Algorithm Recommendation for Data Streams
Jáder Martins Camboim De Sá,
Andre Luis Debiaso Rossi,
Gustavo Enrique De Almeida Prado Alves Batista,
Luís Paulo Faina Garcia
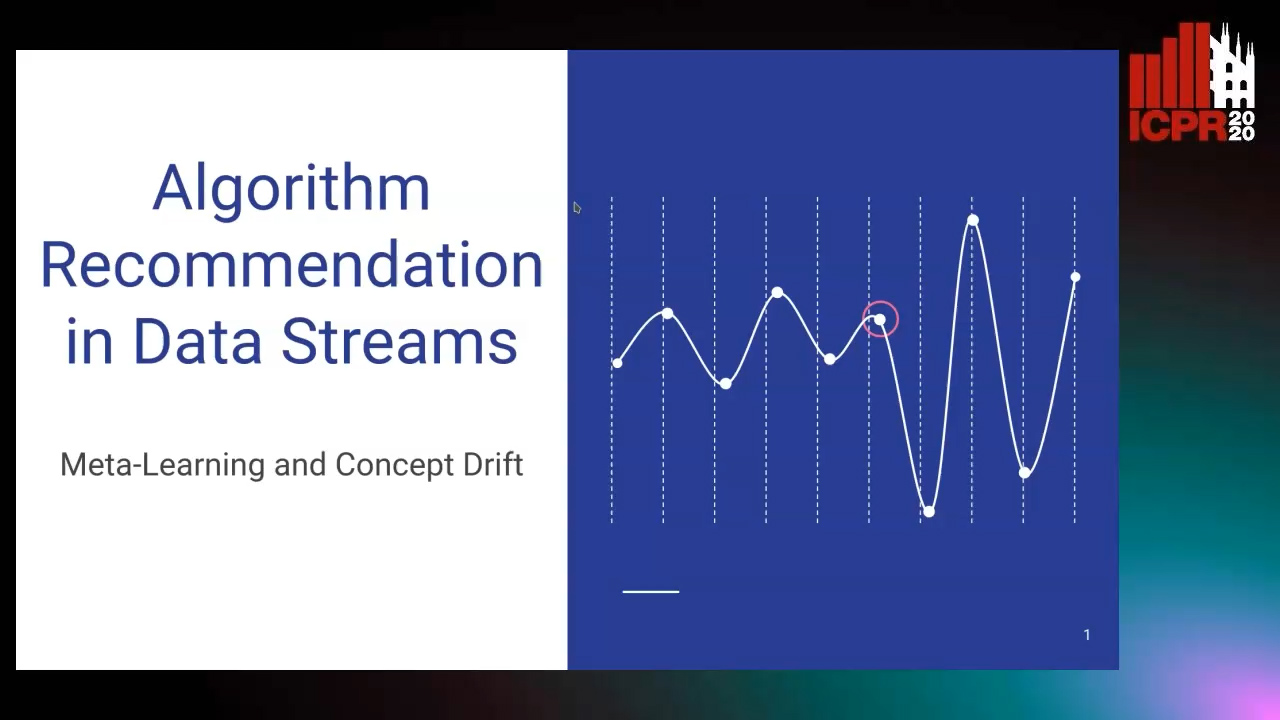
Auto-TLDR; Meta-Learning for Algorithm Selection in Time-Changing Data Streams
Similar papers
Learning Parameter Distributions to Detect Concept Drift in Data Streams
Johannes Haug, Gjergji Kasneci
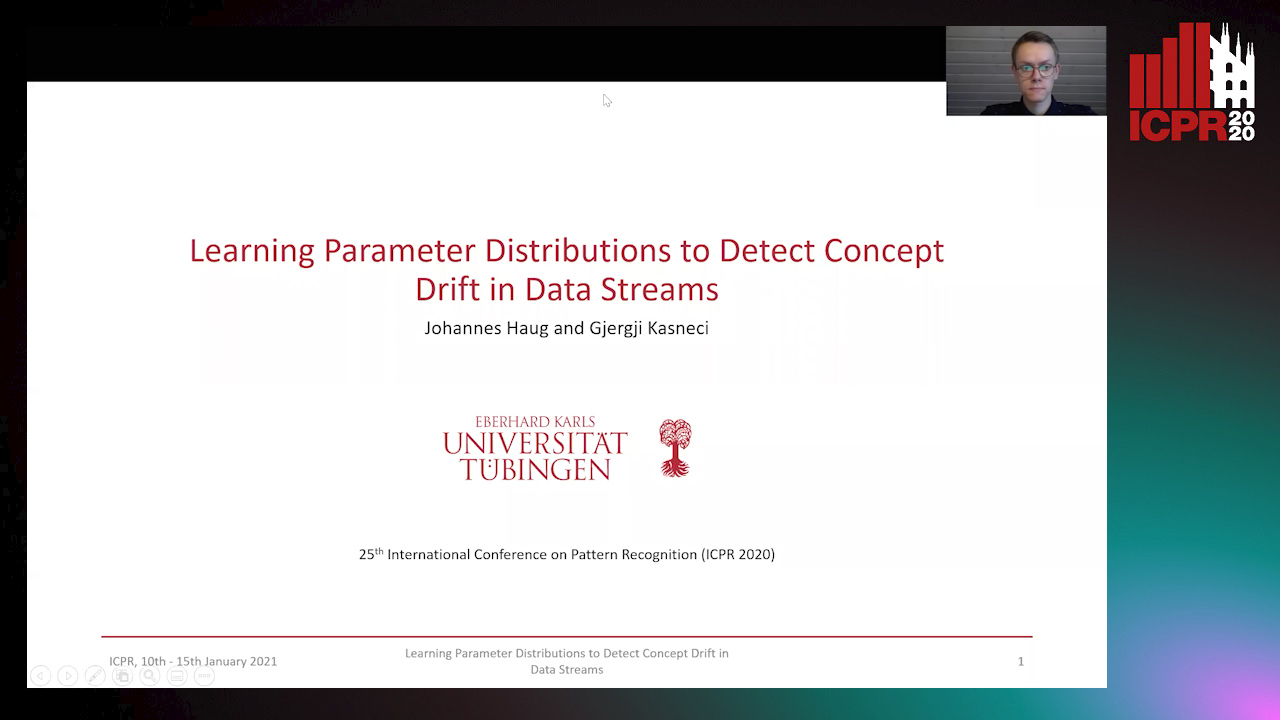
Auto-TLDR; A novel framework for the detection of concept drift in streaming environments
Abstract Slides Poster Similar
Categorizing the Feature Space for Two-Class Imbalance Learning
Rosa Sicilia, Ermanno Cordelli, Paolo Soda
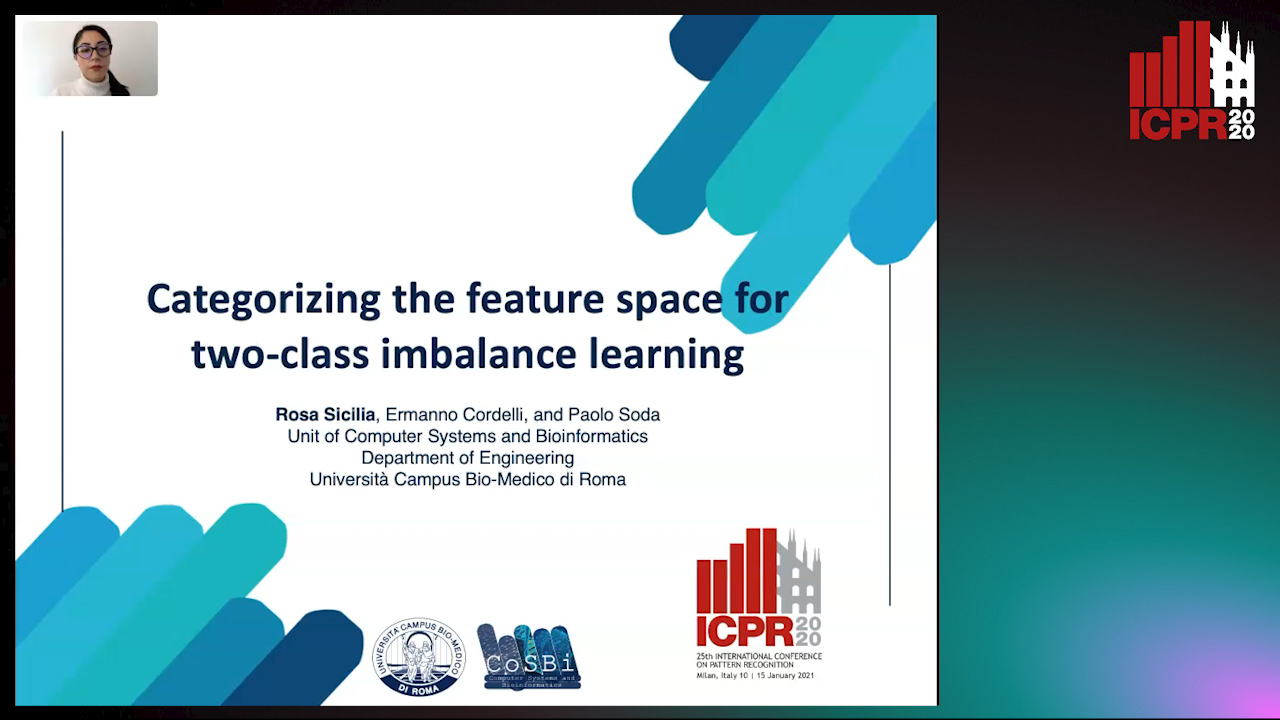
Auto-TLDR; Efficient Ensemble of Classifiers for Minority Class Inference
Abstract Slides Poster Similar
Drift Anticipation with Forgetting to Improve Evolving Fuzzy System
Clément Leroy, Eric Anquetil, Nathalie Girard
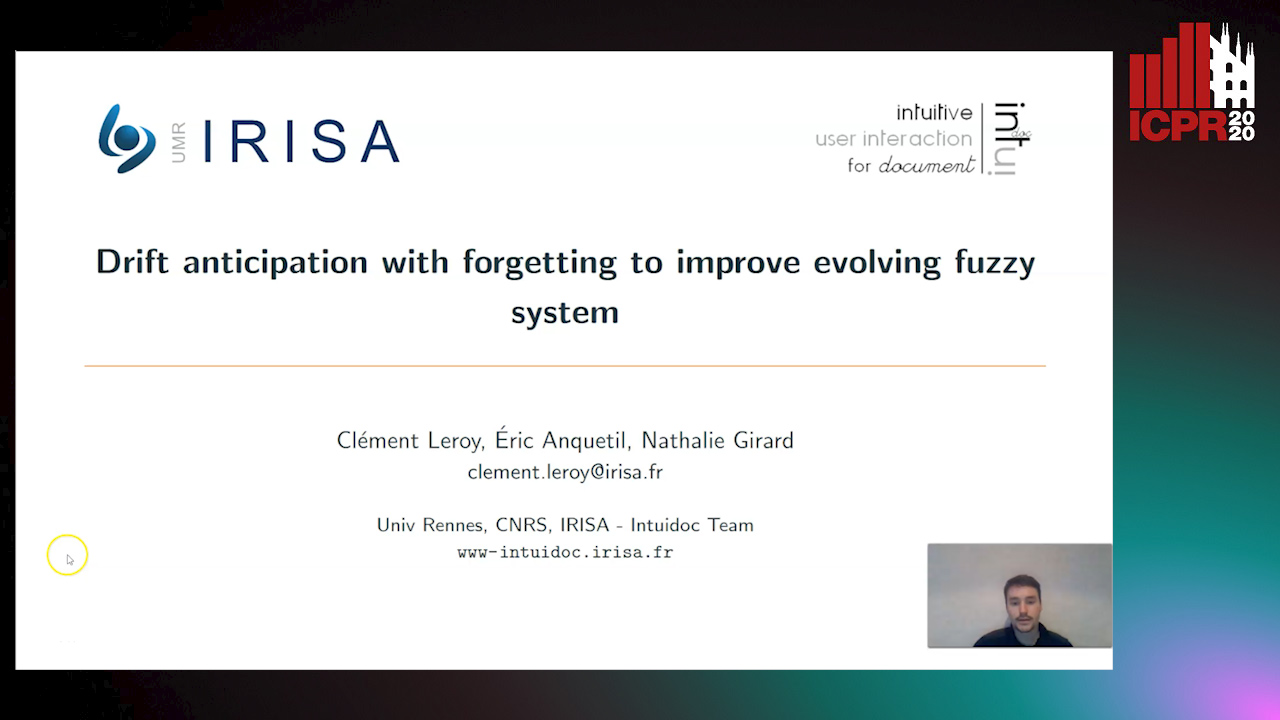
Auto-TLDR; A coherent method to integrate forgetting in Evolving Fuzzy System
Abstract Slides Poster Similar
The eXPose Approach to Crosslier Detection
Antonio Barata, Frank Takes, Hendrik Van Den Herik, Cor Veenman
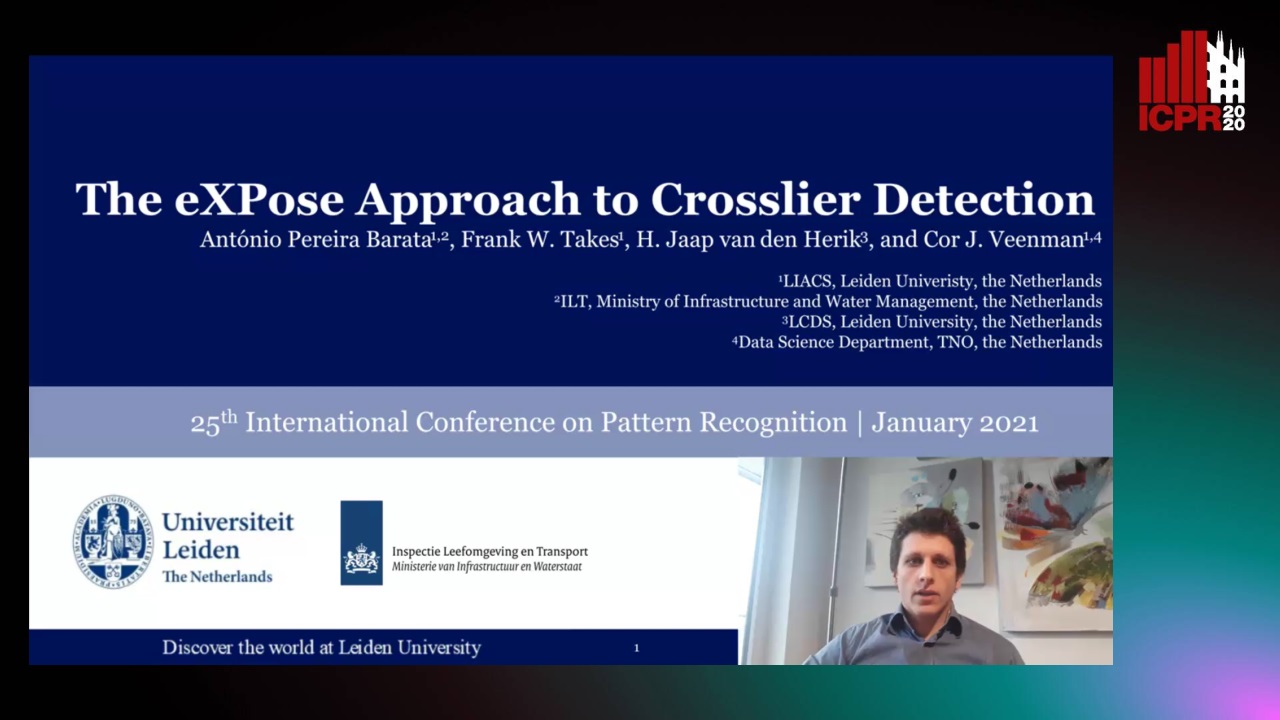
Auto-TLDR; EXPose: Crosslier Detection Based on Supervised Category Modeling
Abstract Slides Poster Similar
Personalized Models in Human Activity Recognition Using Deep Learning
Hamza Amrani, Daniela Micucci, Paolo Napoletano
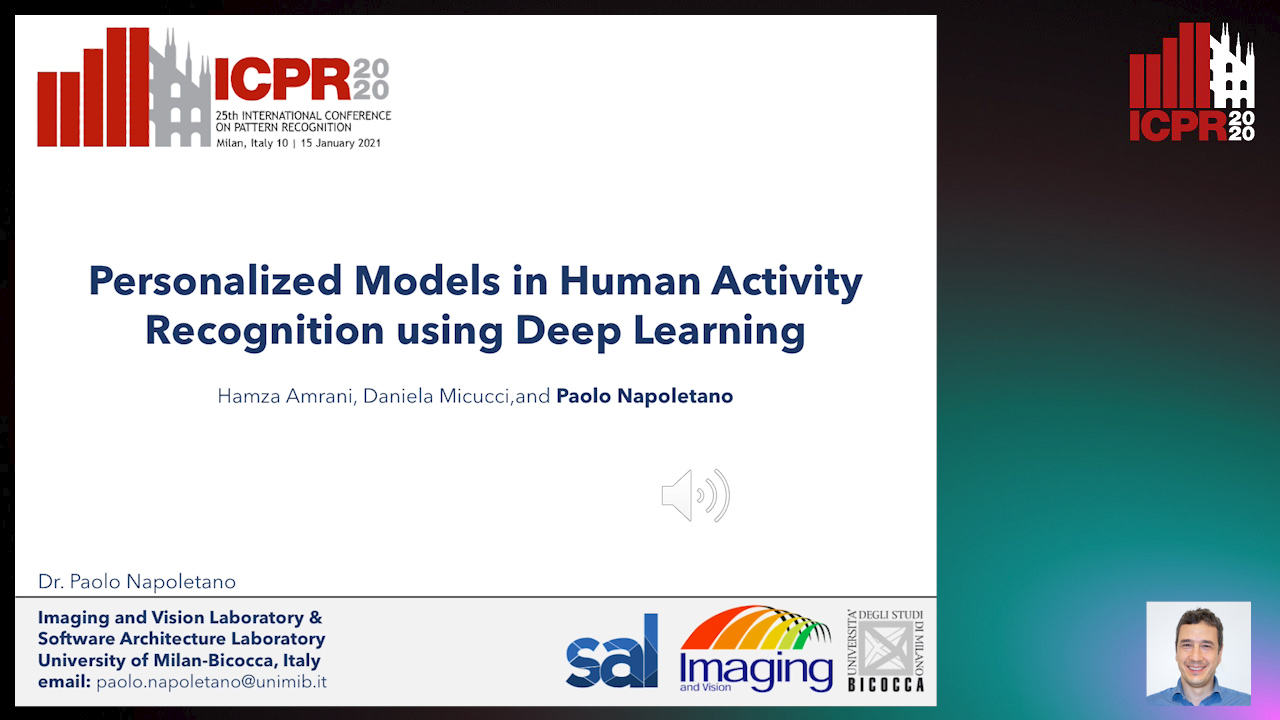
Auto-TLDR; Incremental Learning for Personalized Human Activity Recognition
