Rupali Khatun
Paper download is intended for registered attendees only, and is
subjected to the IEEE Copyright Policy. Any other use is strongly forbidden.
Papers from this author
Uncertainty-Aware Data Augmentation for Food Recognition
Eduardo Aguilar, Bhalaji Nagarajan, Rupali Khatun, Marc Bolaños, Petia Radeva
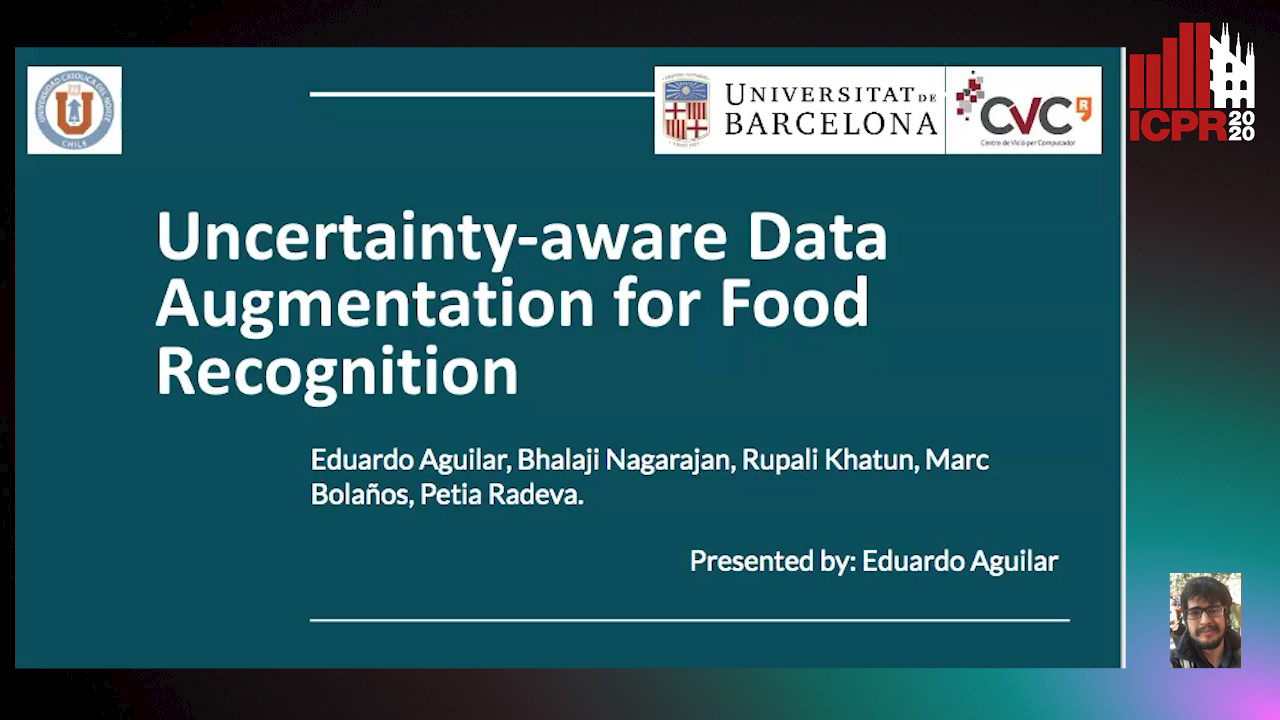
Auto-TLDR; Data Augmentation for Food Recognition Using Epistemic Uncertainty
Abstract Slides Poster Similar
Food recognition has recently attracted attention of many researchers. However, high food ambiguity, inter-class variability and intra-class similarity define a real challenge for the Deep learning and Computer Vision algorithms. In order to improve their performance, it is necessary to better understand what the model learns and, from this, to determine the type of data that should be additionally included for being the most beneficial to the training procedure. In this paper, we propose a new data augmentation strategy that estimates and uses the epistemic uncertainty to guide the model training. The method follows an active learning framework, where the new synthetic images are generated from the hard to classify real ones present in the training data based on the epistemic uncertainty. Hence, it allows the food recognition algorithm to focus on difficult images in order to learn their discriminatives features. On the other hand, avoiding data generation from images that do not contribute to the recognition makes it faster and more efficient. We show that the proposed method allows to improve food recognition and provides a better trade-off between micro- and macro-recall measures.