Marco Fiorucci
Paper download is intended for registered attendees only, and is
subjected to the IEEE Copyright Policy. Any other use is strongly forbidden.
Papers from this author
Ancient Document Layout Analysis: Autoencoders Meet Sparse Coding
Homa Davoudi, Marco Fiorucci, Arianna Traviglia
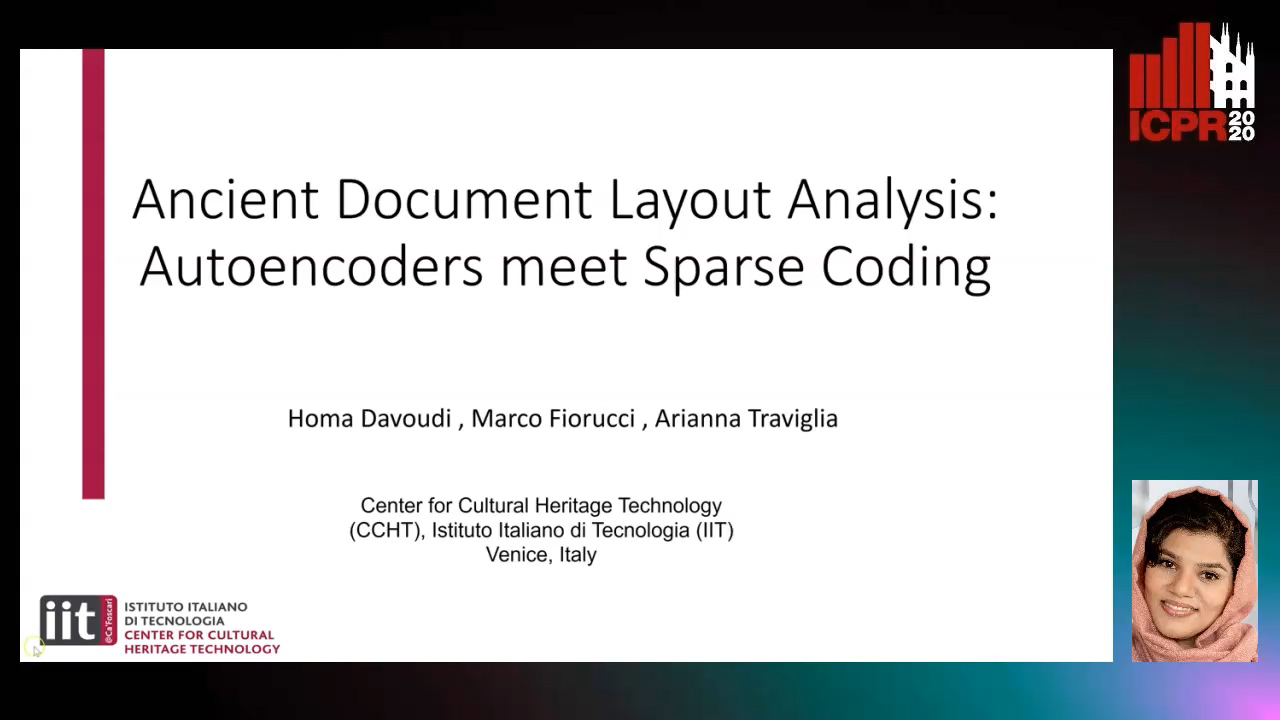
Auto-TLDR; Unsupervised Unsupervised Representation Learning for Document Layout Analysis
Abstract Slides Poster Similar
Layout analysis of historical handwritten documents is a key pre-processing step in document image analysis that, by segmenting the image into its homogeneous regions, facilitates subsequent procedures such as optical character recognition and automatic transcription. Learning-based approaches have shown promising performances in layout analysis, however, the majority of them requires tedious pixel-wise labelled training data to achieve generalisation capabilities, this limitation preventing their application due to the lack of large labelled datasets. This paper proposes a novel unsupervised representation learning method for documents’ layout analysis that reduces the need for labelled data: a sparse autoencoder is first trained in an unsupervised manner on a historical text document’s image; representation of image patches, computed by the sparse encoder, is then used to classify pixels into various region categories of the document using a feed-forward neural network. A new training method, inspired by the ISTA algorithm, is also introduced here to train the sparse encoder. Experimental results on DIVA-HisDB dataset demonstrate that the proposed method outperforms previous approaches based on unsupervised representation learning while achieving performances comparable to the state-of-the-art fully supervised methods.