Abstract Slides Poster Similar
Decision Snippet Features
Pascal Welke, Fouad Alkhoury, Christian Bauckhage, Stefan Wrobel
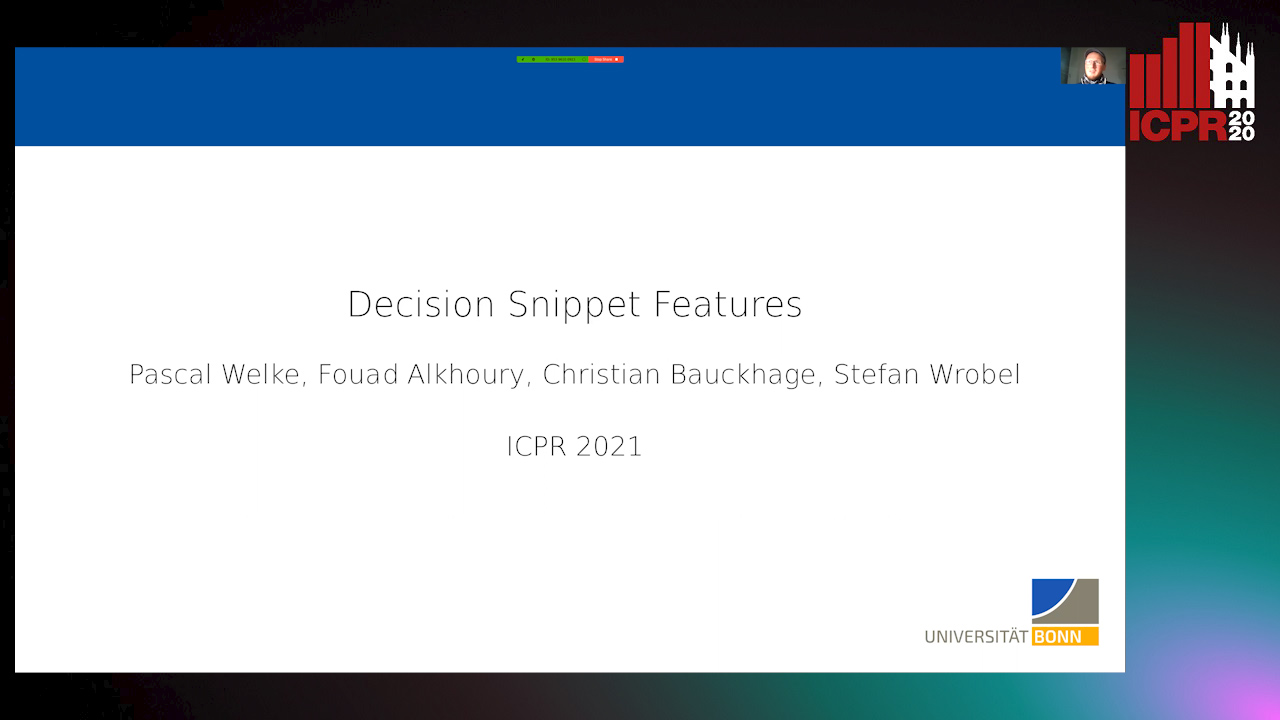
Auto-TLDR; Decision Snippet Features for Interpretability
Abstract Slides Poster Similar
Automatic Classification of Human Granulosa Cells in Assisted Reproductive Technology Using Vibrational Spectroscopy Imaging
Marina Paolanti, Emanuele Frontoni, Giorgia Gioacchini, Giorgini Elisabetta, Notarstefano Valentina, Zacà Carlotta, Carnevali Oliana, Andrea Borini, Marco Mameli
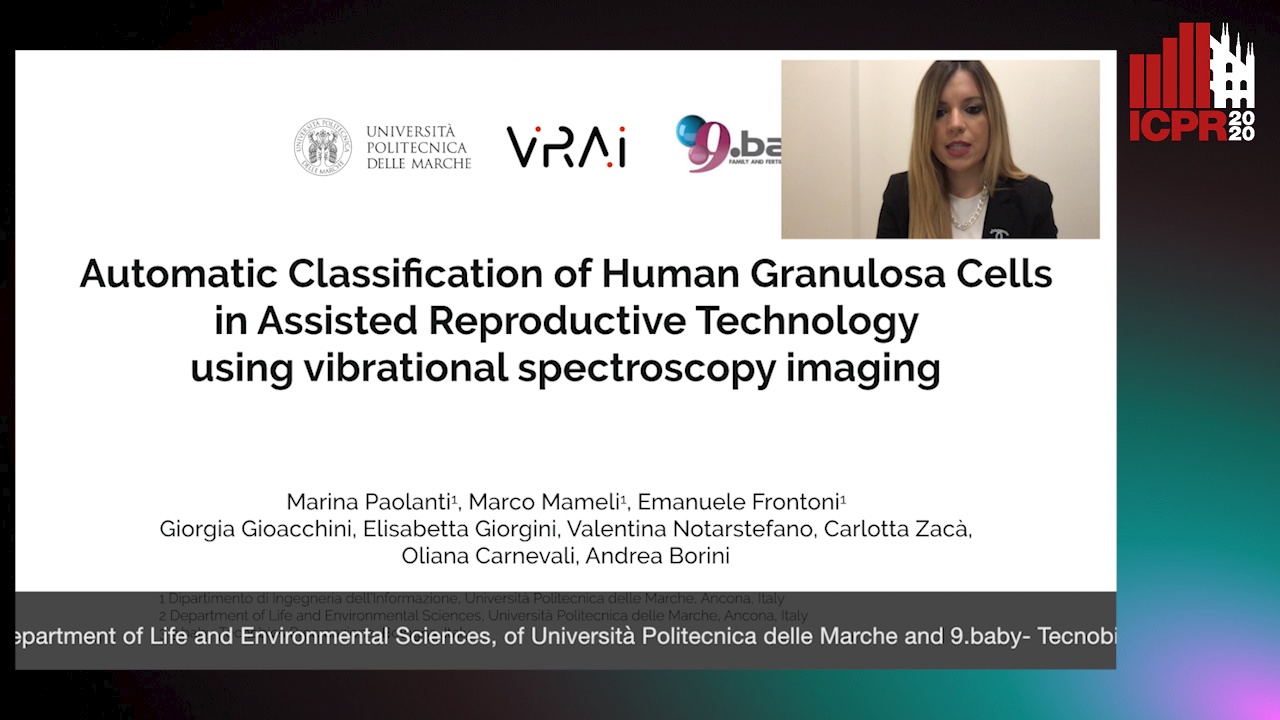
Auto-TLDR; Predicting Oocyte Quality in Assisted Reproductive Technology Using Machine Learning Techniques
Abstract Slides Poster Similar
Boundary Bagging to Address Training Data Issues in Ensemble Classification
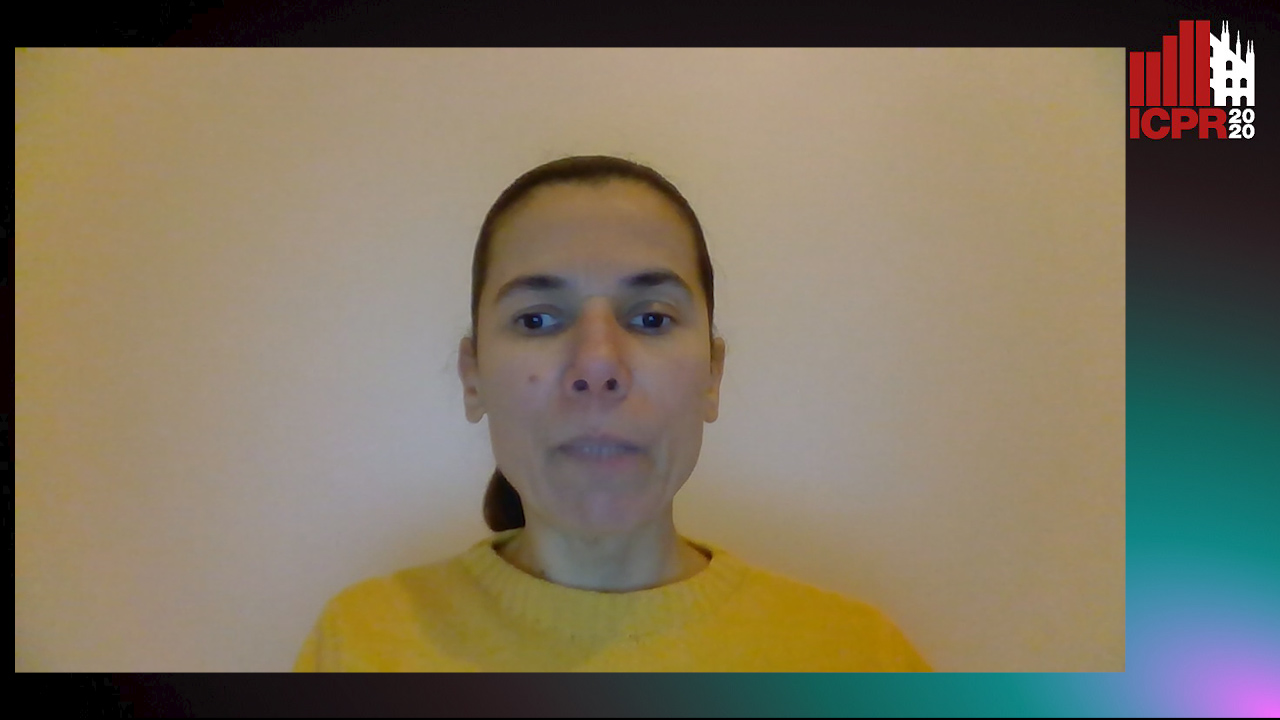
Auto-TLDR; Bagging Ensemble Learning for Multi-Class Imbalanced Classification
Dual-Memory Model for Incremental Learning: The Handwriting Recognition Use Case
Mélanie Piot, Bérangère Bourdoulous, Aurelia Deshayes, Lionel Prevost

Auto-TLDR; A dual memory model for handwriting recognition
Leveraging Sequential Pattern Information for Active Learning from Sequential Data
Raul Fidalgo-Merino, Lorenzo Gabrielli, Enrico Checchi
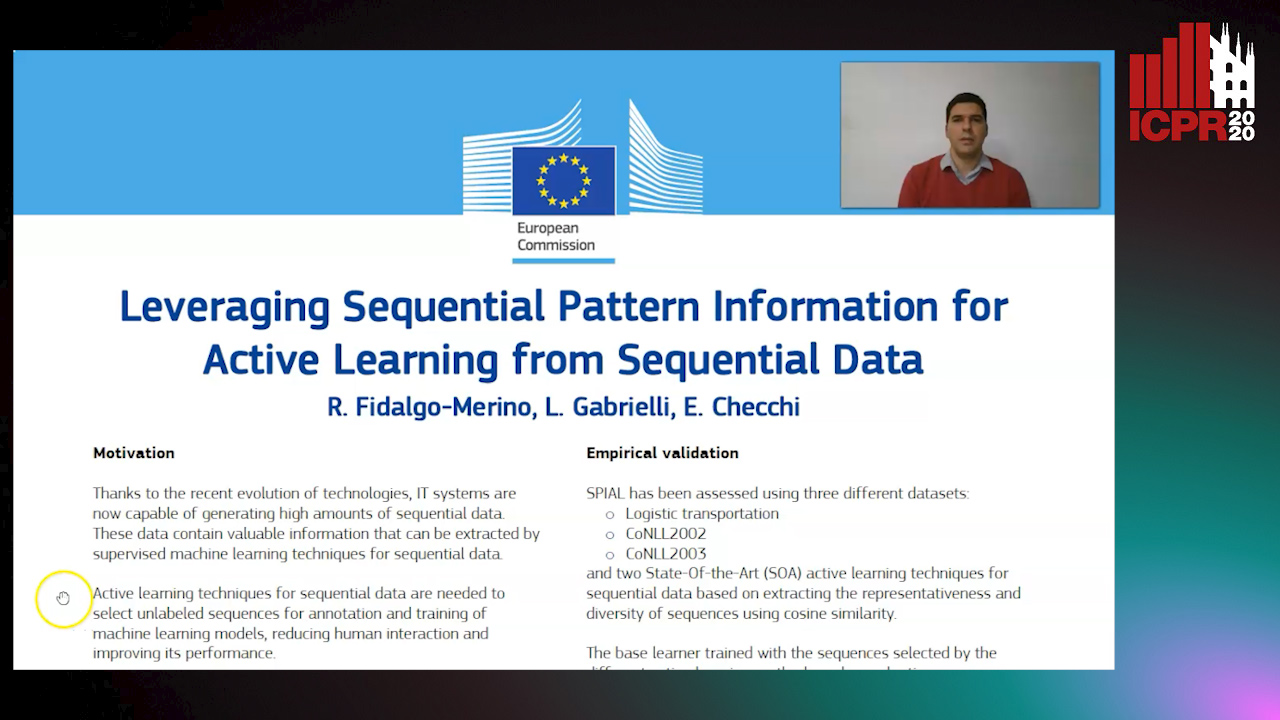
Auto-TLDR; Sequential Pattern Information for Active Learning
Abstract Slides Poster Similar
A Novel Adaptive Minority Oversampling Technique for Improved Classification in Data Imbalanced Scenarios
Ayush Tripathi, Rupayan Chakraborty, Sunil Kumar Kopparapu
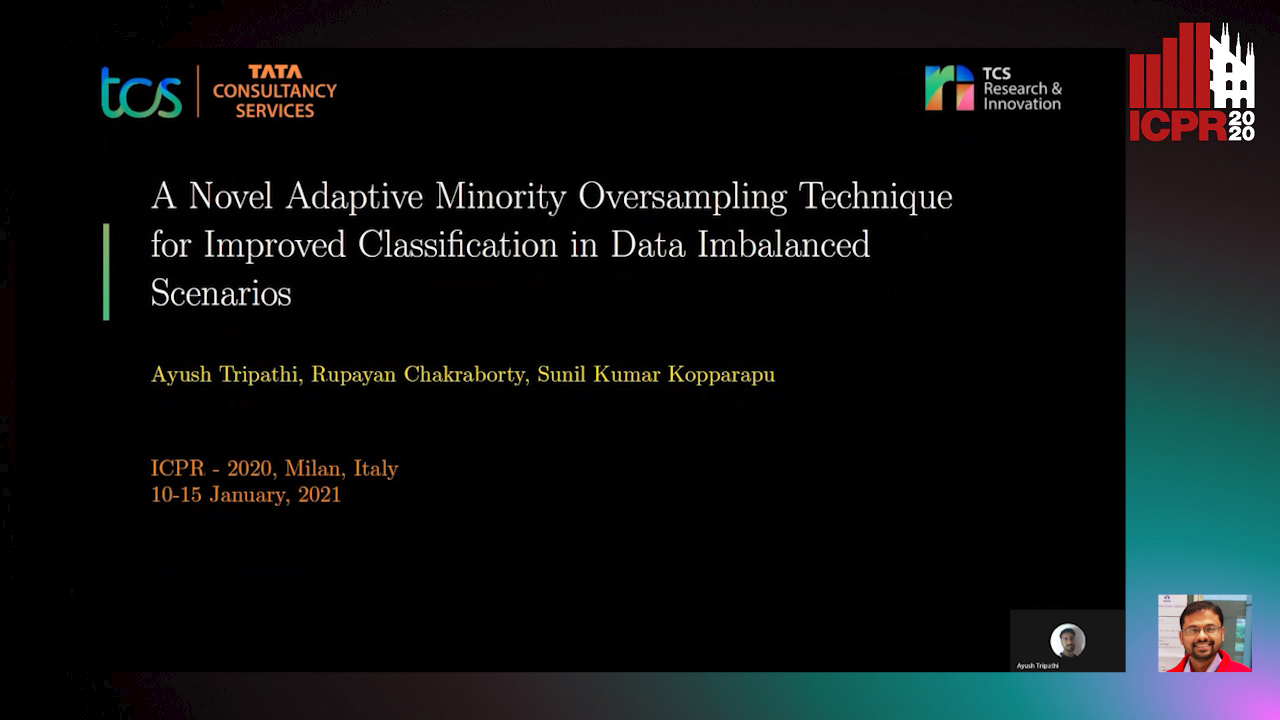
