Juho Kanniainen
Paper download is intended for registered attendees only, and is
subjected to the IEEE Copyright Policy. Any other use is strongly forbidden.
Papers from this author
Data Normalization for Bilinear Structures in High-Frequency Financial Time-Series
Dat Thanh Tran, Juho Kanniainen, Moncef Gabbouj, Alexandros Iosifidis
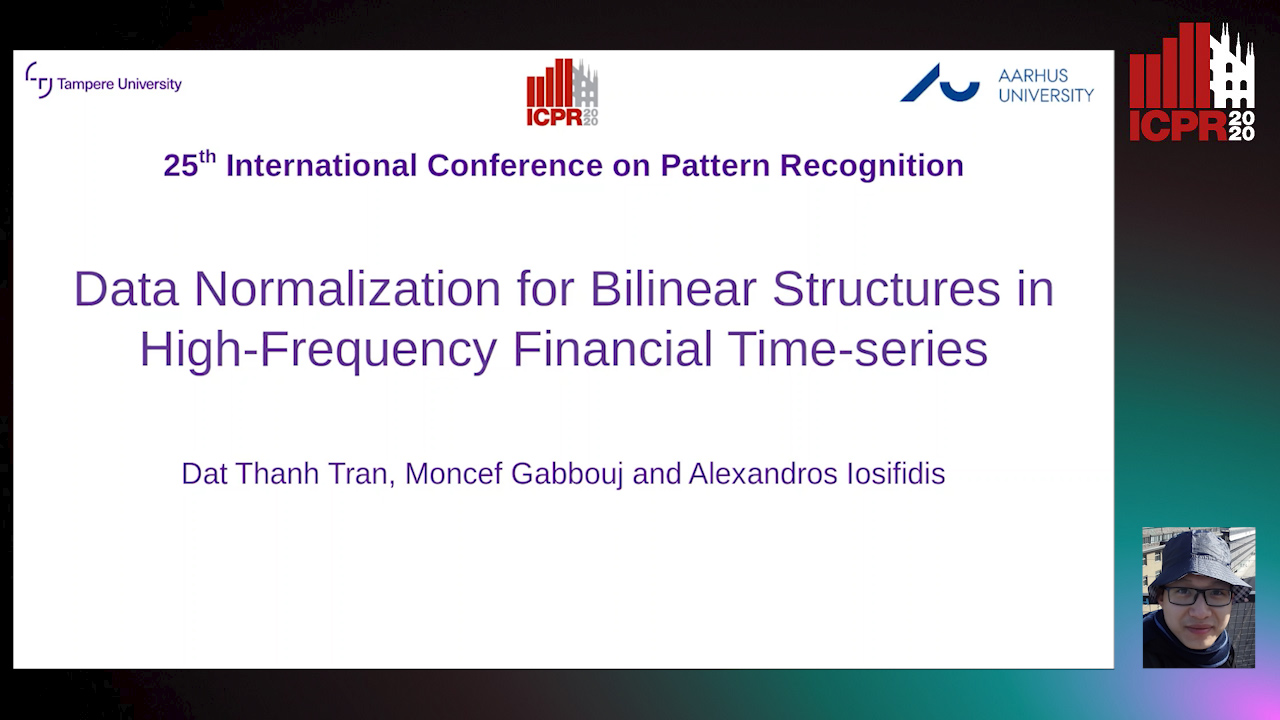
Auto-TLDR; Bilinear Normalization for Financial Time-Series Analysis and Forecasting
Abstract Slides Poster Similar
Financial time-series analysis and forecasting have been extensively studied over the past decades, yet still remain as a very challenging research topic. Since the financial market is inherently noisy and stochastic, a majority of financial time-series of interests are non-stationary, and often obtained from different modalities. This property presents great challenges and can significantly affect the performance of the subsequent analysis/forecasting steps. Recently, the Temporal Attention augmented Bilinear Layer (TABL) has shown great performances in tackling financial forecasting problems. In this paper, by taking into account the nature of bilinear projections in TABL networks, we propose Bilinear Normalization (BiN), a simple, yet efficient normalization layer to be incorporated into TABL networks to tackle potential problems posed by non-stationarity and multimodalities in the input series. Our experiments using a large scale Limit Order Book (LOB) consisting of more than 4 million order events show that BiN-TABL outperforms TABL networks using other state-of-the-arts normalization schemes by a large margin.