Marco Rosano
Paper download is intended for registered attendees only, and is
subjected to the IEEE Copyright Policy. Any other use is strongly forbidden.
Papers from this author
On Embodied Visual Navigation in Real Environments through Habitat
Marco Rosano, Antonino Furnari, Luigi Gulino, Giovanni Maria Farinella
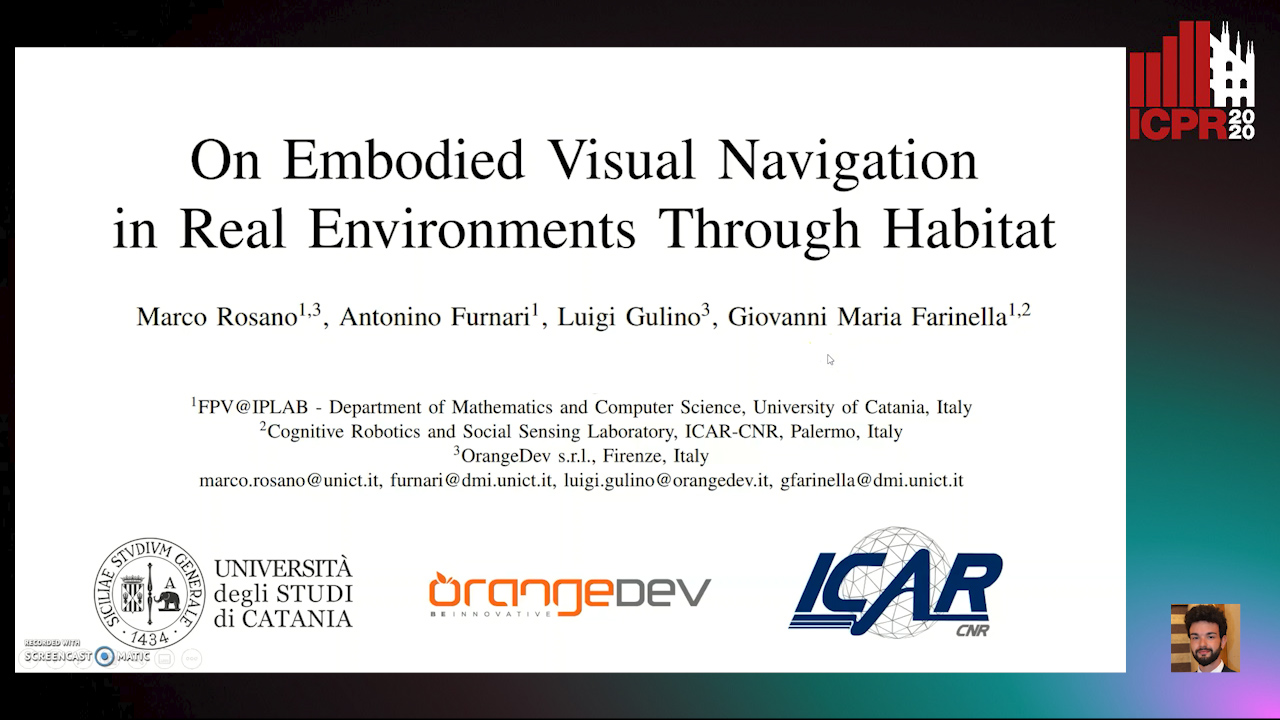
Auto-TLDR; Learning Navigation Policies on Real World Observations using Real World Images and Sensor and Actuation Noise
Abstract Slides Poster Similar
Visual navigation models based on deep learning can learn effective policies when trained on large amounts of visual observations through reinforcement learning. Unfortunately, collecting the required experience deploying a robotic platform in the real world is expensive and time-consuming. To deal with this limitation, several simulation platforms have been proposed in order to train visual navigation policies on virtual environments efficiently. Despite the advantages they offer, simulators present a limited realism in terms of appearance and physical dynamics, leading to navigation policies that do not generalize in the real world. In this paper, we propose a tool based on the Habitat simulator which exploits real world images of the environment, together with sensor and actuator noise models, to produce more realistic navigation episodes. We perform a range of experiments using virtual, real and images transformed with a simple domain adaptation approach. We also assess the impact of sensor and actuation noise on the navigation performance and investigate whether they allow to learn more robust navigation policies. We show that our tool can effectively help to train and evaluate navigation policies on real world observations without running navigation episodes in the real world.