Auto-TLDR; Synthetic Minority OverSampling Technique for Imbalanced Data
Abstract Slides Poster Similar
On Learning Random Forests for Random Forest Clustering
Manuele Bicego, Francisco Escolano
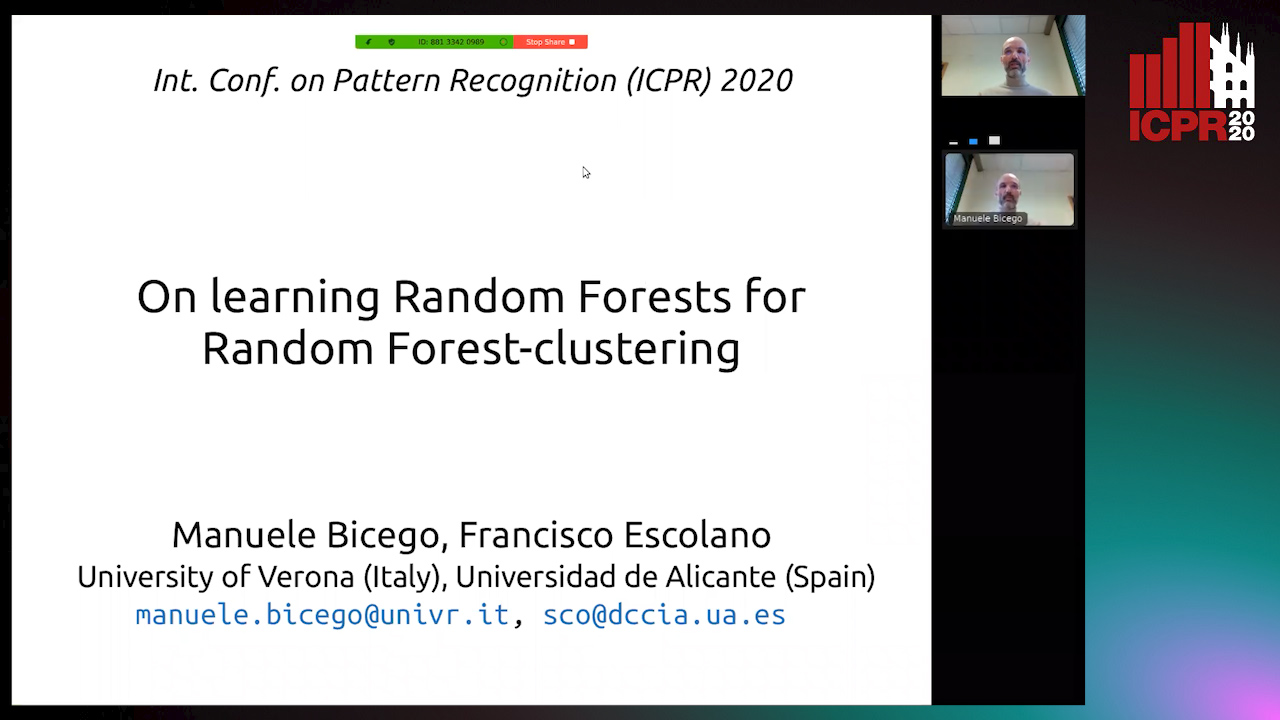
Auto-TLDR; Learning Random Forests for Clustering
Abstract Slides Poster Similar
Using Meta Labels for the Training of Weighting Models in a Sample-Specific Late Fusion Classification Architecture
Peter Bellmann, Patrick Thiam, Friedhelm Schwenker
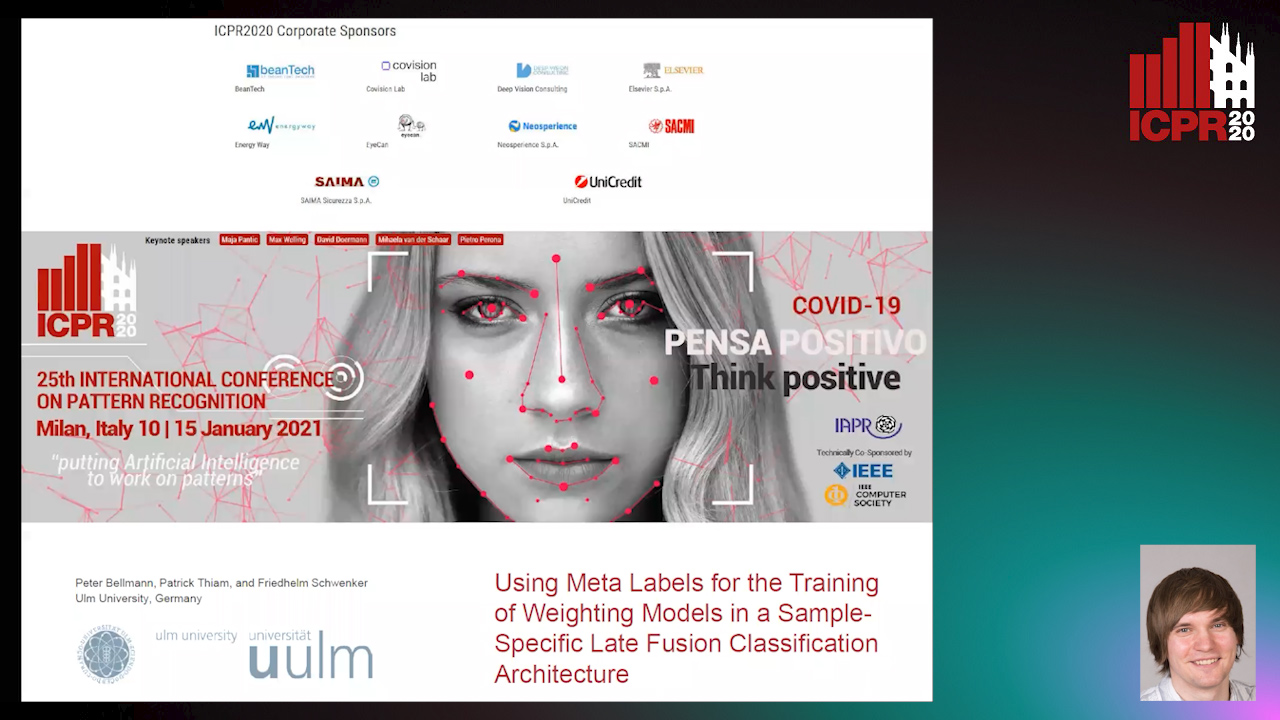
Auto-TLDR; A Late Fusion Architecture for Multiple Classifier Systems
Abstract Slides Poster Similar
Classifier Pool Generation Based on a Two-Level Diversity Approach
Marcos Monteiro, Alceu Britto, Jean Paul Barddal, Luiz Oliveira, Robert Sabourin
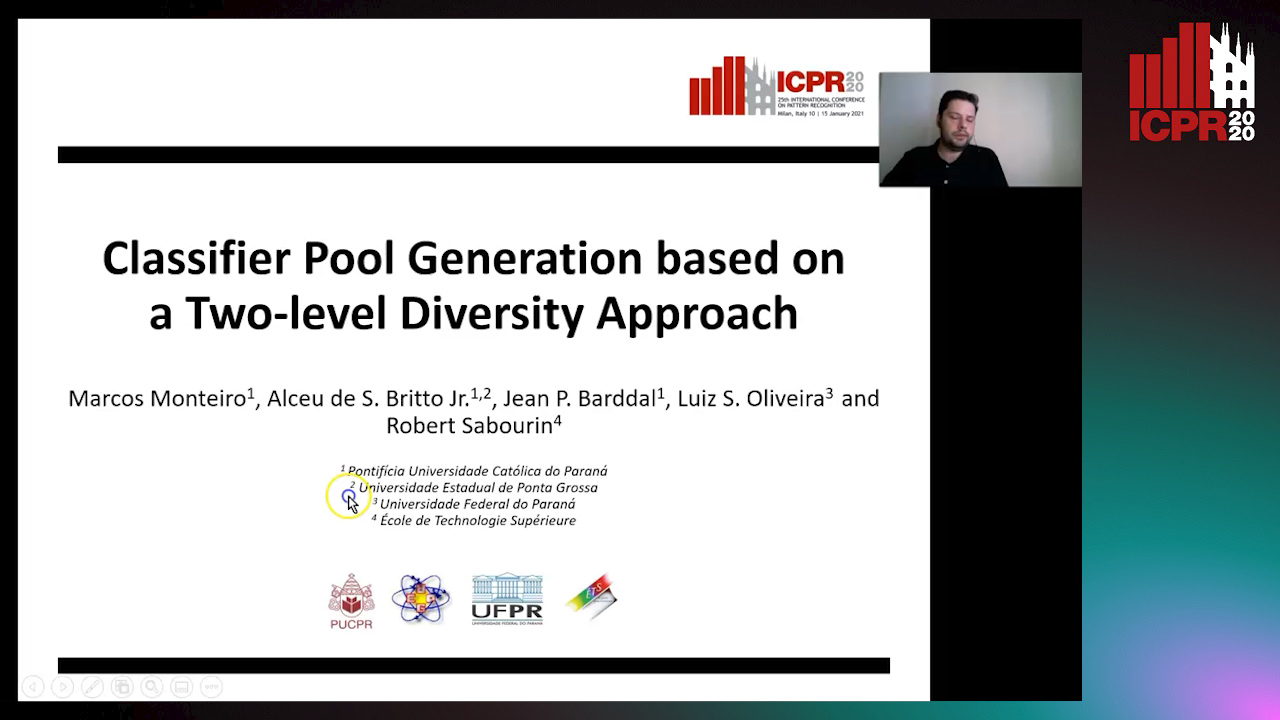
Auto-TLDR; Diversity-Based Pool Generation with Dynamic Classifier Selection and Dynamic Ensemble Selection
Abstract Slides Poster Similar
Creating Classifier Ensembles through Meta-Heuristic Algorithms for Aerial Scene Classification
Álvaro Roberto Ferreira Jr., Gustavo Gustavo Henrique De Rosa, Joao Paulo Papa, Gustavo Carneiro, Fabio Augusto Faria
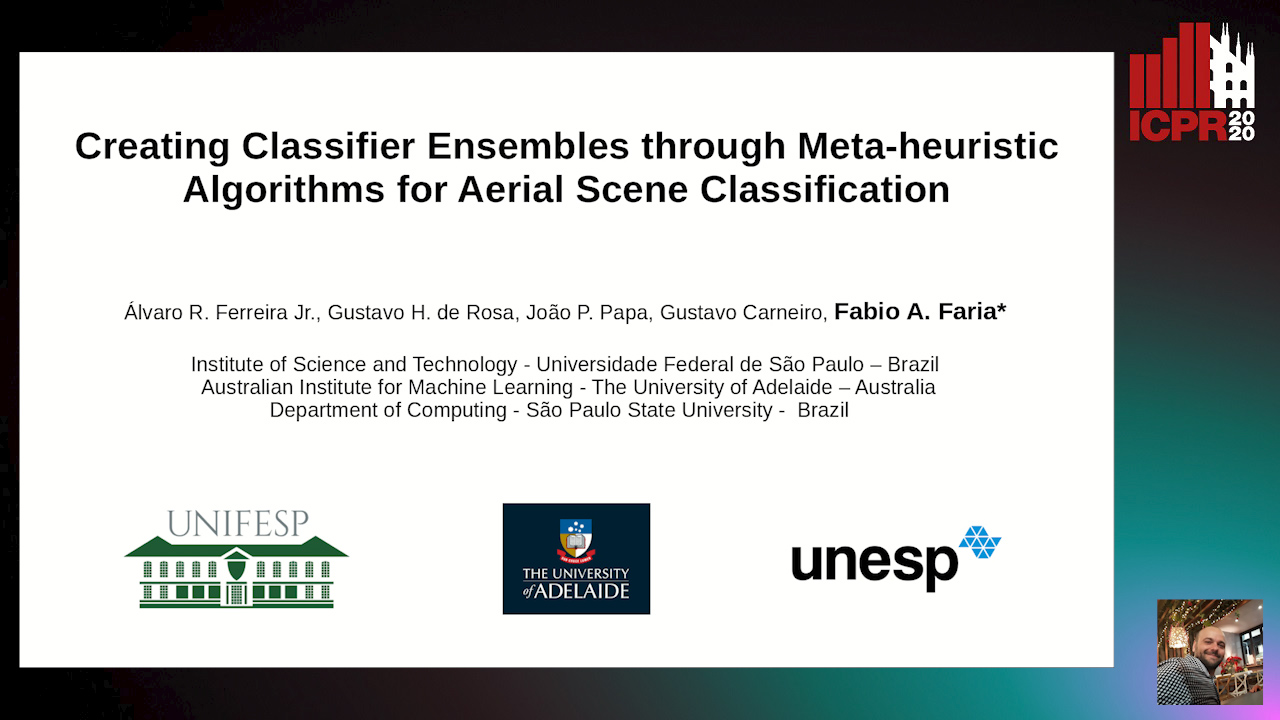
Auto-TLDR; Univariate Marginal Distribution Algorithm for Aerial Scene Classification Using Meta-Heuristic Optimization
Abstract Slides Poster Similar
Class-Incremental Learning with Pre-Allocated Fixed Classifiers
Federico Pernici, Matteo Bruni, Claudio Baecchi, Francesco Turchini, Alberto Del Bimbo
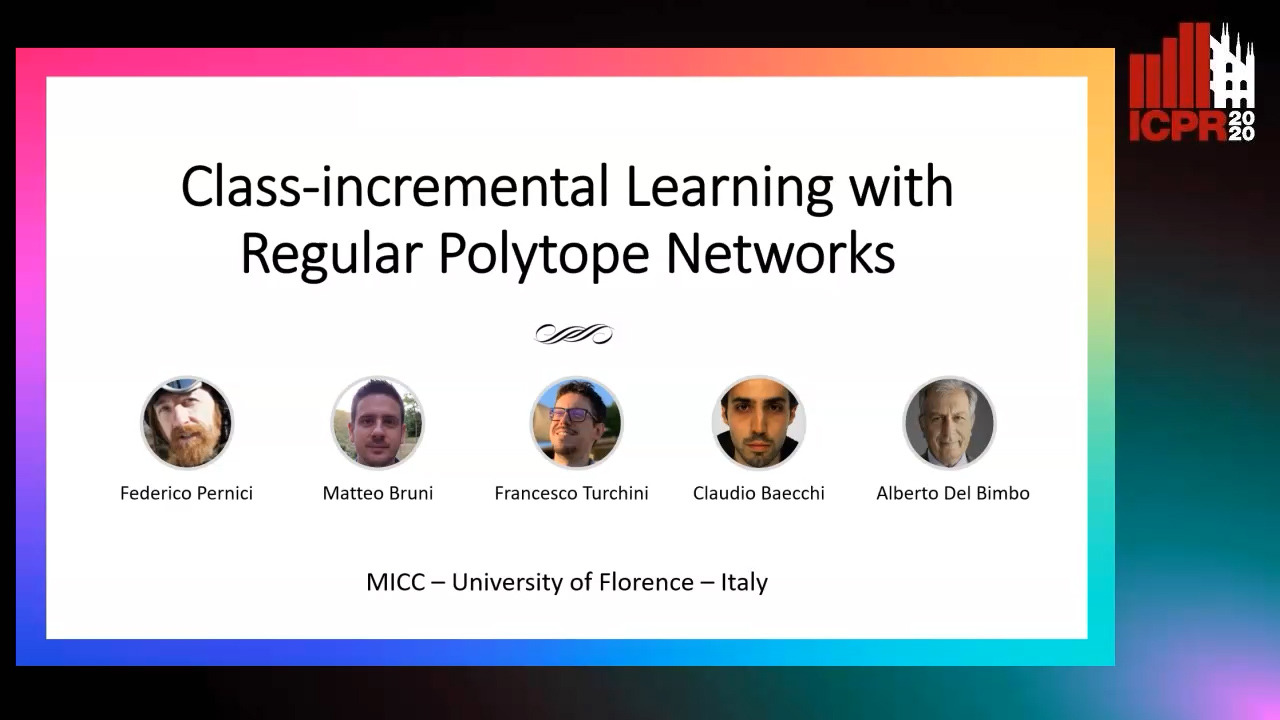
