Chen Yang
Paper download is intended for registered attendees only, and is
subjected to the IEEE Copyright Policy. Any other use is strongly forbidden.
Papers from this author
A Transformer-Based Radical Analysis Network for Chinese Character Recognition
Chen Yang, Qing Wang, Jun Du, Jianshu Zhang, Changjie Wu, Jiaming Wang
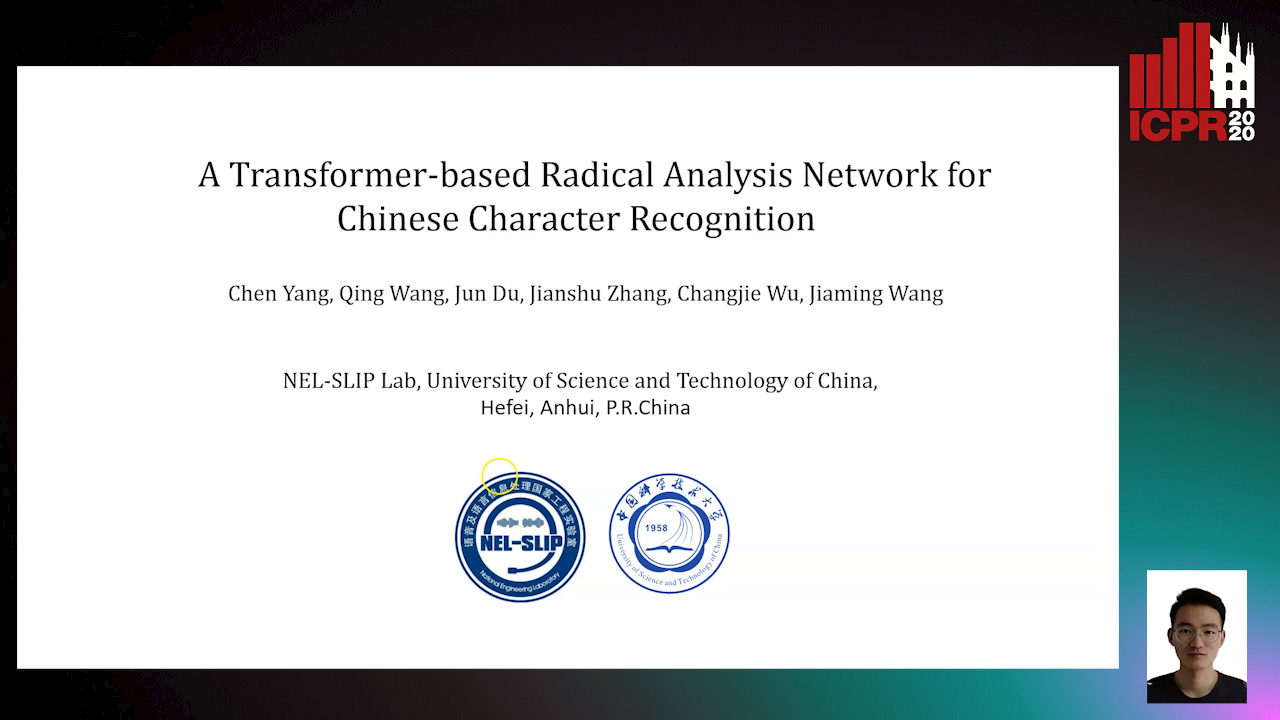
Auto-TLDR; Transformer-based Radical Analysis Network for Chinese Character Recognition
Abstract Slides Poster Similar
Recently, a novel radical analysis network (RAN) has the capability of effectively recognizing unseen Chinese character classes and largely reducing the requirement of training data by treating a Chinese character as a hierarchical composition of radicals rather than a single character class.} However, when dealing with more challenging issues, such as the recognition of complicated characters, low-frequency character categories, and characters in natural scenes, RAN still has a lot of room for improvement. In this paper, we explore options to further improve the structure generalization and robustness capability of RAN with the Transformer architecture, which has achieved start-of-the-art results for many sequence-to-sequence tasks. More specifically, we propose to replace the original attention module in RAN with the transformer decoder, which is named as a transformer-based radical analysis network (RTN). The experimental results show that the proposed approach can significantly outperform the RAN on both printed Chinese character database and natural scene Chinese character database. Meanwhile, further analysis proves that RTN can be better generalized to complex samples and low-frequency characters, and has better robustness in recognizing Chinese characters with different attributes.