Guo Danfeng
Paper download is intended for registered attendees only, and is
subjected to the IEEE Copyright Policy. Any other use is strongly forbidden.
Papers from this author
A Transformer-Based Network for Anisotropic 3D Medical Image Segmentation
Guo Danfeng, Demetri Terzopoulos
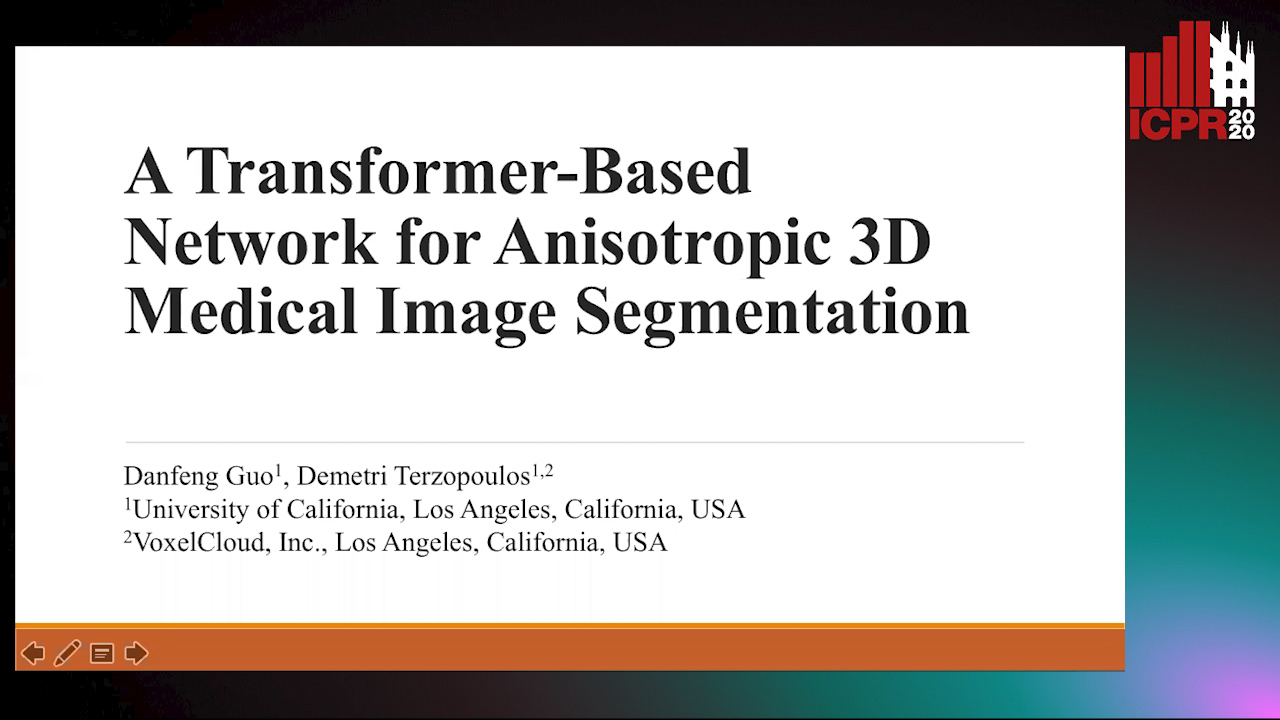
Auto-TLDR; A transformer-based model to tackle the anisotropy problem in 3D medical image analysis
Abstract Slides Poster Similar
A critical challenge of applying neural networks to 3D medical image analysis is to deal with the anisotropy problem. The inter-slice contextual information contained in medical images is important, especially when the structural information of lesions is needed. However, such information often varies with cases because of variable slice spacing. Image anisotropy downgrades model performance especially when slice spacing varies significantly among training and testing datasets. ExsiWe proposed a transformer-based model to tackle the anisotropy problem. It is adaptable to different levels of anisotropy and is computationally efficient. Experiments are conducted on 3D lung cancer segmentation task. Our model achieves an average Dice score of approximately 0.87, which generally outperforms baseline models.