Jinghuang Lin
Paper download is intended for registered attendees only, and is
subjected to the IEEE Copyright Policy. Any other use is strongly forbidden.
Papers from this author
Text Recognition in Real Scenarios with a Few Labeled Samples
Jinghuang Lin, Cheng Zhanzhan, Fan Bai, Yi Niu, Shiliang Pu, Shuigeng Zhou
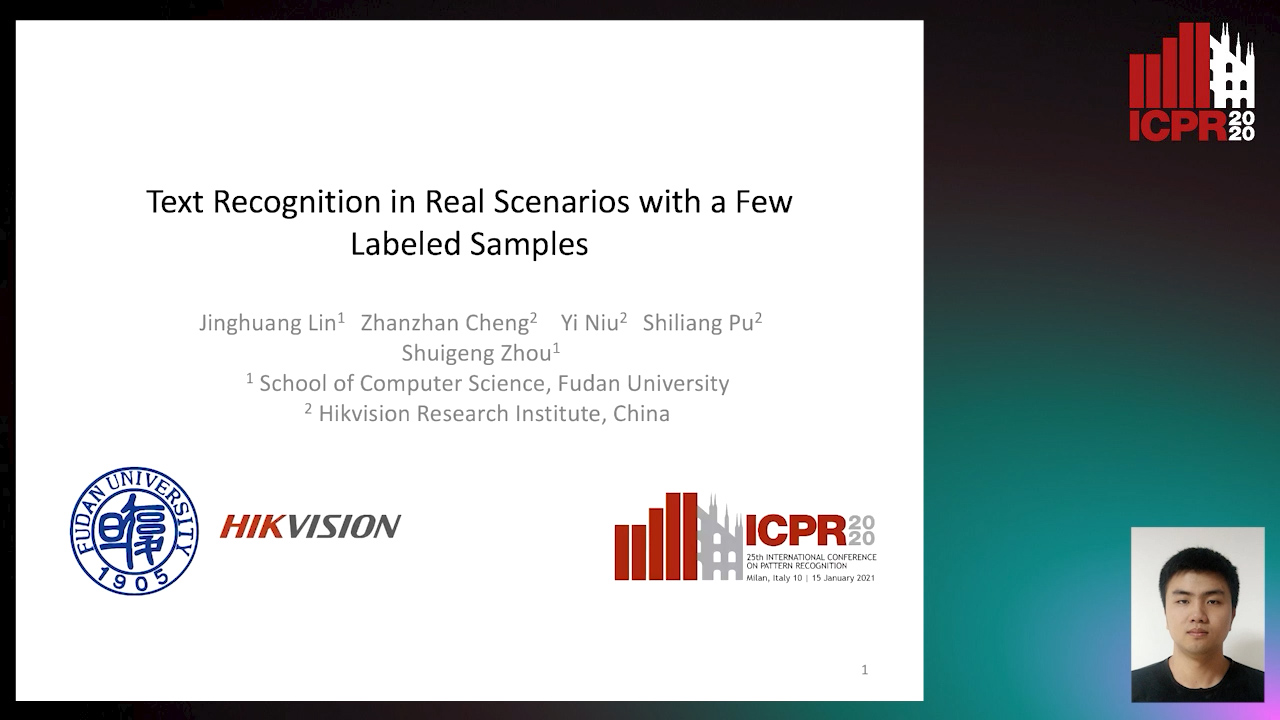
Auto-TLDR; Few-shot Adversarial Sequence Domain Adaptation for Scene Text Recognition
Abstract Slides Poster Similar
Scene text recognition (STR) is still a hot research topic in computer vision field due to its various applications. Existing works mainly focus on learning a general model with a huge number of synthetic text images to recognize unconstrained scene texts, and have achieved substantial progress. However, these methods are not quite applicable in many real-world scenarios where 1) high recognition accuracy is required, while 2) labeled samples are lacked. To tackle this challenging problem, this paper proposes a few-shot adversarial sequence domain adaptation (FASDA) approach to build sequence adaptation between the synthetic source domain (with many synthetic labeled samples) and a specific target domain (with only some or a few real labeled samples). This is done by simultaneously learning each character’s feature representation with an attention mech- anism and establishing the corresponding character-level latent subspace with adversarial learning. Our approach can maximize the character-level confusion between the source domain and the target domain, thus achieves the sequence-level adaptation with even a small number of labeled samples in the target domain. Extensive experiments on various datasets show that our method significantly outperforms the finetuning scheme, and obtains comparable performance to the state-of-the-art STR methods.