Abdullah-Al-Zubaer Imran
Paper download is intended for registered attendees only, and is
subjected to the IEEE Copyright Policy. Any other use is strongly forbidden.
Papers from this author
Progressive Adversarial Semantic Segmentation
Abdullah-Al-Zubaer Imran, Demetri Terzopoulos
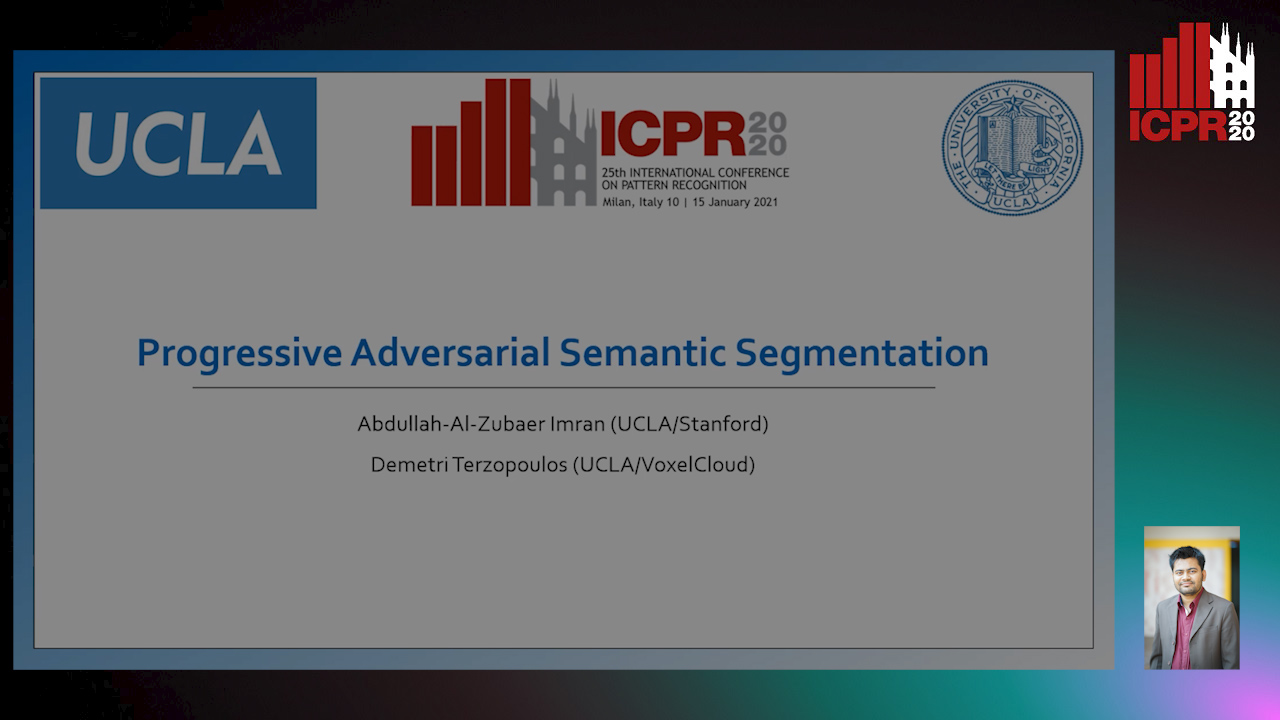
Auto-TLDR; Progressive Adversarial Semantic Segmentation for End-to-End Medical Image Segmenting
Abstract Slides Poster Similar
Medical image computing has advanced rapidly with the advent of deep learning techniques such as convolutional neural networks. Deep convolutional neural networks can perform exceedingly well given full supervision. However, the success of such fully-supervised models for various image analysis tasks (e.g., anatomy or lesion segmentation from medical images) is limited to the availability of massive amounts of labeled data. Given small sample sizes, such models are prohibitively data biased with large domain shift. To tackle this problem, we propose a novel end-to-end medical image segmentation model, namely Progressive Adversarial Semantic Segmentation (PASS), which can make improved segmentation predictions without requiring any domain-specific data during training time. Our extensive experimentation with 8 public diabetic retinopathy and chest X-ray datasets, confirms the effectiveness of PASS for accurate vascular and pulmonary segmentation, both for in-domain and cross-domain evaluations.