Auto-TLDR; Class-Incremental Learning with Pre-allocated Output Nodes for Fixed Classifier
Abstract Slides Poster Similar
3CS Algorithm for Efficient Gaussian Process Model Retrieval
Fabian Berns, Kjeld Schmidt, Ingolf Bracht, Christian Beecks
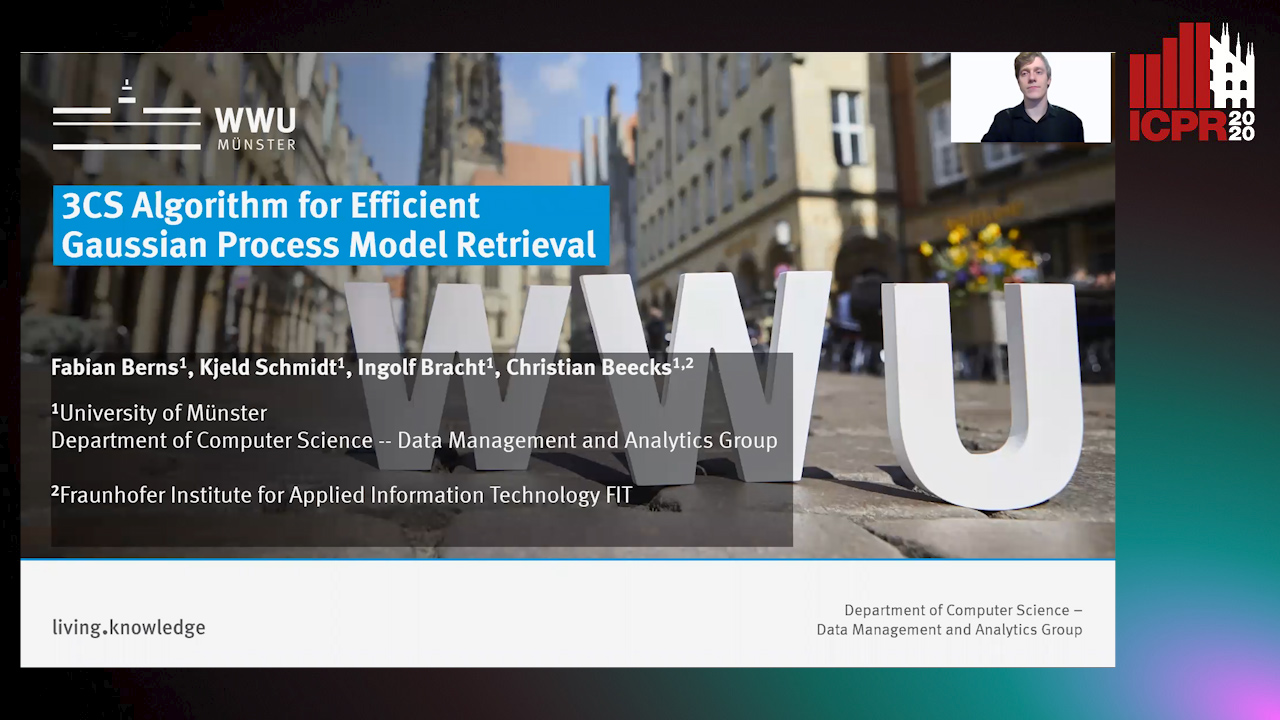
Auto-TLDR; Efficient retrieval of Gaussian Process Models for large-scale data using divide-&-conquer-based approach
Abstract Slides Poster Similar
A Novel Random Forest Dissimilarity Measure for Multi-View Learning
Hongliu Cao, Simon Bernard, Robert Sabourin, Laurent Heutte
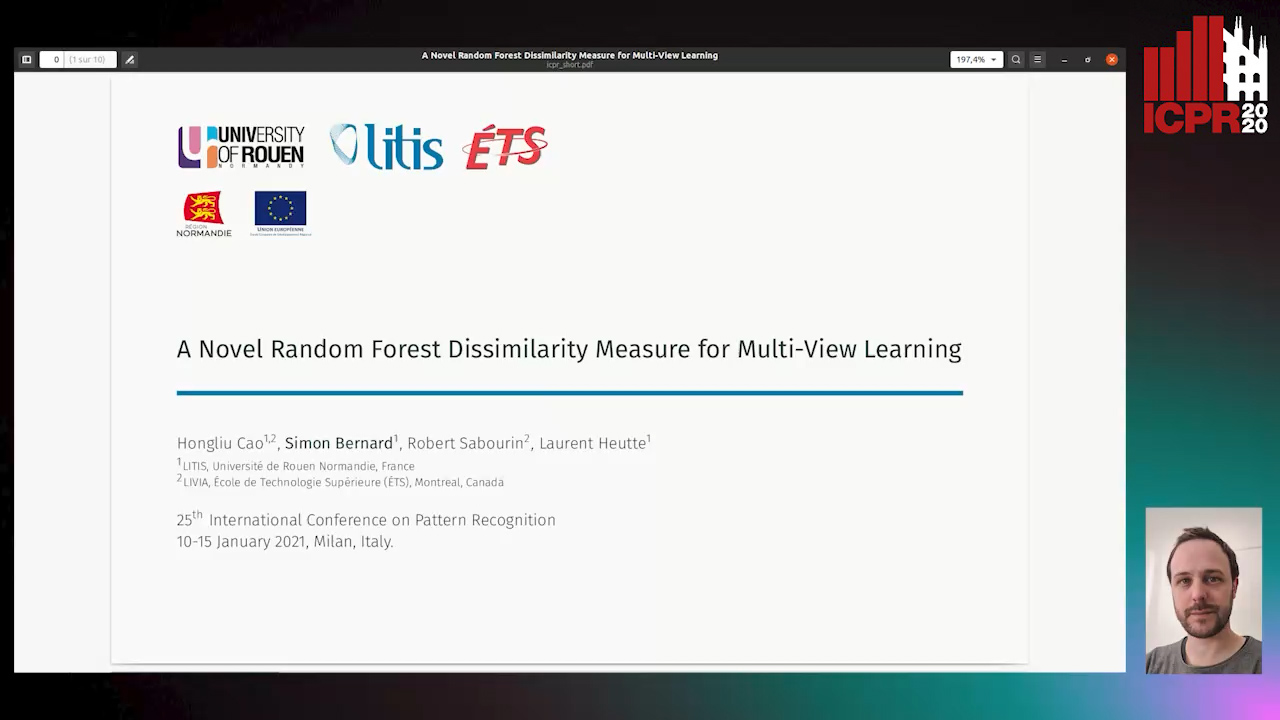
Auto-TLDR; Multi-view Learning with Random Forest Relation Measure and Instance Hardness
Abstract Slides Poster Similar
Learning with Delayed Feedback
Pranavan Theivendiram, Terence Sim
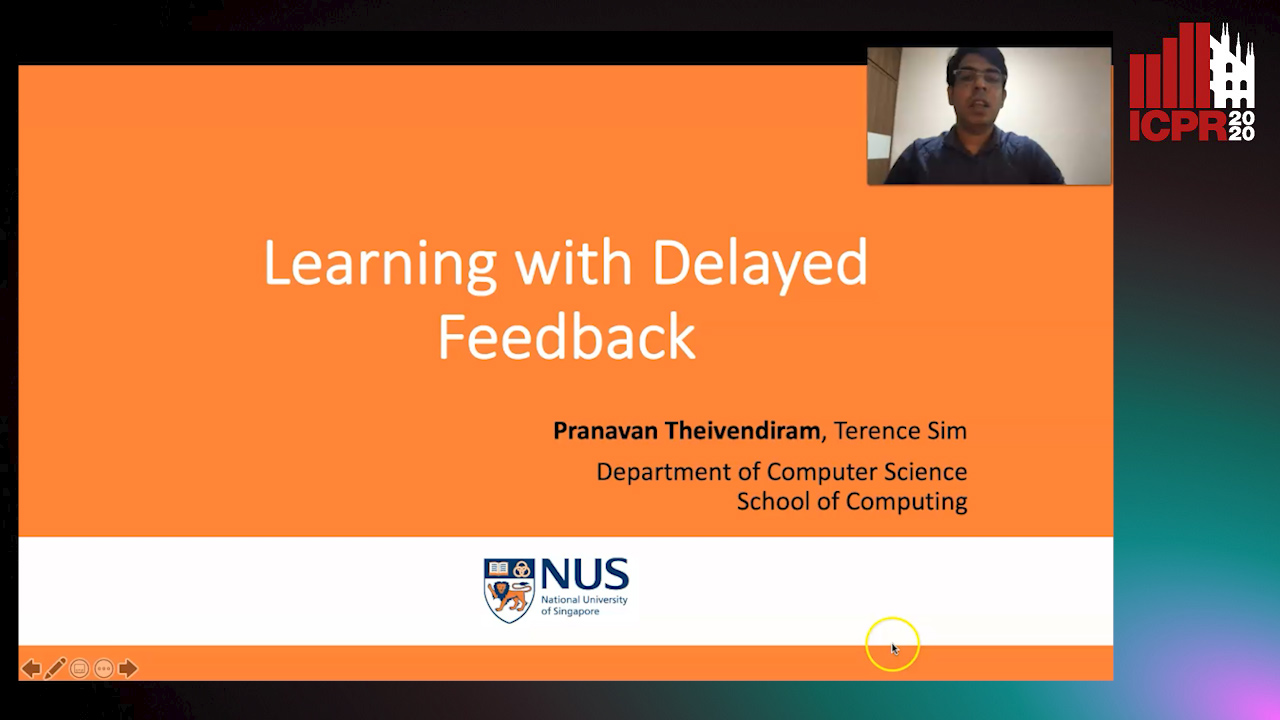
Auto-TLDR; Unsupervised Machine Learning with Delayed Feedback
Abstract Slides Poster Similar
Hierarchical Routing Mixture of Experts
Wenbo Zhao, Yang Gao, Shahan Ali Memon, Bhiksha Raj, Rita Singh
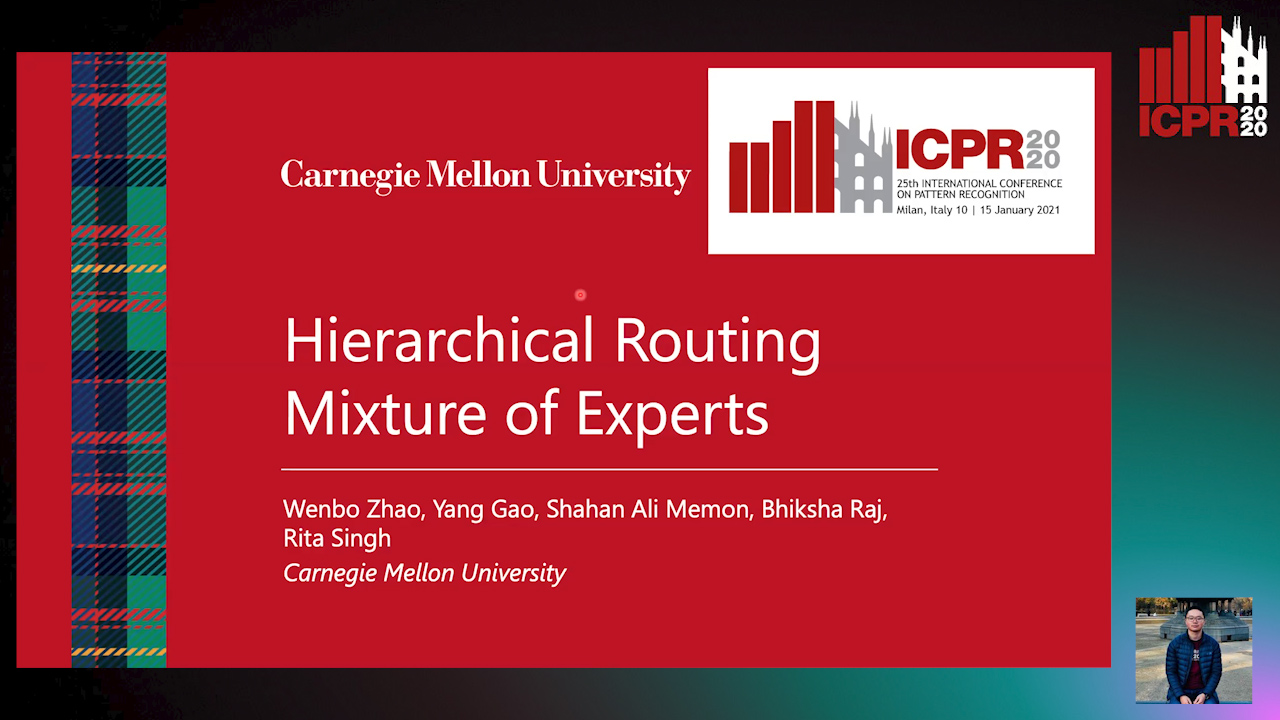
Auto-TLDR; A Binary Tree-structured Hierarchical Routing Mixture of Experts for Regression
Abstract Slides Poster Similar
Aggregating Dependent Gaussian Experts in Local Approximation
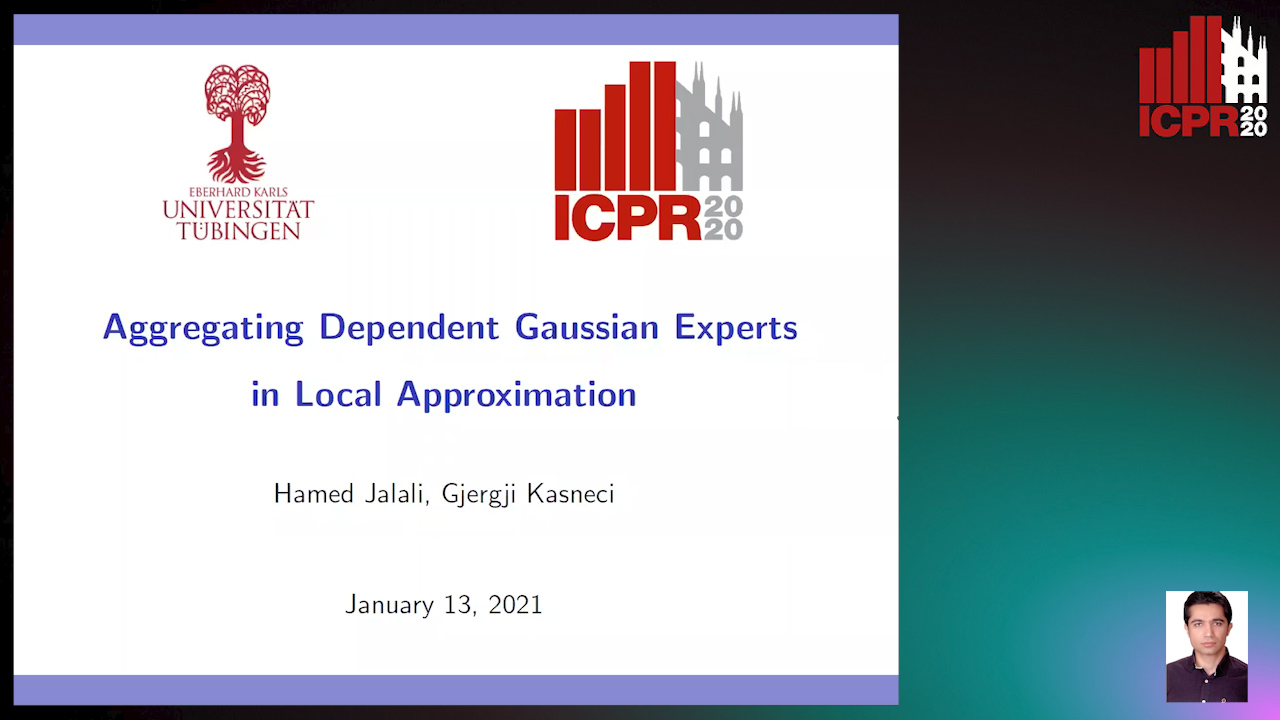
Auto-TLDR; A novel approach for aggregating the Gaussian experts by detecting strong violations of conditional independence
Abstract Slides Poster Similar
ARCADe: A Rapid Continual Anomaly Detector
Ahmed Frikha, Denis Krompass, Volker Tresp
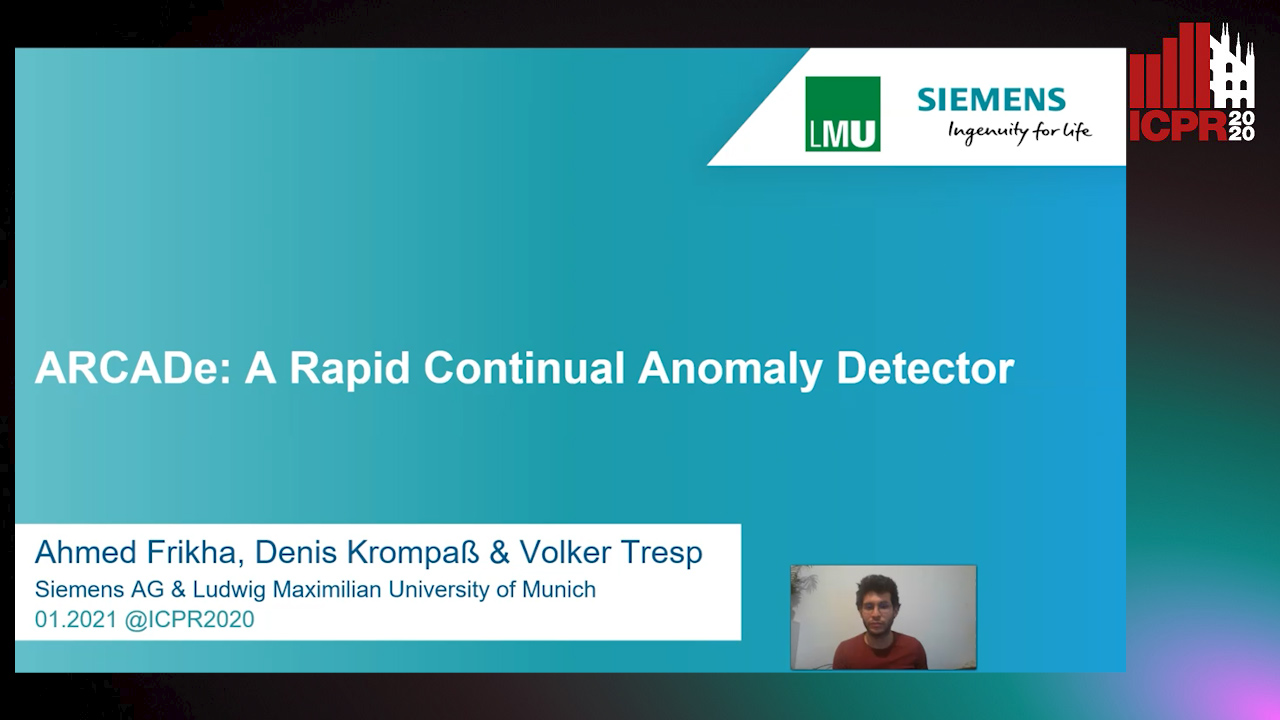
Auto-TLDR; ARCADe: A Meta-Learning Approach for Continuous Anomaly Detection
