Omar Ali Sheikh-Omar
Paper download is intended for registered attendees only, and is
subjected to the IEEE Copyright Policy. Any other use is strongly forbidden.
Papers from this author
Supervised Domain Adaptation Using Graph Embedding
Lukas Hedegaard, Omar Ali Sheikh-Omar, Alexandros Iosifidis
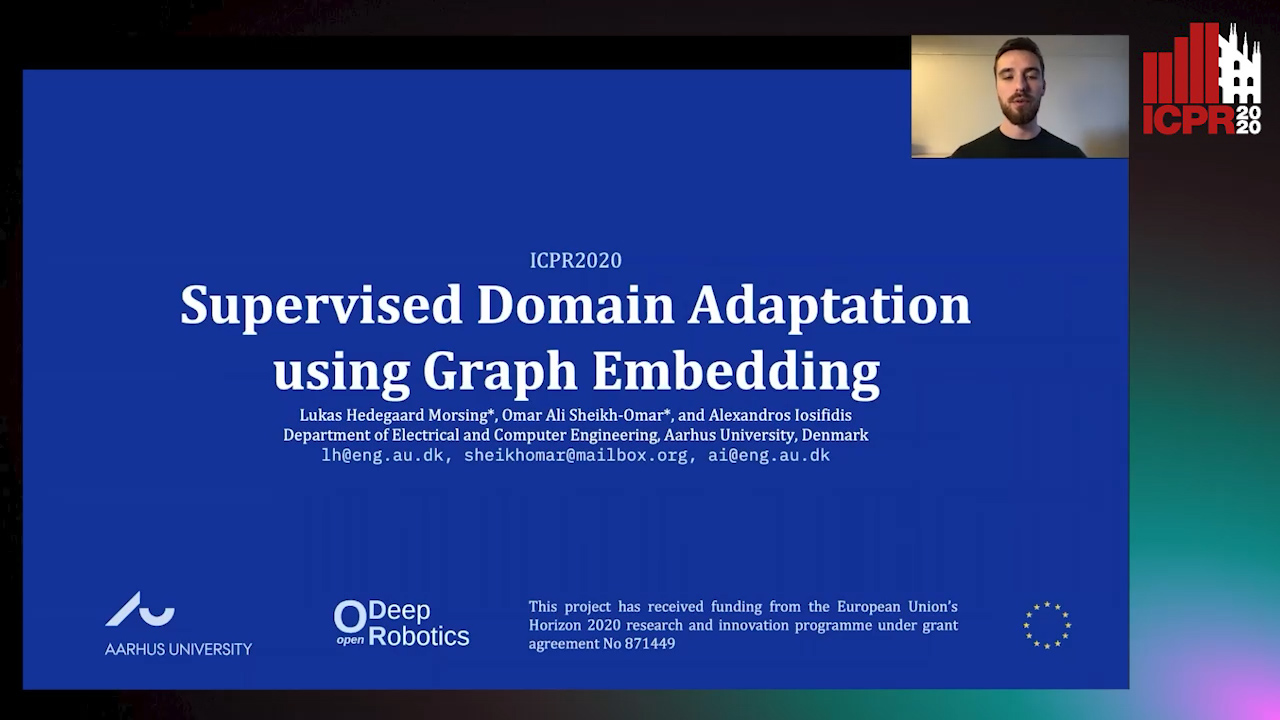
Auto-TLDR; Domain Adaptation from the Perspective of Multi-view Graph Embedding and Dimensionality Reduction
Abstract Slides Poster Similar
Getting deep convolutional neural networks to perform well requires a large amount of training data. When the available labelled data is small, it is often beneficial to use transfer learning to leverage a related larger dataset (source) in order to improve the performance on the small dataset (target). Among the transfer learning approaches, domain adaptation methods assume that distributions between the two domains are shifted and attempt to realign them. In this paper, we consider the domain adaptation problem from the perspective of multi-view graph embedding and dimensionality reduction. Instead of solving the generalised eigenvalue problem to perform the embedding, we formulate the graph-preserving criterion as loss in the neural network and learn a domain-invariant feature transformation in an end-to-end fashion. We show that the proposed approach leads to a powerful Domain Adaptation framework which generalises the prior methods CCSA and d-SNE, and enables simple and effective loss designs; an LDA-inspired instantiation of the framework leads to performance on par with the state-of-the-art on the most widely used Domain Adaptation benchmarks, Office31 and MNIST to USPS datasets.