Daniel Gribel
Paper download is intended for registered attendees only, and is
subjected to the IEEE Copyright Policy. Any other use is strongly forbidden.
Papers from this author
Assortative-Constrained Stochastic Block Models
Daniel Gribel, Thibaut Vidal, Michel Gendreau
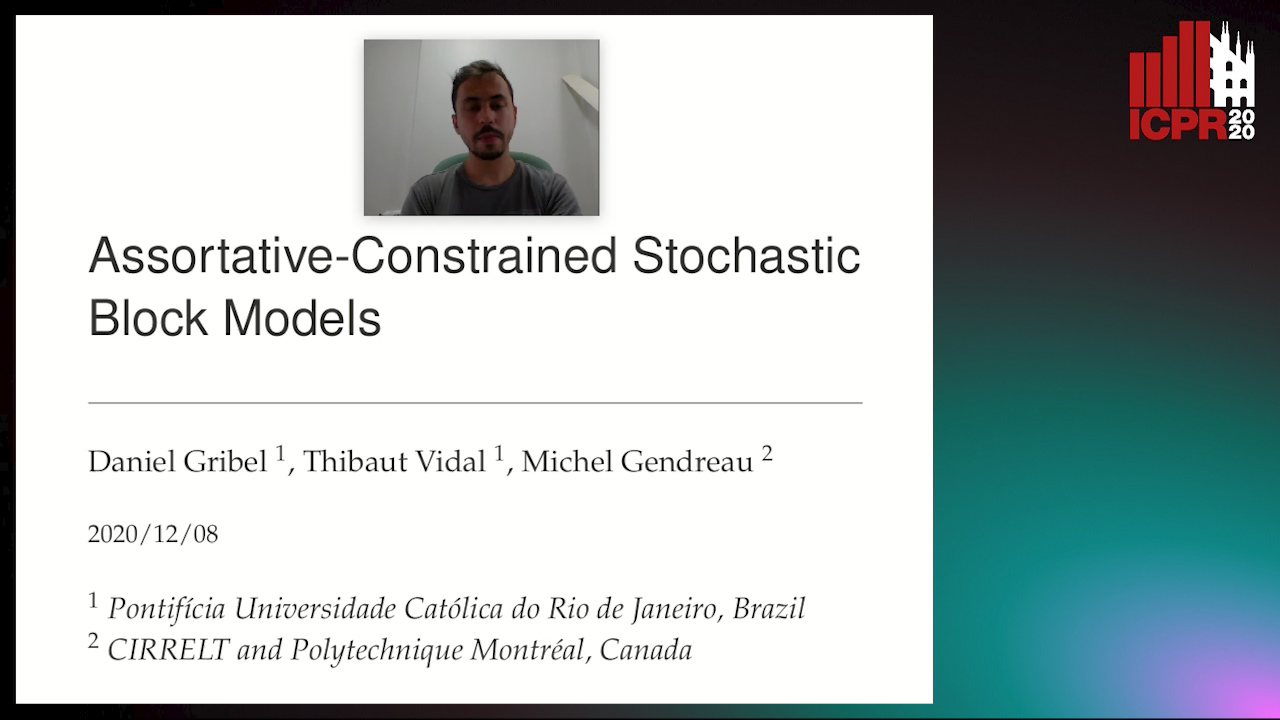
Auto-TLDR; Constrained Stochastic Block Models for Assortative Communities in Neural Networks
Abstract Slides Poster Similar
Stochastic block models (SBMs) are often used to find assortative community structures in networks, such that the probability of connections within communities is higher than in between communities. However, classic SBMs are not limited to assortative structures. In this study, we discuss the implications of this model-inherent indifference towards assortativity or disassortativity, and show that it can lead to undesirable outcomes in datasets which are known to be assortative but which contain a reduced amount of information. To circumvent these issues, we propose a constrained SBM that imposes strong assortativity constraints, along with efficient algorithmic solutions. These constraints significantly boost community-detection capabilities in regimes which are close to the detectability threshold. They also permit to identify structurally-different communities in networks representing cerebral-cortex activity regions.