Abstract Slides Poster Similar
Explainable Online Validation of Machine Learning Models for Practical Applications
Wolfgang Fuhl, Yao Rong, Thomas Motz, Michael Scheidt, Andreas Markus Hartel, Andreas Koch, Enkelejda Kasneci
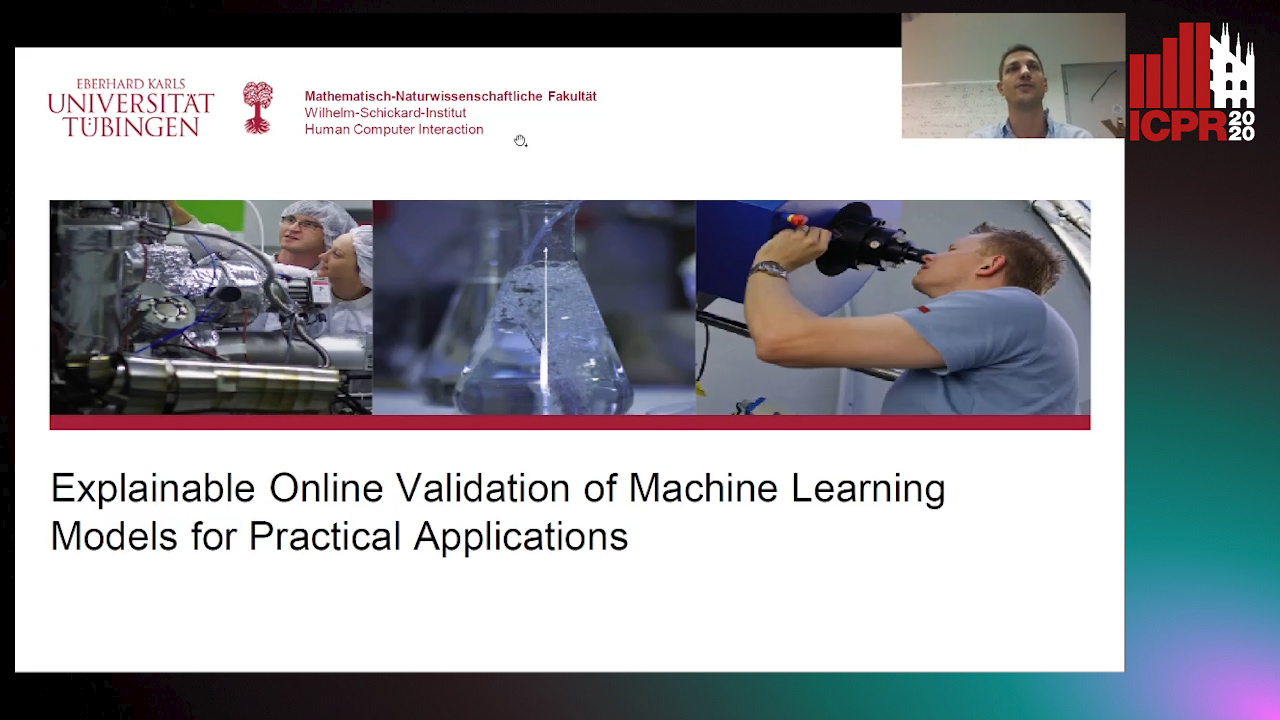
Auto-TLDR; A Reformulation of Regression and Classification for Machine Learning Algorithm Validation
Abstract Slides Poster Similar
Adversarial Encoder-Multi-Task-Decoder for Multi-Stage Processes
Andre Mendes, Julian Togelius, Leandro Dos Santos Coelho

Auto-TLDR; Multi-Task Learning and Semi-Supervised Learning for Multi-Stage Processes
Rethinking Experience Replay: A Bag of Tricks for Continual Learning
Pietro Buzzega, Matteo Boschini, Angelo Porrello, Simone Calderara
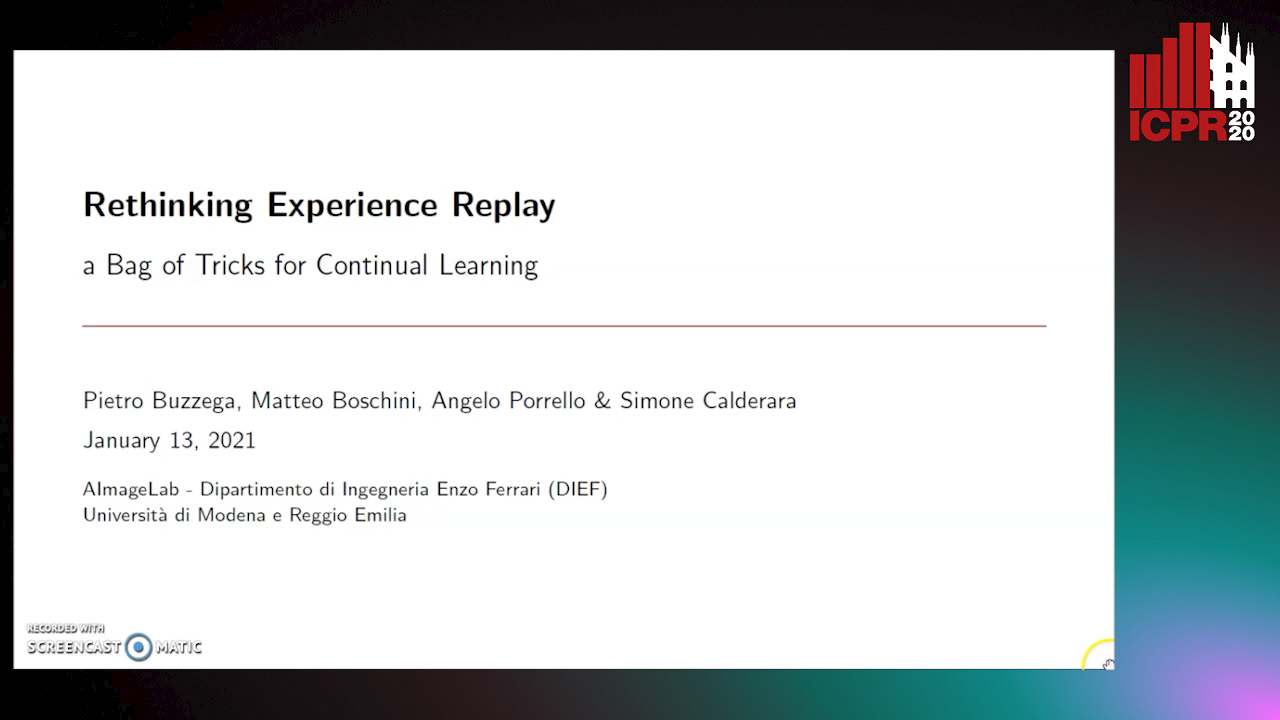
Auto-TLDR; Experience Replay for Continual Learning: A Practical Approach
Abstract Slides Poster Similar
MD-kNN: An Instance-Based Approach for Multi-Dimensional Classification
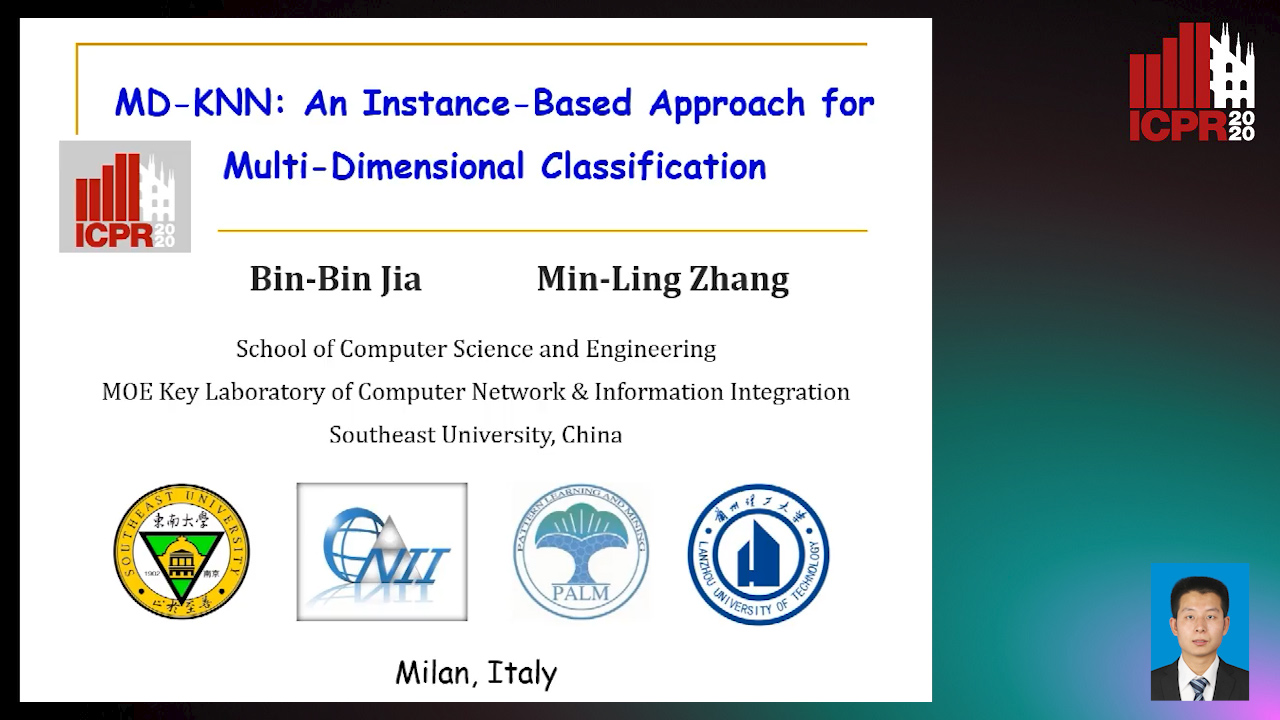
Auto-TLDR; MD-kNN: Adapting Instance-based Techniques for Multi-dimensional Classification
Abstract Slides Poster Similar
Memetic Evolution of Training Sets with Adaptive Radial Basis Kernels for Support Vector Machines
Jakub Nalepa, Wojciech Dudzik, Michal Kawulok
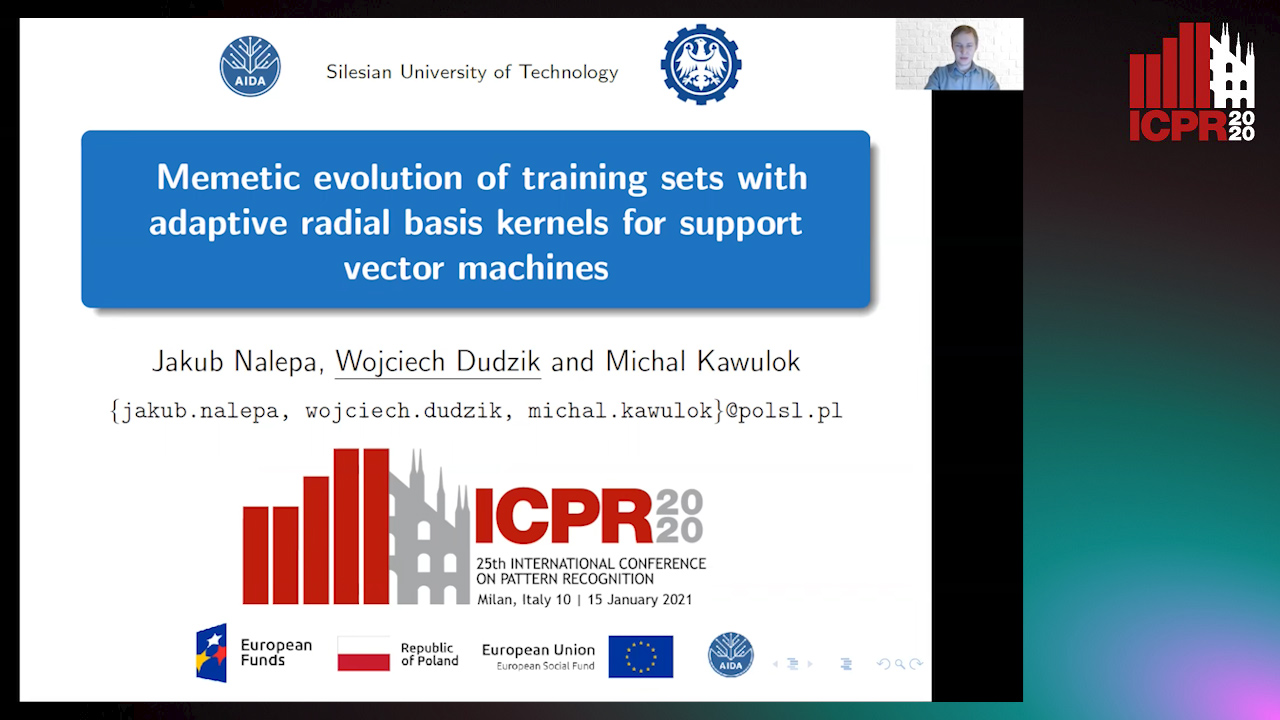
Auto-TLDR; Memetic Algorithm for Evolving Support Vector Machines with Adaptive Kernels
Abstract Slides Poster Similar
An Adaptive Video-To-Video Face Identification System Based on Self-Training
Eric Lopez-Lopez, Carlos V. Regueiro, Xosé M. Pardo
