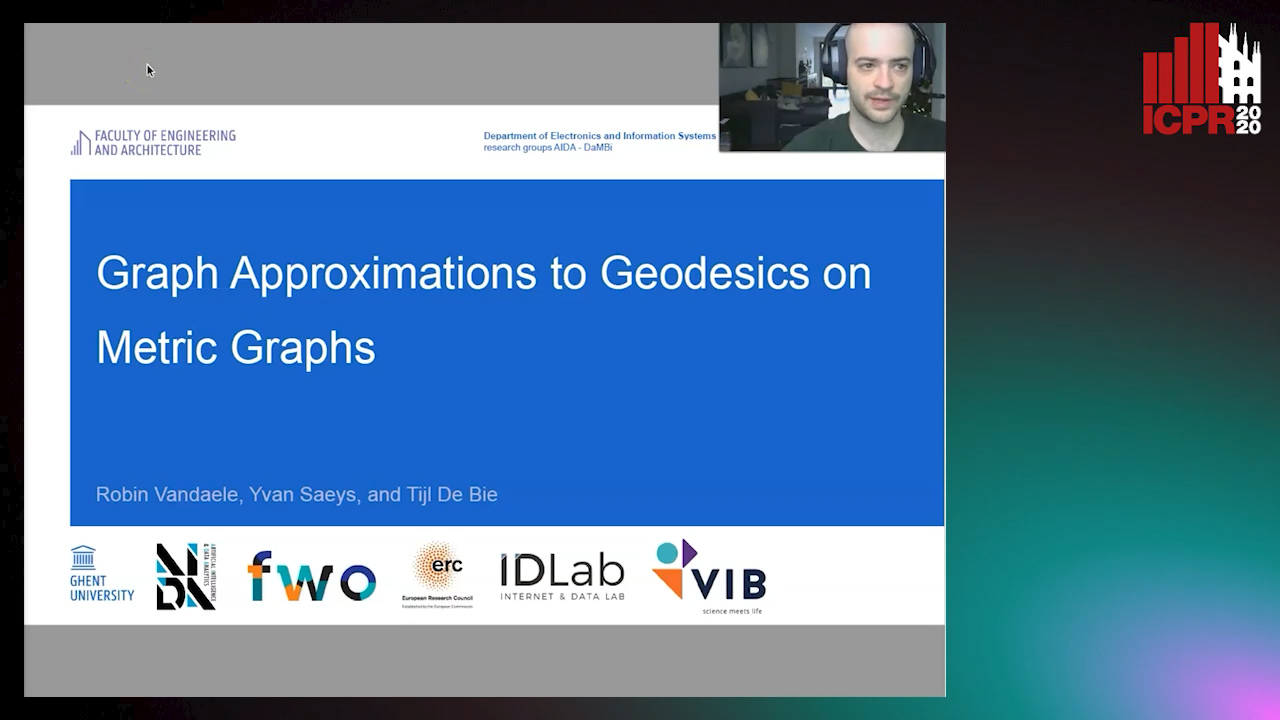
Auto-TLDR; Topological Pattern Recognition of Metric Graphs Using Proximity Graphs
Abstract Slides Poster Similar
In machine learning, high-dimensional point clouds are often assumed to be sampled from a topological space of which the intrinsic dimension is significantly lower than the representation dimension. Proximity graphs, such as the Rips graph or kNN graph, are often used as an intermediate representation to learn or visualize topological and geometrical properties of this space. The key idea behind this approach is that distances on the graph preserve the geodesic distances on the unknown space well, and as such, can be used to infer local and global geometric patterns of this space. Prior results provide us with conditions under which these distances are well-preserved for geodesically convex, smooth, compact manifolds. Yet, proximity graphs are ideal representations for a much broader class of spaces, such as metric graphs, i.e., graphs embedded in the Euclidean space. It turns out—as proven in this paper—that these existing conditions cannot be straightforwardly adapted to these spaces. In this work, we provide novel, flexible, and insightful characteristics and results for topological pattern recognition of metric graphs to bridge this gap.