Xiang Xie
Paper download is intended for registered attendees only, and is
subjected to the IEEE Copyright Policy. Any other use is strongly forbidden.
Papers from this author
Watermelon: A Novel Feature Selection Method Based on Bayes Error Rate Estimation and a New Interpretation of Feature Relevance and Redundancy
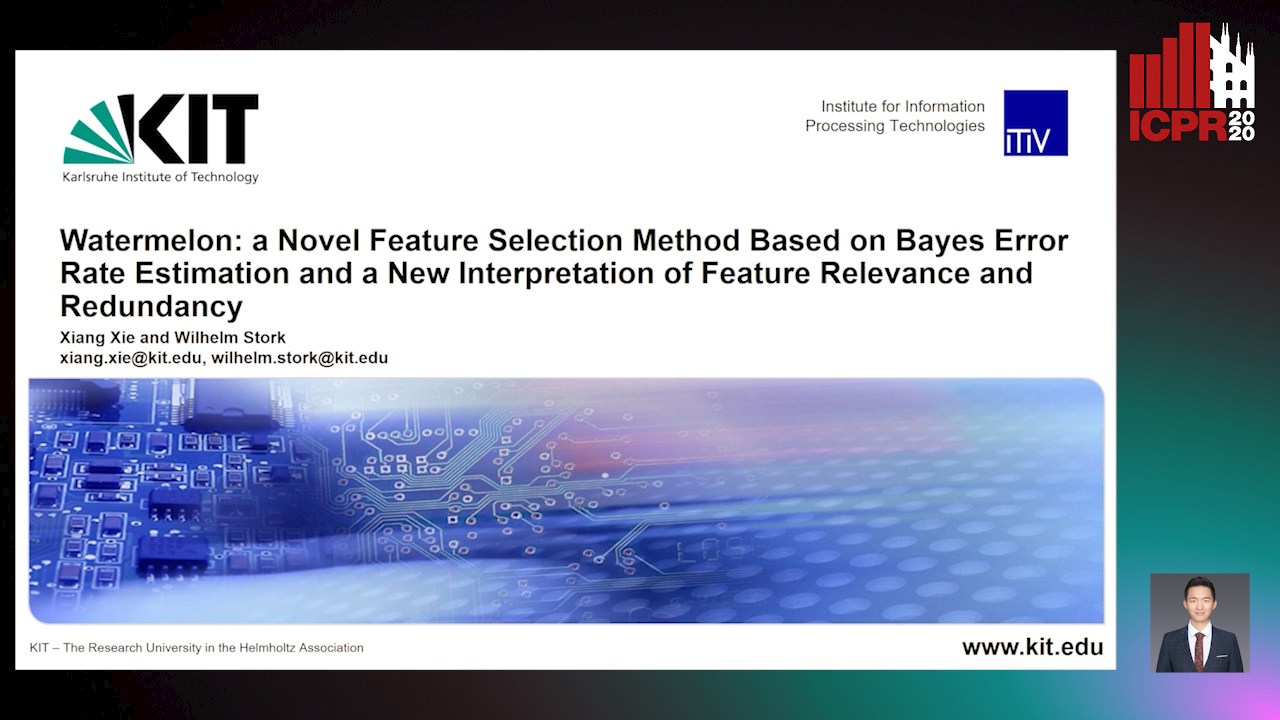
Auto-TLDR; Feature Selection Using Bayes Error Rate Estimation for Dynamic Feature Selection
Abstract Slides Poster Similar
Feature selection has become a crucial part of many classification problems in which high-dimensional datasets may contain tens of thousands of features. In this paper, we propose a novel feature selection method scoring the features through estimating the Bayes error rate based on kernel density estimation. Additionally, we update the scores of features dynamically by quantitatively interpreting the effects of feature relevance and redundancy in a new way. Distinguishing from the common heuristic applied by many feature selection methods, which prefers choosing features that are not relevant to each other, our approach penalizes only monotonically correlated features and rewards any other kind of relevance among features based on Spearman’s rank correlation coefficient and normalized mutual information. We conduct extensive experiments on seventeen diverse classification benchmarks, the results show that our approach overperforms other seventeen popular state-of-the-art feature selection methods in most cases.