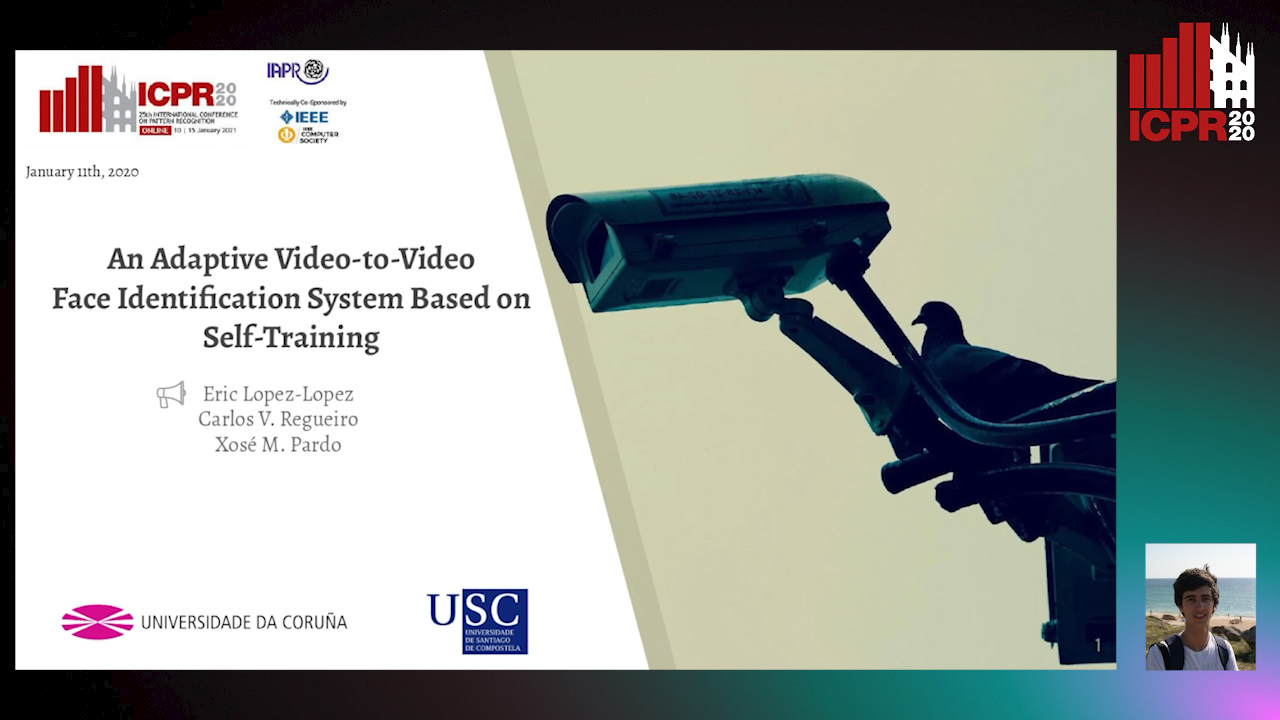
Auto-TLDR; Adaptive Video-to-Video Face Recognition using Dynamic Ensembles of SVM's
Abstract Slides Poster Similar
Emerging Relation Network and Task Embedding for Multi-Task Regression Problems
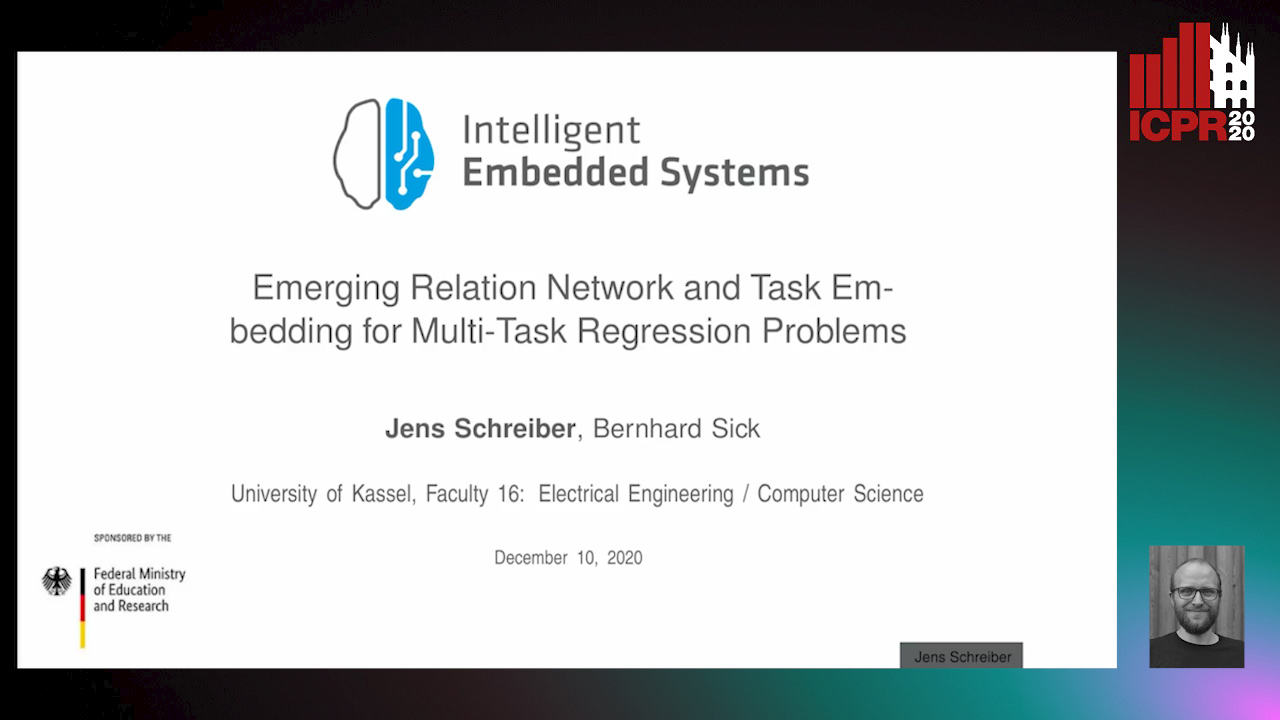
Auto-TLDR; A Comparative Study of Multi-Task Learning for Non-linear Time Series Problems
Abstract Slides Poster Similar
Position-Aware Safe Boundary Interpolation Oversampling
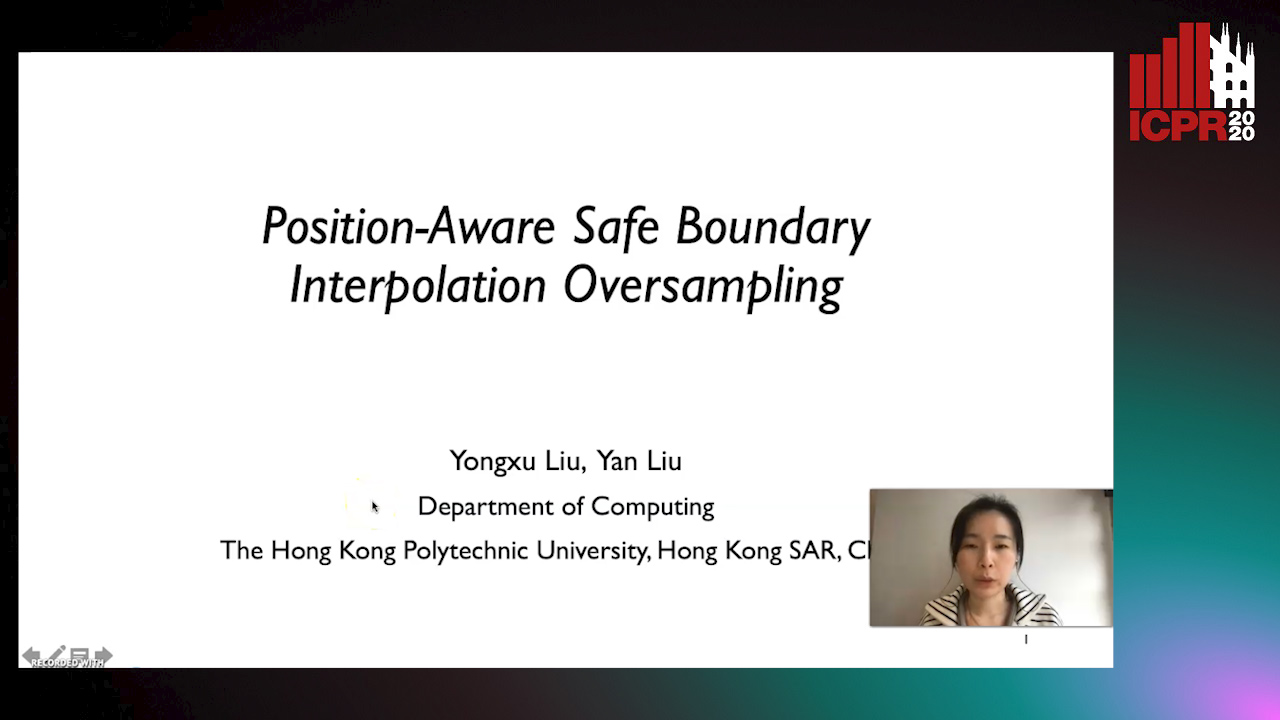
Auto-TLDR; PABIO: Position-Aware Safe Boundary Interpolation-Based Oversampling for Imbalanced Data
Abstract Slides Poster Similar
MetaMix: Improved Meta-Learning with Interpolation-based Consistency Regularization
Yangbin Chen, Yun Ma, Tom Ko, Jianping Wang, Qing Li
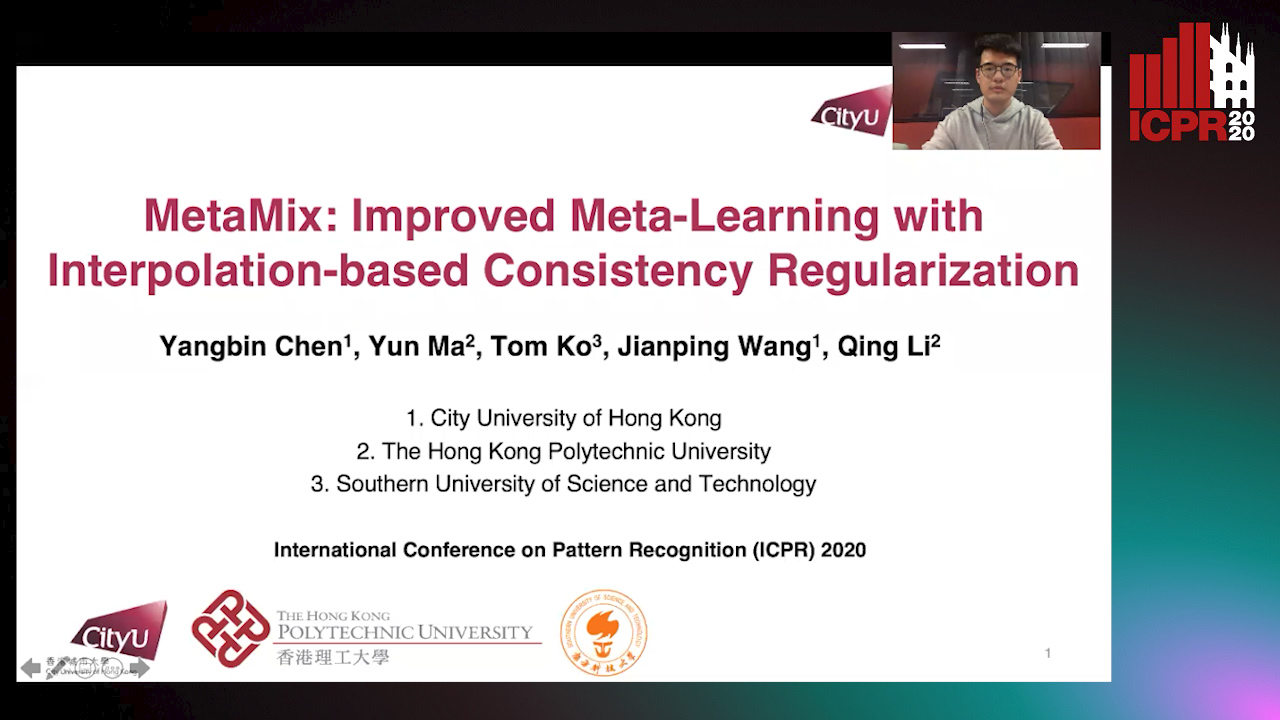
Auto-TLDR; MetaMix: A Meta-Agnostic Meta-Learning Algorithm for Few-Shot Classification
Abstract Slides Poster Similar
A Heuristic-Based Decision Tree for Connected Components Labeling of 3D Volumes
Maximilian Söchting, Stefano Allegretti, Federico Bolelli, Costantino Grana
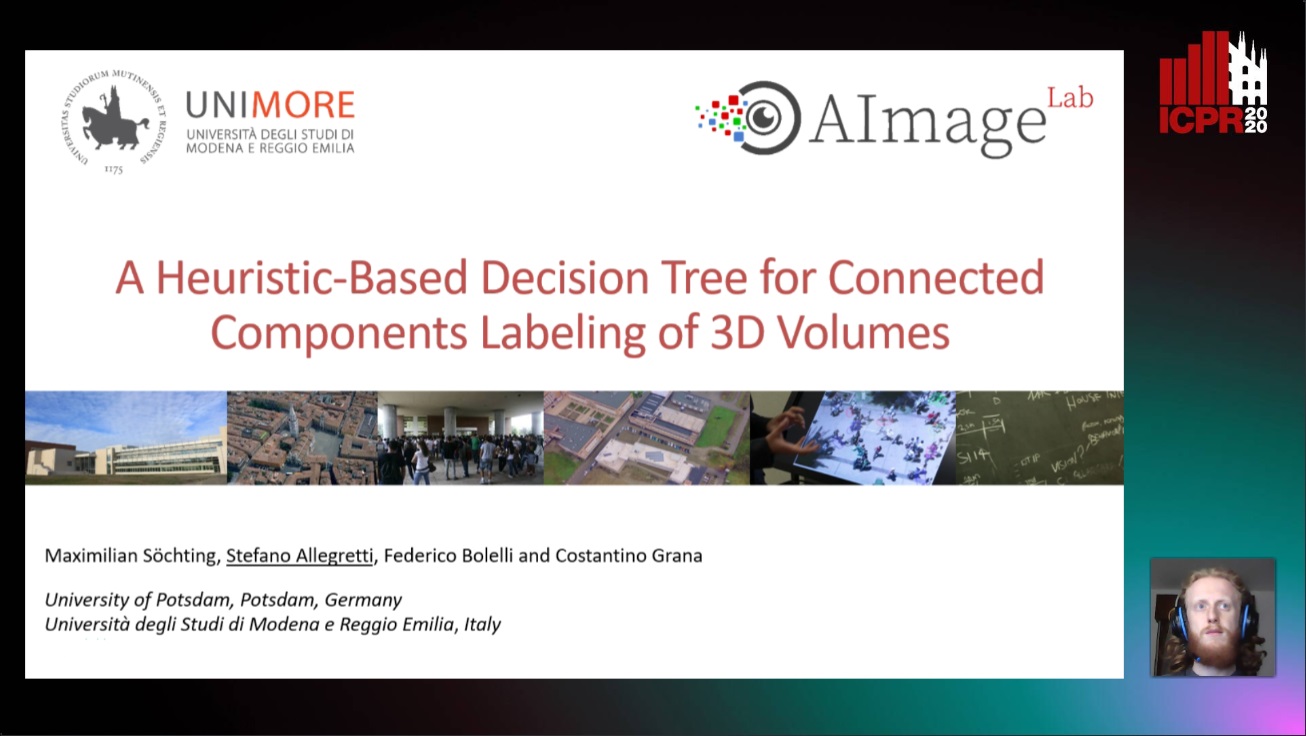
Auto-TLDR; Entropy Partitioning Decision Tree for Connected Components Labeling
Abstract Slides Poster Similar
Multi-Attribute Learning with Highly Imbalanced Data
Lady Viviana Beltran Beltran, Mickaël Coustaty, Nicholas Journet, Juan C. Caicedo, Antoine Doucet
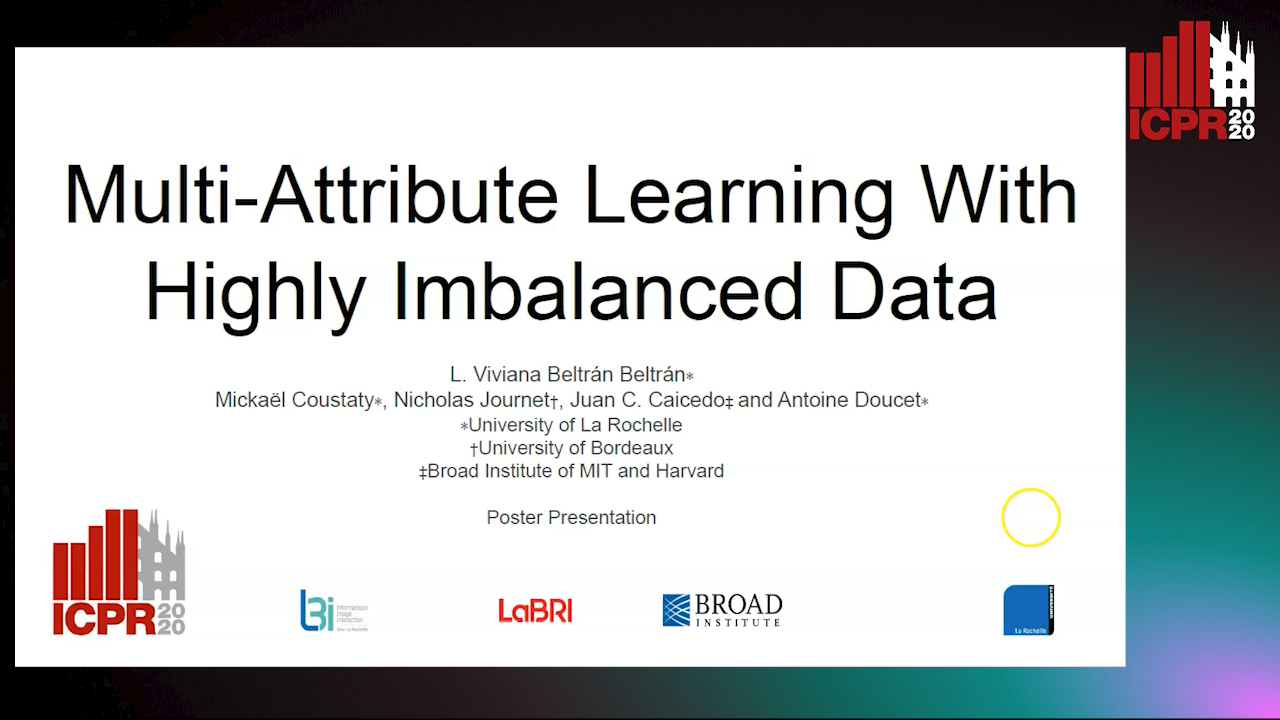
Auto-TLDR; Data Imbalance in Multi-Attribute Deep Learning Models: Adaptation to face each one of the problems derived from imbalance
Abstract Slides Poster Similar
