Zheng Guo
Paper download is intended for registered attendees only, and is
subjected to the IEEE Copyright Policy. Any other use is strongly forbidden.
Papers from this author
A Distinct Discriminant Canonical Correlation Analysis Network Based Deep Information Quality Representation for Image Classification
Lei Gao, Zheng Guo, Ling Guan Ling Guan
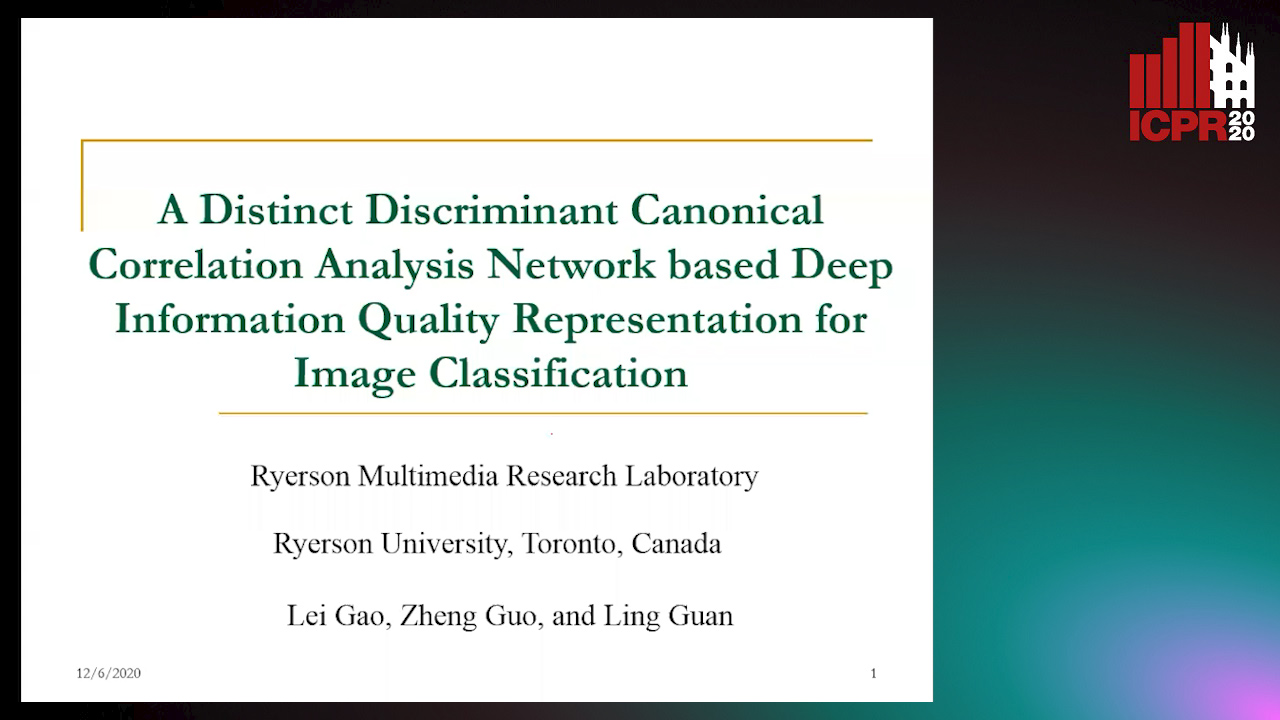
Auto-TLDR; DDCCANet: Deep Information Quality Representation for Image Classification
Abstract Slides Poster Similar
In this paper, we present a distinct discriminant canonical correlation analysis network (DDCCANet) based deep information quality representation with application to image classification. Specifically, to explore the sufficient discriminant information between different data sets, the within-class and between-class correlation matrices are employed and optimized jointly. Moreover, different from the existing canonical correlation analysis network (CCANet) and related algorithms, an information theoretic descriptor, information quality (IQ), is adopted to generate the deep-level feature representation for image classification. Benefiting from the explored discriminant information and IQ descriptor, it is potential to gain a more effective deep-level representation from multi-view data sets, leading to improved performance in classification tasks. To demonstrate the effectiveness of the proposed DDCCANet, we conduct experiments on the Olivetti Research Lab (ORL) face database, ETH80 database and CIFAR10 database. Experimental results show the superiority of the proposed solution on image classification.