Guillaume Vaudaux-Ruth
Paper download is intended for registered attendees only, and is
subjected to the IEEE Copyright Policy. Any other use is strongly forbidden.
Papers from this author
ActionSpotter: Deep Reinforcement Learning Framework for Temporal Action Spotting in Videos
Guillaume Vaudaux-Ruth, Adrien Chan-Hon-Tong, Catherine Achard
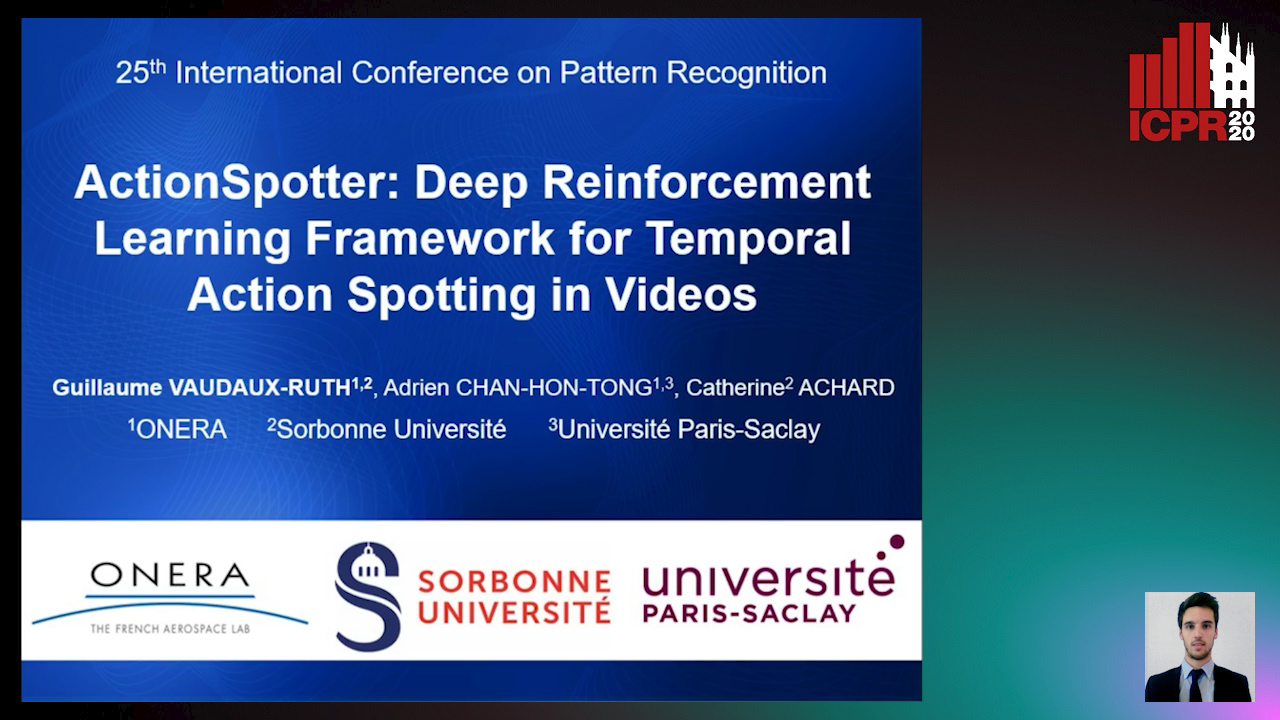
Auto-TLDR; ActionSpotter: A Reinforcement Learning Algorithm for Action Spotting in Video
Abstract Slides Poster Similar
Action spotting has recently been proposed as an alternative to action detection and key frame extraction. However, the current state-of-the-art method of action spotting requires an expensive ground truth composed of the search sequences employed by human annotators spotting actions - a critical limitation. In this article, we propose to use a reinforcement learning algorithm to perform efficient action spotting using only the temporal segments from the action detection annotations, thus opening an interesting solution for video understanding. Experiments performed on THUMOS14 and ActivityNet datasets show that the proposed method, named ActionSpotter, leads to good results and outperforms state-of-the-art detection outputs redrawn for this application. In particular, the spotting mean Average Precision on THUMOS14 is significantly improved from 59.7% to 65.6% while skipping 23% of video.