Automatic Annotation of Corpora for Emotion Recognition through Facial Expressions Analysis
Alex Mircoli, Claudia Diamantini, Domenico Potena, Emanuele Storti
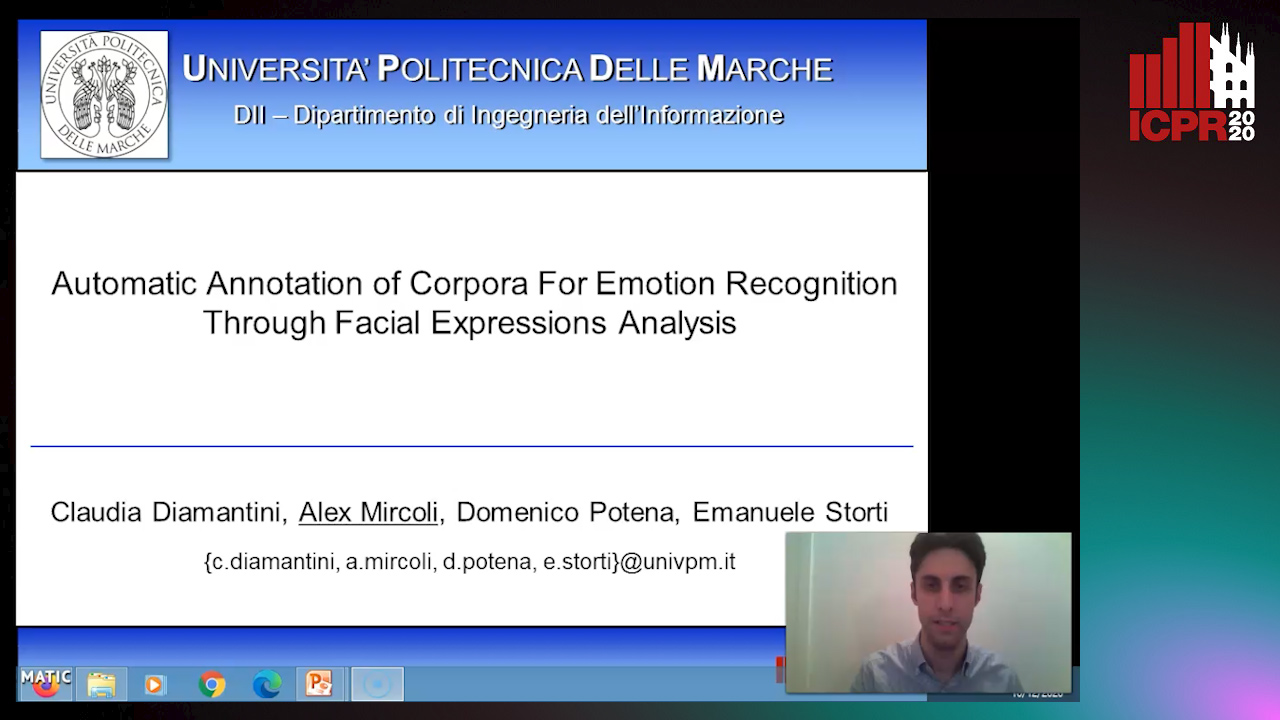
Auto-TLDR; Automatic annotation of video subtitles on the basis of facial expressions using machine learning algorithms
Abstract Slides Poster Similar
Mean Decision Rules Method with Smart Sampling for Fast Large-Scale Binary SVM Classification
Alexandra Makarova, Mikhail Kurbakov, Valentina Sulimova
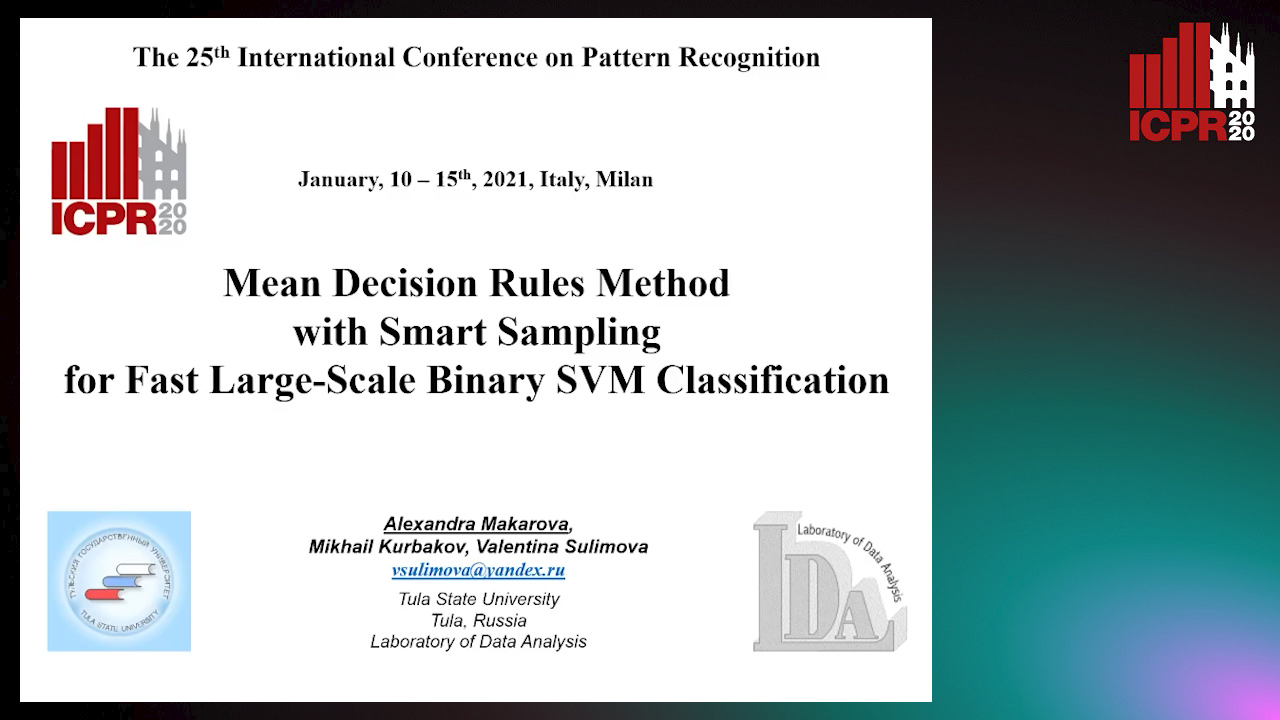
Auto-TLDR; Improving Mean Decision Rule for Large-Scale Binary SVM Problems
Abstract Slides Poster Similar
Adaptive Matching of Kernel Means
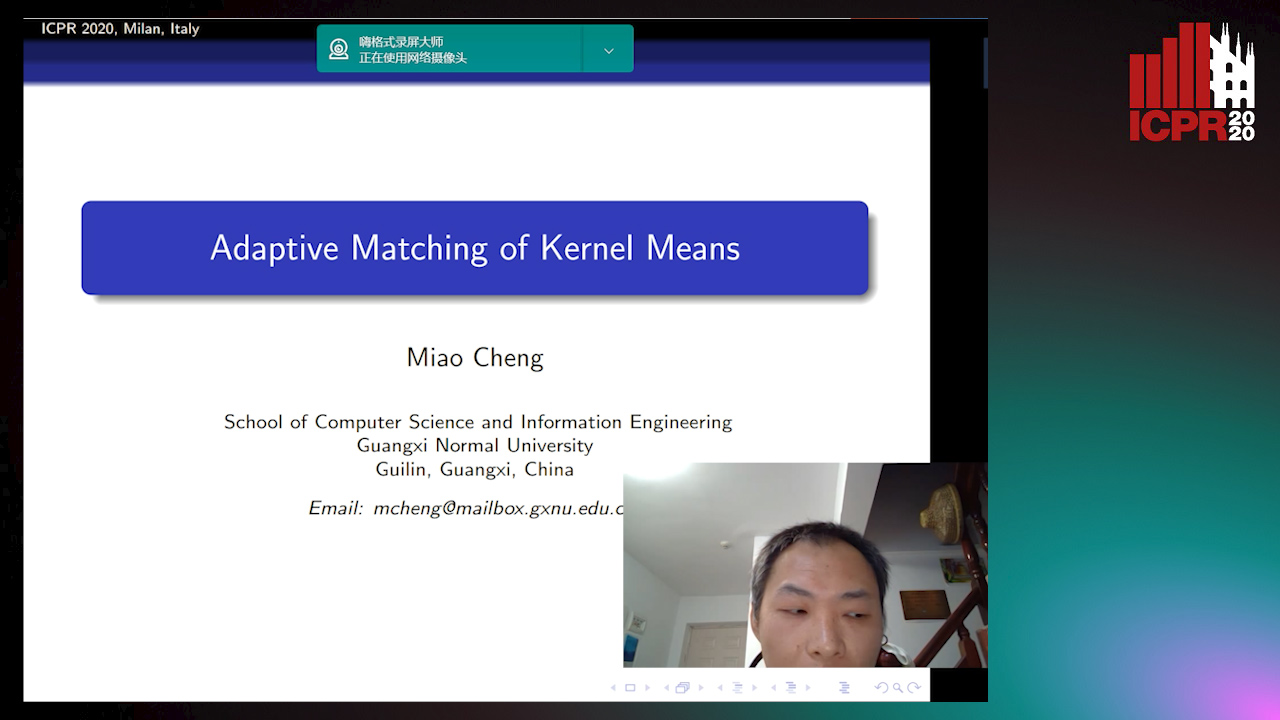
Auto-TLDR; Adaptive Matching of Kernel Means for Knowledge Discovery and Feature Learning
Abstract Slides Poster Similar
Location Prediction in Real Homes of Older Adults based on K-Means in Low-Resolution Depth Videos
Simon Simonsson, Flávia Dias Casagrande, Evi Zouganeli
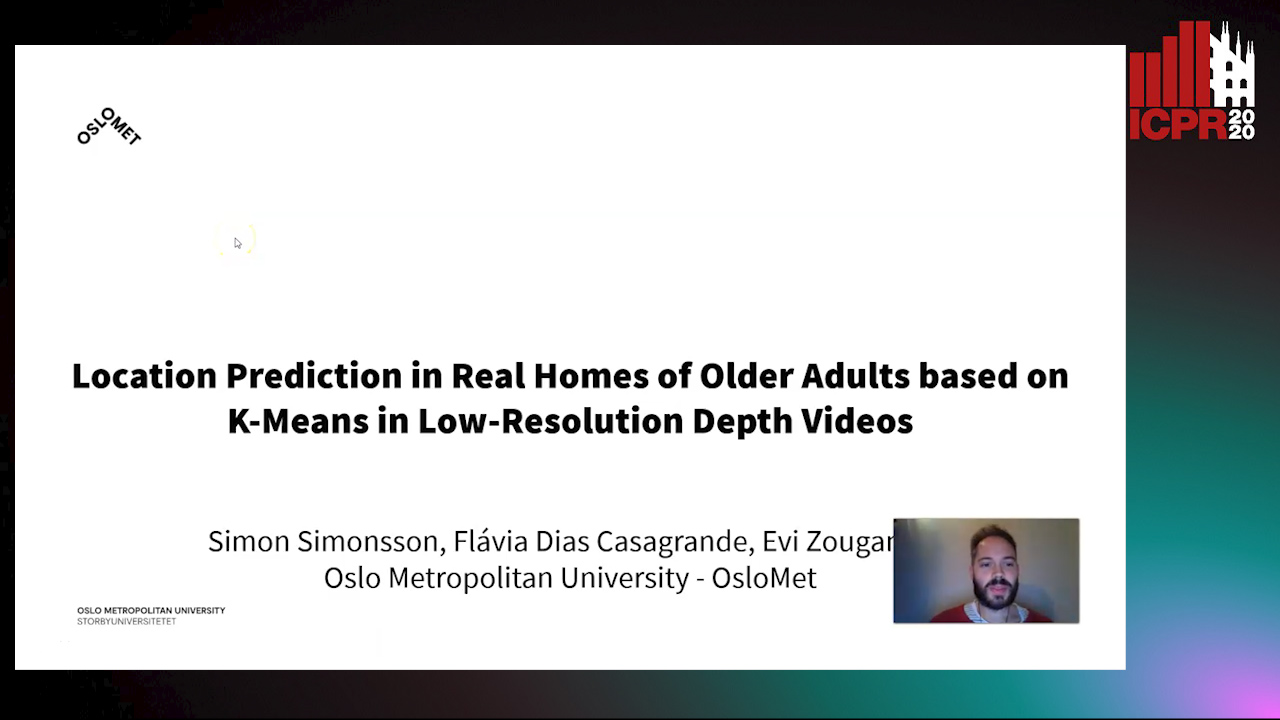
Auto-TLDR; Semi-supervised Learning for Location Recognition and Prediction in Smart Homes using Depth Video Cameras
Abstract Slides Poster Similar
Evaluation of Anomaly Detection Algorithms for the Real-World Applications
Marija Ivanovska, Domen Tabernik, Danijel Skocaj, Janez Pers
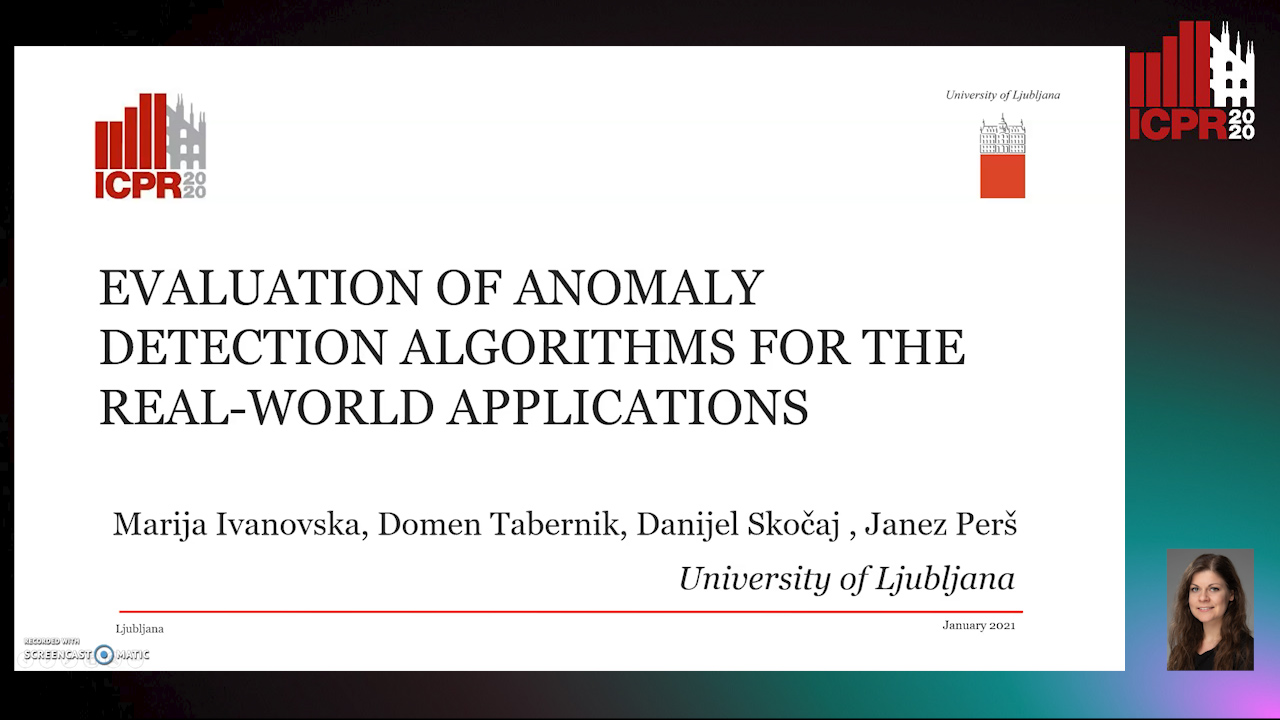
Auto-TLDR; Evaluating Anomaly Detection Algorithms for Practical Applications
Abstract Slides Poster Similar
Comparison of Stacking-Based Classifier Ensembles Using Euclidean and Riemannian Geometries
Vitaliy Tayanov, Adam Krzyzak, Ching Y Suen
