Ryosuke Furuta
Paper download is intended for registered attendees only, and is
subjected to the IEEE Copyright Policy. Any other use is strongly forbidden.
Papers from this author
Feature Point Matching in Cross-Spectral Images with Cycle Consistency Learning
Ryosuke Furuta, Naoaki Noguchi, Xueting Wang, Toshihiko Yamasaki
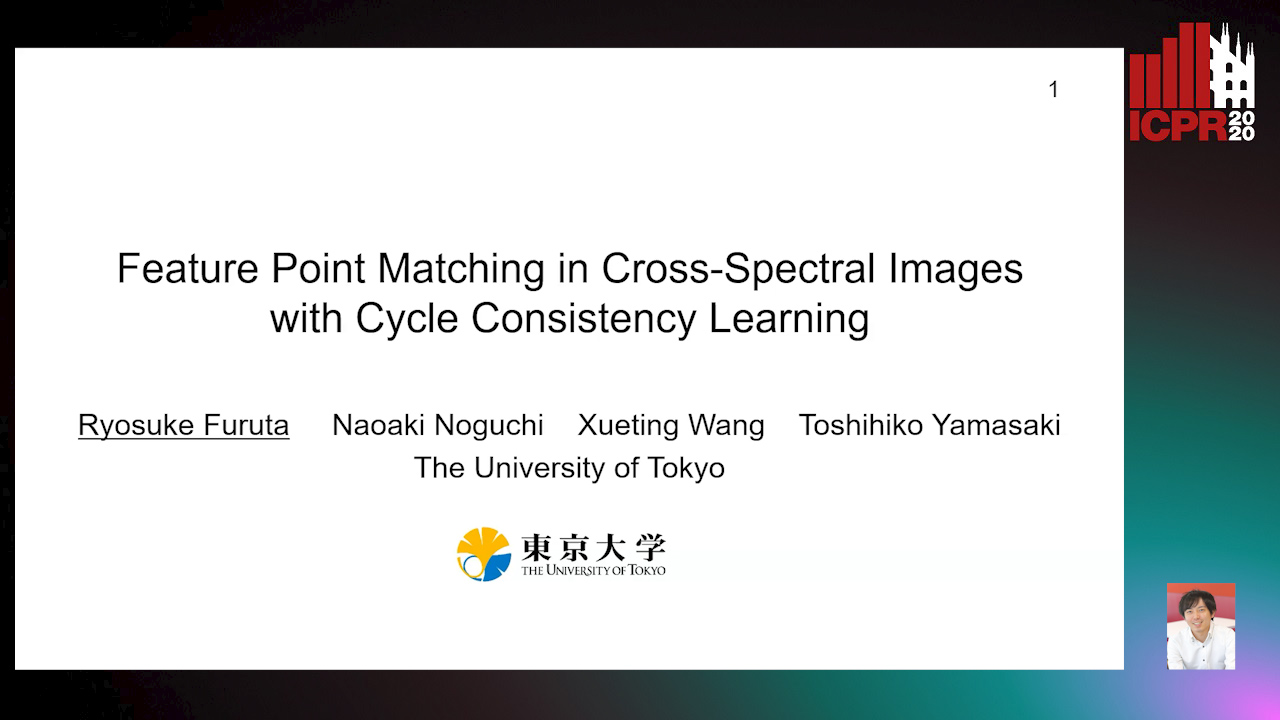
Auto-TLDR; Unsupervised Learning for General Feature Point Matching in Cross-Spectral Settings
Abstract Slides Poster Similar
Feature point matching is an important problem because its applications cover a wide range of tasks in computer vision. Deep learning-based methods for learning local features have recently shown superior performance. However, it is not easy to collect the training data in these methods, especially in cross-spectral settings such as the correspondence between RGB and near-infrared images. In this paper, we propose an unsupervised learning method for general feature point matching. Because we train a convolutional neural network as a feature extractor in order to satisfy the cycle consistency of the correspondences between an input image pair, the proposed method does not require supervision and works even in cross-spectral settings. In our experiments, we apply the proposed method to stereo matching, which is a dense feature point matching problem. The experimental results, which simulate cross-spectral settings with three different settings, i.e., RGB stereo, RGB vs gray-scale, and anaglyph (red vs cyan), show that our proposed method outperforms the compared methods, which employ handcrafted features for stereo matching, by a significant margin.