Davide Cavagnino
Paper download is intended for registered attendees only, and is
subjected to the IEEE Copyright Policy. Any other use is strongly forbidden.
Papers from this author
Delving in the Loss Landscape to Embed Robust Watermarks into Neural Networks
Enzo Tartaglione, Marco Grangetto, Davide Cavagnino, Marco Botta
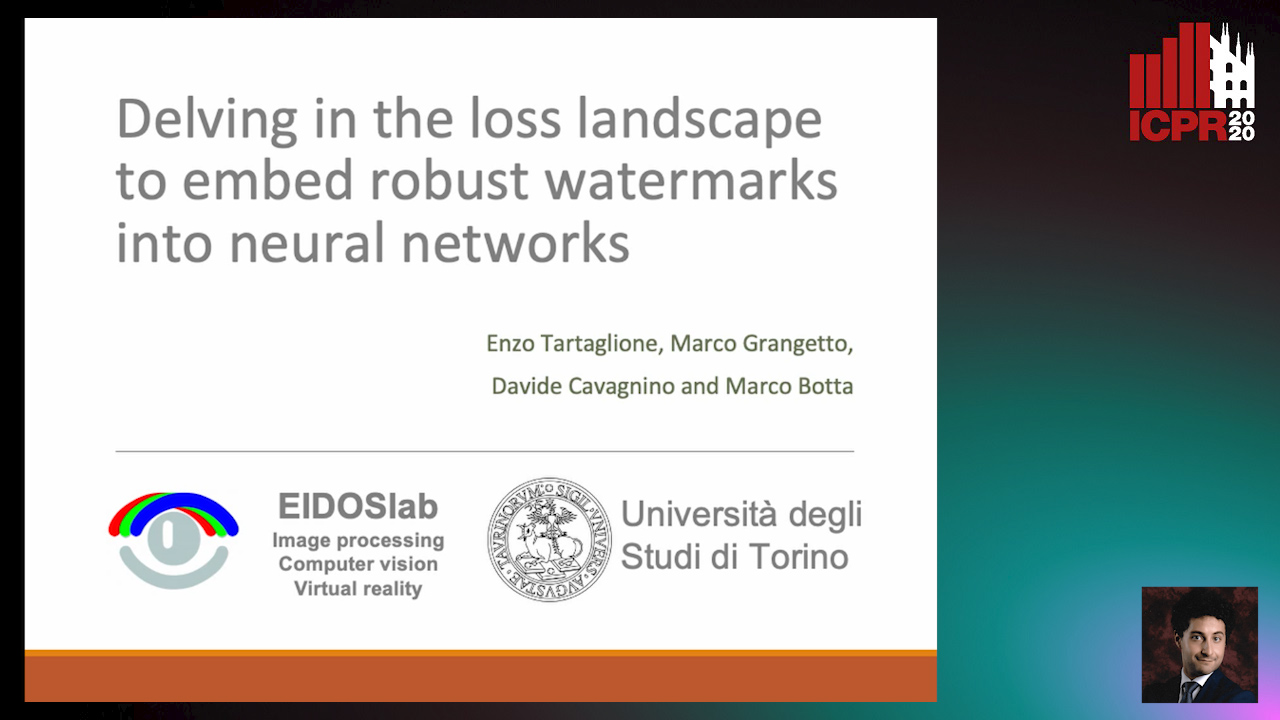
Auto-TLDR; Watermark Aware Training of Neural Networks
Abstract Slides Poster Similar
In the last decade the use of artificial neural networks (ANNs) in many fields like image processing or speech recognition has become a common practice because of their effectiveness to solve complex tasks. However, in such a rush, very little attention has been paid to security aspects. In this work we explore the possibility to embed a watermark into the ANN parameters. We exploit model redundancy and adaptation capacity to lock a subset of its parameters to carry the watermark sequence. The watermark can be extracted in a simple way to claim copyright on models but can be very easily attacked with model fine-tuning. To tackle this culprit we devise a novel watermark aware training strategy. We aim at delving into the loss landscape to find an optimal configuration of the parameters such that we are robust to fine-tuning attacks towards the watermarked parameters. Our experimental results on classical ANN models trained on well-known MNIST and CIFAR-10 datasets show that the proposed approach makes the embedded watermark robust to fine-tuning and compression attacks.