Junsong Yuan
Paper download is intended for registered attendees only, and is
subjected to the IEEE Copyright Policy. Any other use is strongly forbidden.
Papers from this author
Temporal Pulses Driven Spiking Neural Network for Time and Power Efficient Object Recognition in Autonomous Driving
Wei Wang, Shibo Zhou, Jingxi Li, Xiaohua Li, Junsong Yuan, Zhanpeng Jin
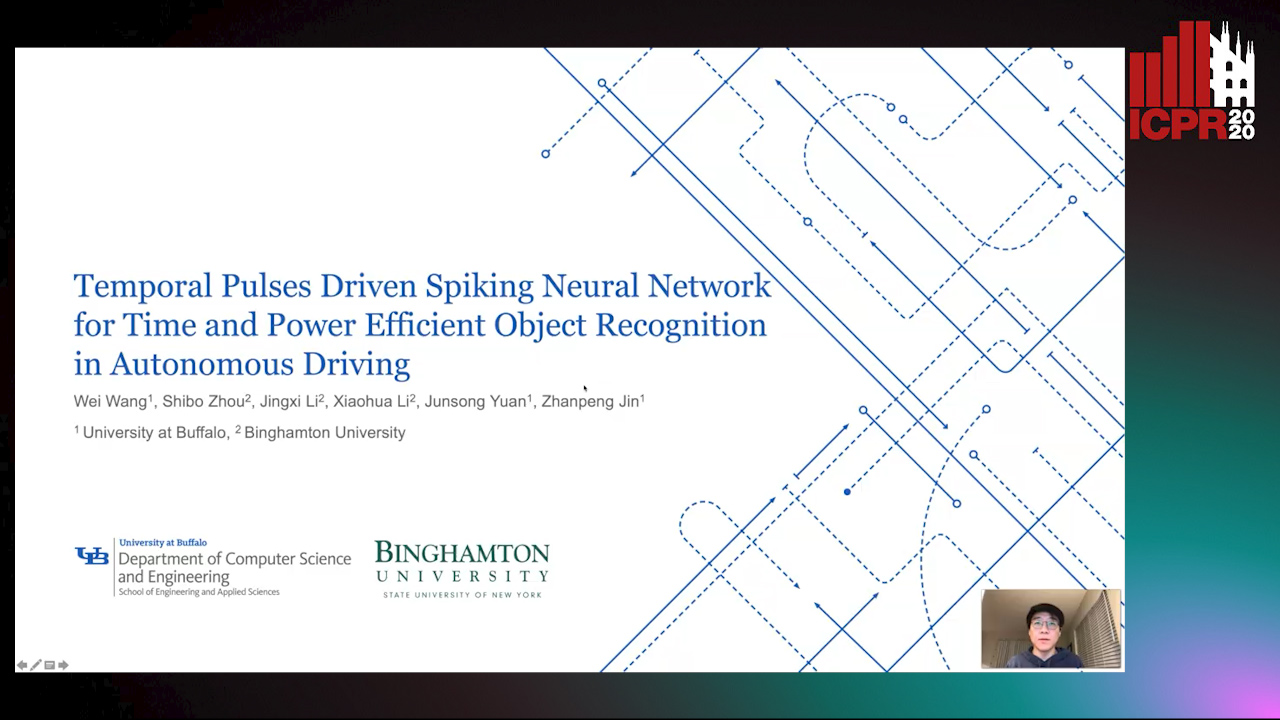
Auto-TLDR; Spiking Neural Network for Real-Time Object Recognition on Temporal LiDAR Pulses
Abstract Slides Poster Similar
Accurate real-time object recognition from sensory data has long been a crucial and challenging task for autonomous driving. Even though deep neural networks (DNNs) have been widely applied in this area, their considerable processing latency, power consumption as well as computational complexity have been challenging issues for real-time autonomous driving applications. In this paper, we propose an approach to address the real-time object recognition problem utilizing spiking neural networks (SNNs). The proposed SNN model works directly with raw temporal LiDAR pulses without the pulse-to-point cloud preprocessing procedure, which can significantly reduce delay and power consumption. Being evaluated on various datasets derived from LiDAR and dynamic vision sensor (DVS), including Sim LiDAR, KITTI, and DVS-barrel, our proposed model has shown remarkable time and power efficiency, while achieving comparable recognition performance as the state-of-the-art methods. This paper highlights the SNN's great potentials in autonomous driving and related applications. To the best of our knowledge, this is the first attempt to use SNN to directly perform time and energy efficient object recognition on temporal LiDAR pulses in the setting of autonomous driving.