Stefano Mattoccia
Paper download is intended for registered attendees only, and is
subjected to the IEEE Copyright Policy. Any other use is strongly forbidden.
Papers from this author
Leveraging a Weakly Adversarial Paradigm for Joint Learning of Disparity and Confidence Estimation
Matteo Poggi, Fabio Tosi, Filippo Aleotti, Stefano Mattoccia
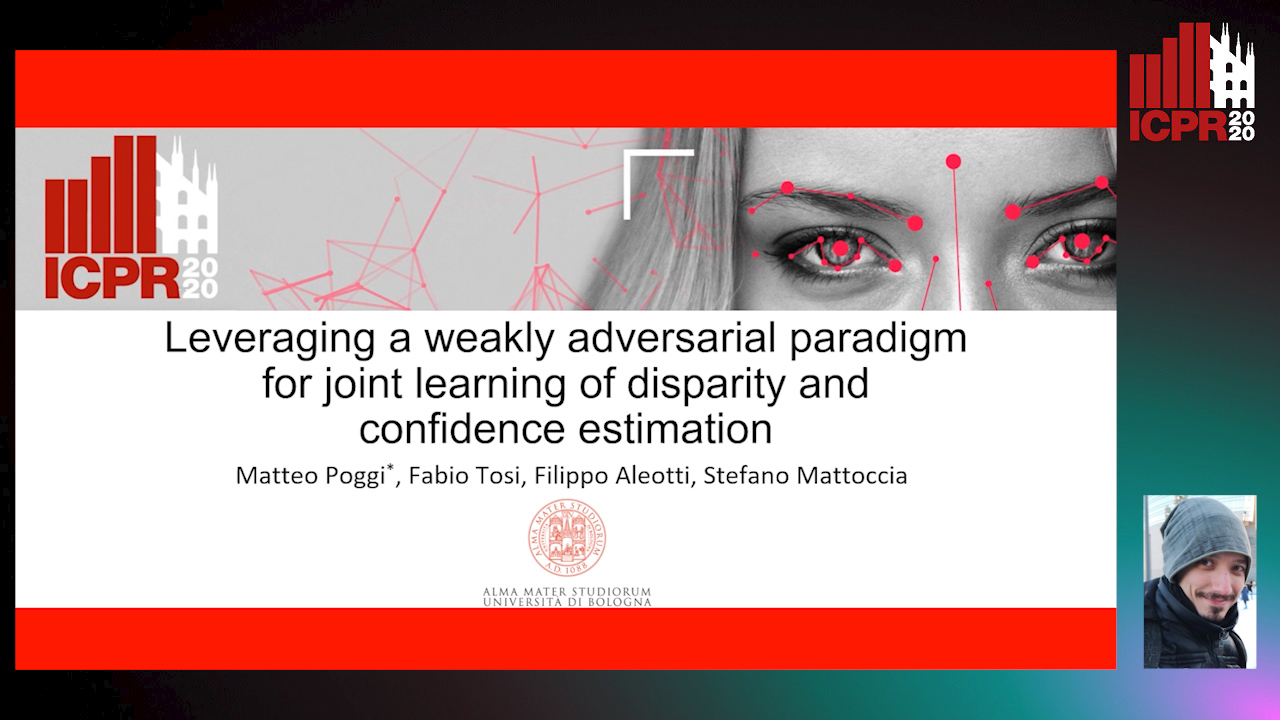
Auto-TLDR; Joint Training of Deep-Networks for Outlier Detection from Stereo Images
Abstract Slides Poster Similar
Deep architectures represent the state-of-the-art for perceiving depth from stereo images. Although these methods are highly accurate, it is crucial to effectively detect any outlier through confidence measures since a wrong perception of even small portions of the sensed scene might lead to catastrophic consequences, for instance, in autonomous driving. Purposely, state-of-the-art confidence estimation methods rely on deep-networks as well. In this paper, arguing that these tasks are two sides of the same coin, we propose a novel paradigm for their joint training. Specifically, inspired by the successful deployment of GANs in other fields, we design two deep architectures: a generator for disparity estimation and a discriminator for distinguishing correct assignments from outliers. The two networks are jointly trained in a new peculiar weakly adversarial manner pushing the former to fix the errors detected by the discriminator while keeping the correct prediction unchanged. Experimental results on standard stereo datasets prove that such joint training paradigm yields significant improvements. Moreover, an additional outcome of our proposal is the ability to detect outliers with better accuracy compared to the state-of-the-art.