Theodoros Bozinis
Paper download is intended for registered attendees only, and is
subjected to the IEEE Copyright Policy. Any other use is strongly forbidden.
Papers from this author
Improving Visual Question Answering Using Active Perception on Static Images
Theodoros Bozinis, Nikolaos Passalis, Anastasios Tefas
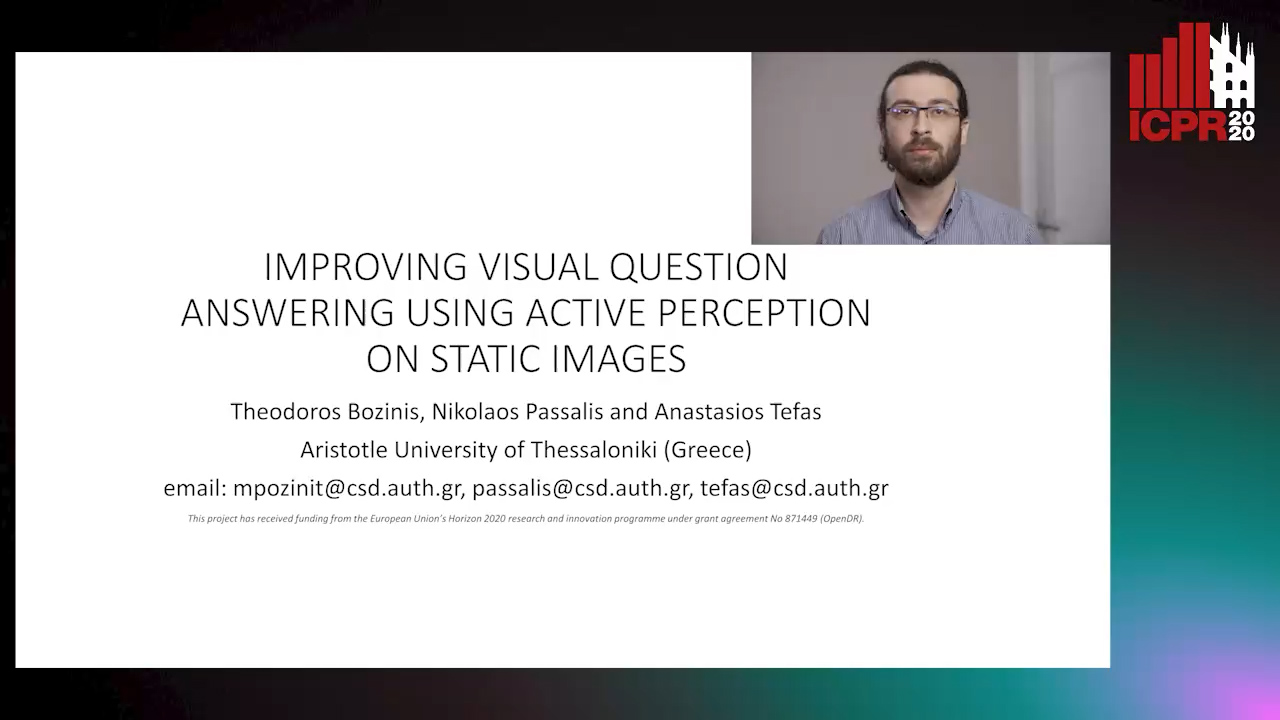
Auto-TLDR; Fine-Grained Visual Question Answering with Reinforcement Learning-based Active Perception
Abstract Slides Poster Similar
Visual Question Answering (VQA) is one of the most challenging emerging applications of deep learning. Providing powerful attention mechanisms is crucial for VQA, since the model must correctly identify the region of an image that is relevant to the question at hand. However, existing models analyze the input images at a fixed and typically small resolution, often leading to discarding valuable fine-grained details. To overcome this limitation, in this work we propose a reinforcement learning-based active perception approach that works by applying a series of transformation operations on the images (translation, zoom) in order to facilitate answering the question at hand. This allows for performing fine-grained analysis, effectively increasing the resolution at which the models process information. The proposed method is orthogonal to existing attention mechanisms and it can be combined with most existing VQA methods. The effectiveness of the proposed method is experimentally demonstrated on a challenging VQA dataset.