Renshu Gu
Paper download is intended for registered attendees only, and is
subjected to the IEEE Copyright Policy. Any other use is strongly forbidden.
Papers from this author
Exploring Severe Occlusion: Multi-Person 3D Pose Estimation with Gated Convolution
Renshu Gu, Gaoang Wang, Jenq-Neng Hwang
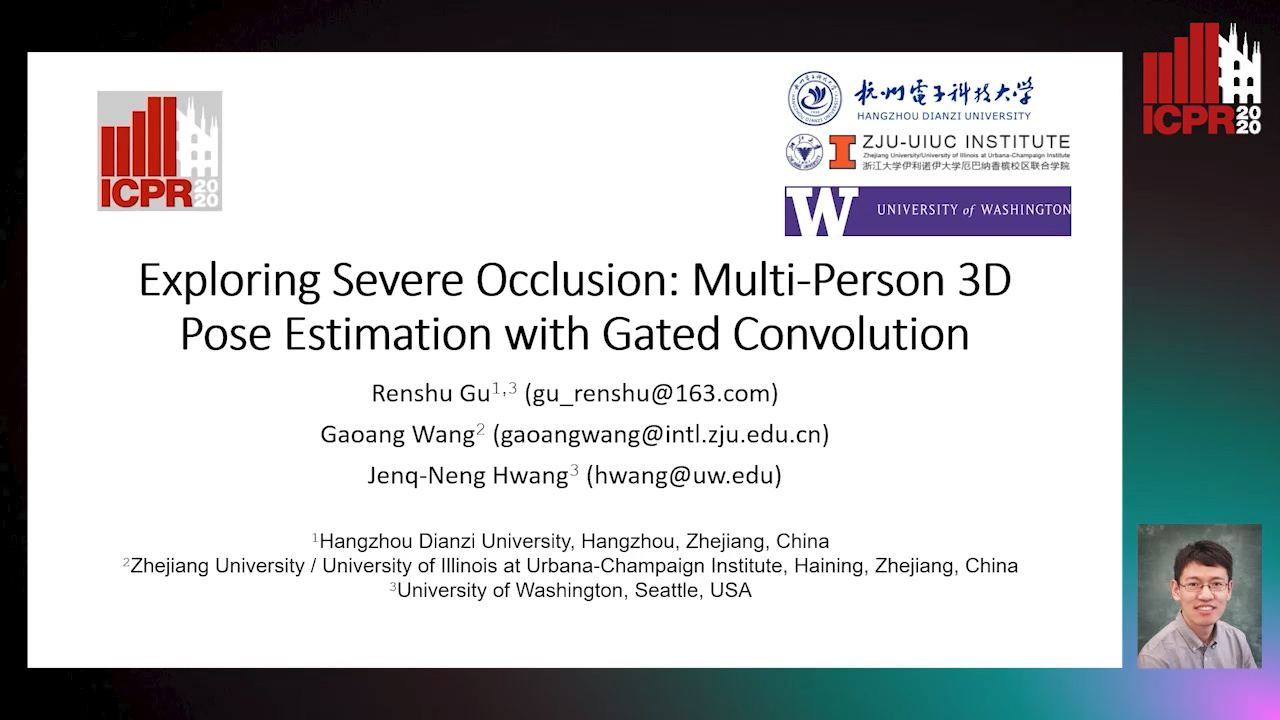
Auto-TLDR; 3D Human Pose Estimation for Multi-Human Videos with Occlusion
3D human pose estimation (HPE) is crucial in human behavior analysis, augmented reality/virtual reality (AR/VR) applications, and self-driving industry. Videos that contain multiple potentially occluded people captured from freely moving monocular cameras are very common in real-world scenarios, while 3D HPE for such scenarios is quite challenging, partially because there is a lack of such data with accurate 3D ground truth labels in existing datasets. In this paper, we propose a temporal regression network with a gated convolution module to transform 2D joints to 3D and recover the missing occluded joints in the meantime. A simple yet effective localization approach is further conducted to transform the normalized pose to the global trajectory. To verify the effectiveness of our approach, we also collect a new moving camera multi-human (MMHuman) dataset that includes multiple people with heavy occlusion captured by moving cameras. The 3D ground truth joints are provided by accurate motion capture (MoCap) system. From the experiments on static-camera based Human3.6M data and our own collected moving-camera based data, we show that our proposed method outperforms most state-of-the-art 2D-to-3D pose estimation methods, especially for the scenarios with heavy occlusions.