Zongmin Wang
Paper download is intended for registered attendees only, and is
subjected to the IEEE Copyright Policy. Any other use is strongly forbidden.
Papers from this author
CSpA-DN: Channel and Spatial Attention Dense Network for Fusing PET and MRI Images
Bicao Li, Zhoufeng Liu, Shan Gao, Jenq-Neng Hwang, Jun Sun, Zongmin Wang
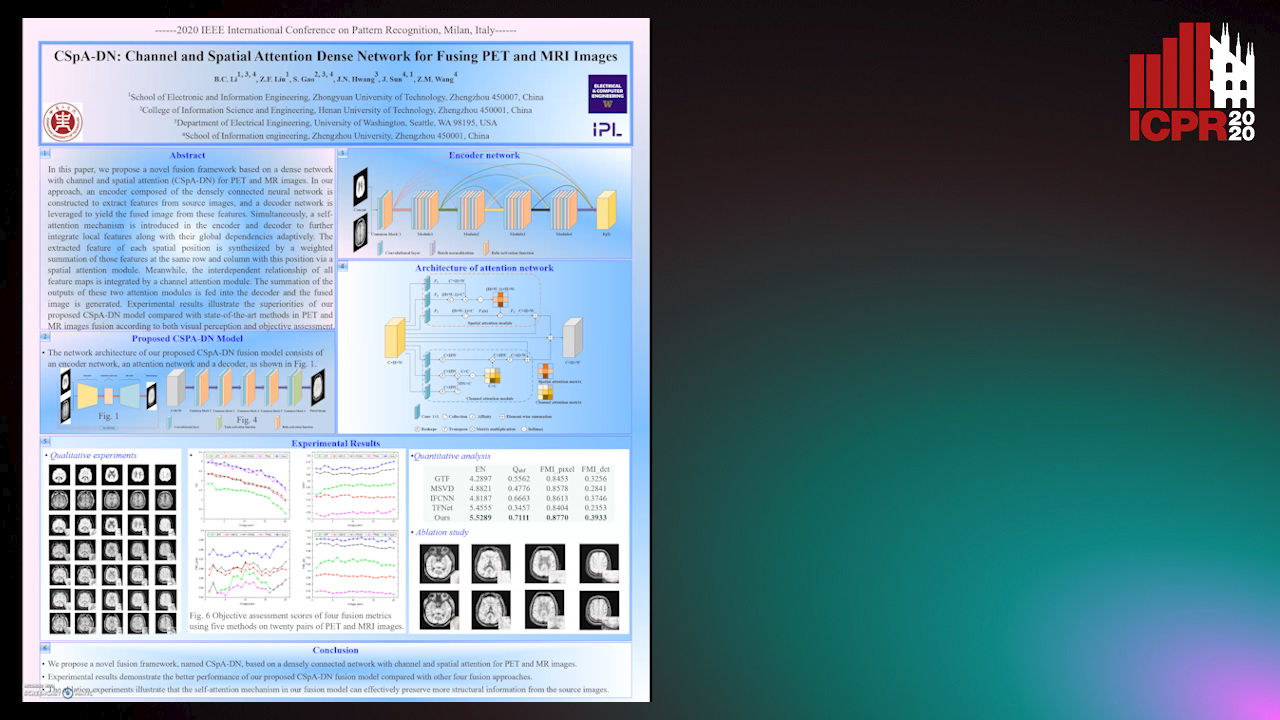
Auto-TLDR; CSpA-DN: Unsupervised Fusion of PET and MR Images with Channel and Spatial Attention
Abstract Slides Poster Similar
In this paper, we propose a novel unsupervised fusion framework based on a dense network with channel and spatial attention (CSpA-DN) for PET and MR images. In our approach, an encoder composed of the densely connected neural network is constructed to extract features from source images, and a decoder network is leveraged to yield the fused image from these features. Simultaneously, a self-attention mechanism is introduced in the encoder and decoder to further integrate local features along with their global dependencies adaptively. The extracted feature of each spatial position is synthesized by a weighted summation of those features at the same row and column with this position via a spatial attention module. Meanwhile, the interdependent relationship of all feature maps is integrated by a channel attention module. The summation of the outputs of these two attention modules is fed into the decoder and the fused image is generated. Experimental results illustrate the superiorities of our proposed CSpA-DN model compared with state-of-the-art methods in PET and MR images fusion according to both visual perception and objective assessment.