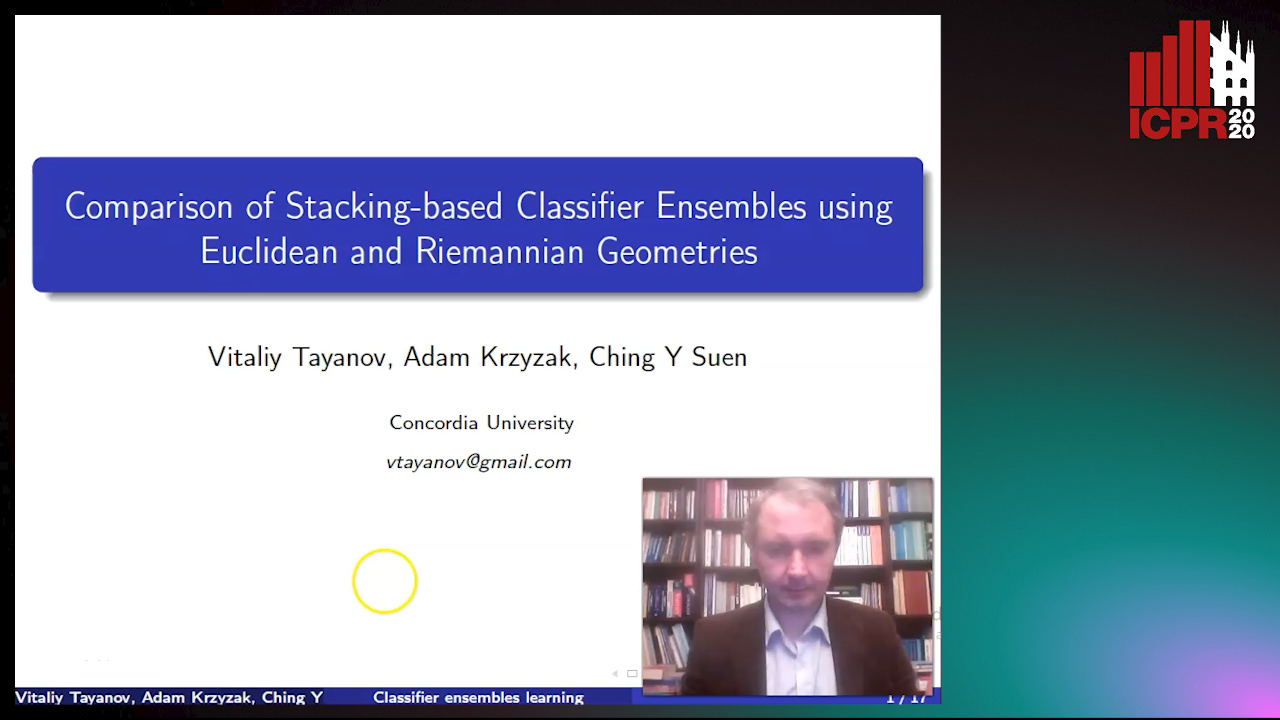
Auto-TLDR; Classifier Stacking in Riemannian Geometries using Cascades of Random Forest and Extra Trees
Abstract Slides Poster Similar
Minority Class Oriented Active Learning for Imbalanced Datasets
Umang Aggarwal, Adrian Popescu, Celine Hudelot
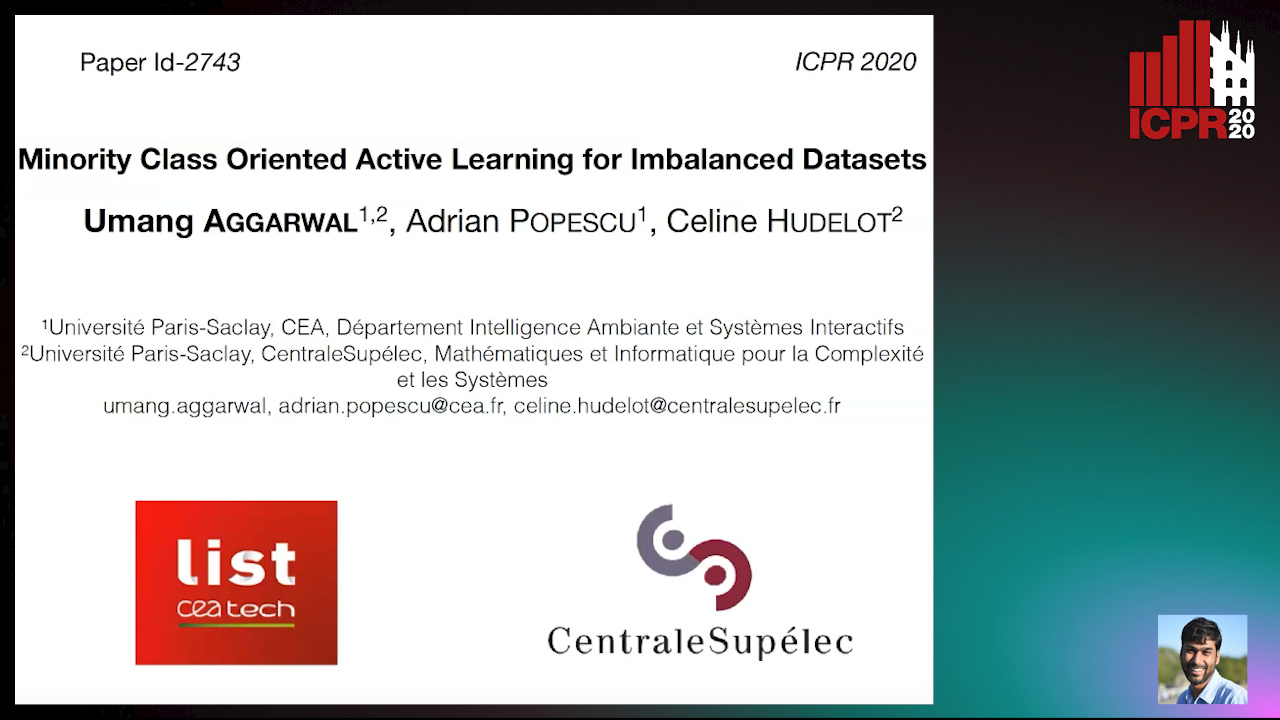
Auto-TLDR; Active Learning for Imbalanced Datasets
Abstract Slides Poster Similar
Seasonal Inhomogeneous Nonconsecutive Arrival Process Search and Evaluation
Kimberly Holmgren, Paul Gibby, Joseph Zipkin
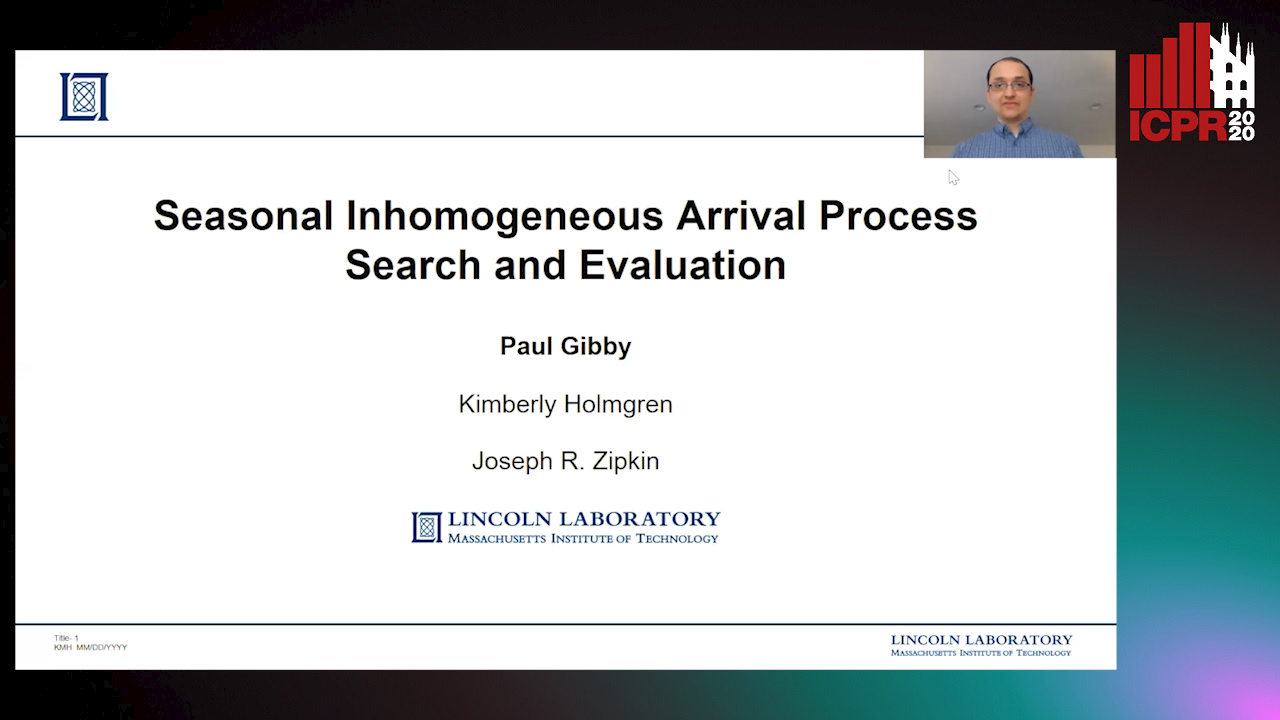
Auto-TLDR; SINAPSE: Fitting a Sparse Time Series Model to Seasonal Data
Abstract Slides Poster Similar
Trainable Spectrally Initializable Matrix Transformations in Convolutional Neural Networks
Michele Alberti, Angela Botros, Schuetz Narayan, Rolf Ingold, Marcus Liwicki, Mathias Seuret
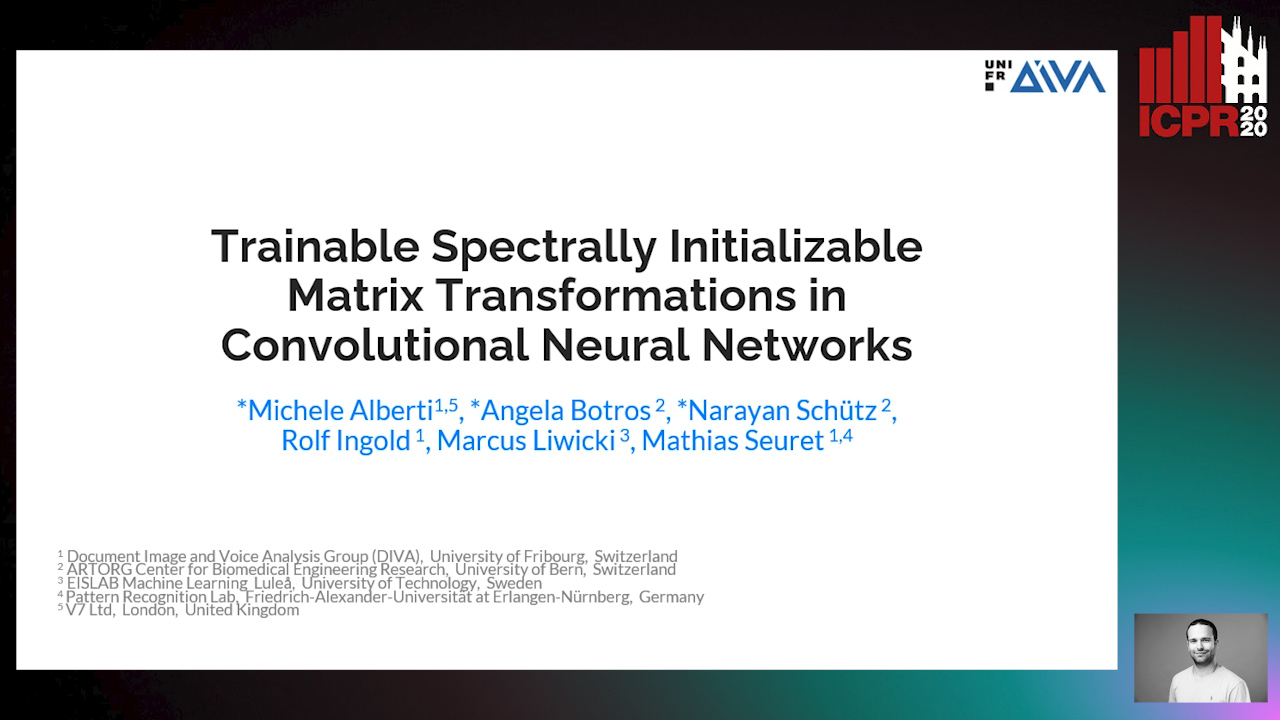
Auto-TLDR; Trainable and Spectrally Initializable Matrix Transformations for Neural Networks
Abstract Slides Poster Similar
A Close Look at Deep Learning with Small Data
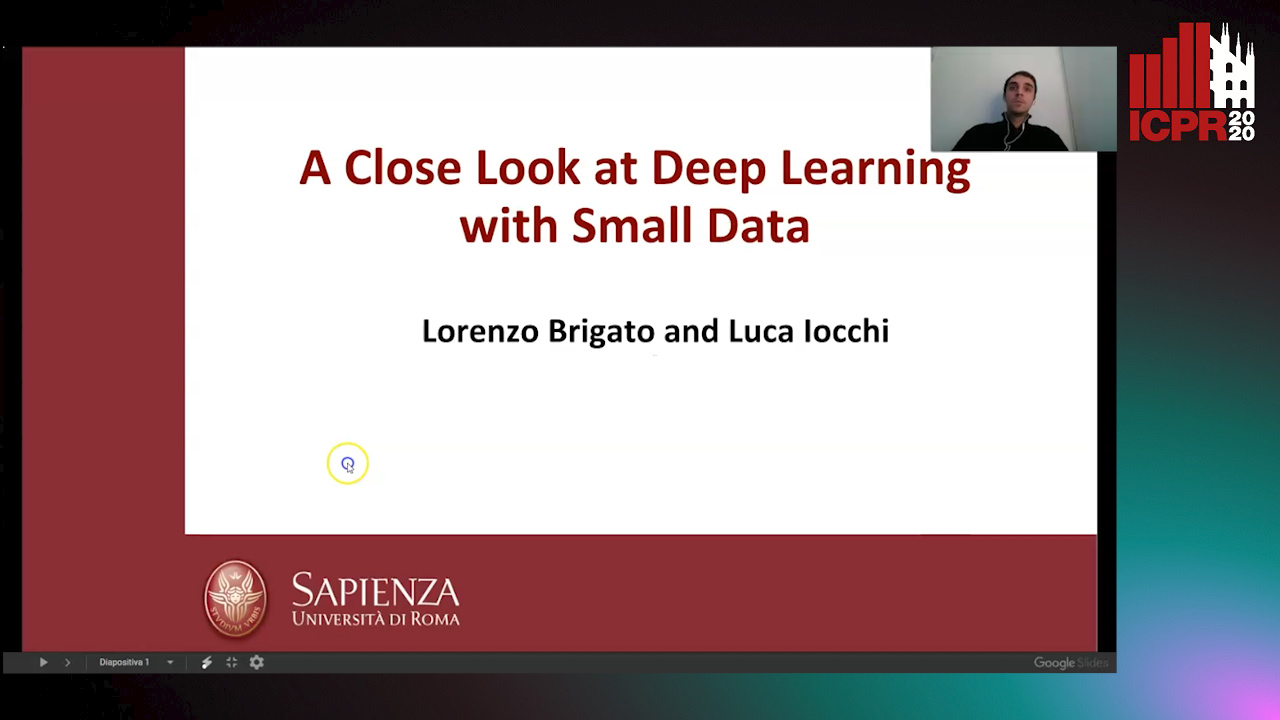
Auto-TLDR; Low-Complex Neural Networks for Small Data Conditions
Abstract Slides Poster Similar
Factor Screening Using Bayesian Active Learning and Gaussian Process Meta-Modelling
Cheng Li, Santu Rana, Andrew William Gill, Dang Nguyen, Sunil Kumar Gupta, Svetha Venkatesh

Auto-TLDR; Data-Efficient Bayesian Active Learning for Factor Screening in Combat Simulations
Relative Feature Importance
Gunnar König, Christoph Molnar, Bernd Bischl, Moritz Grosse-Wentrup
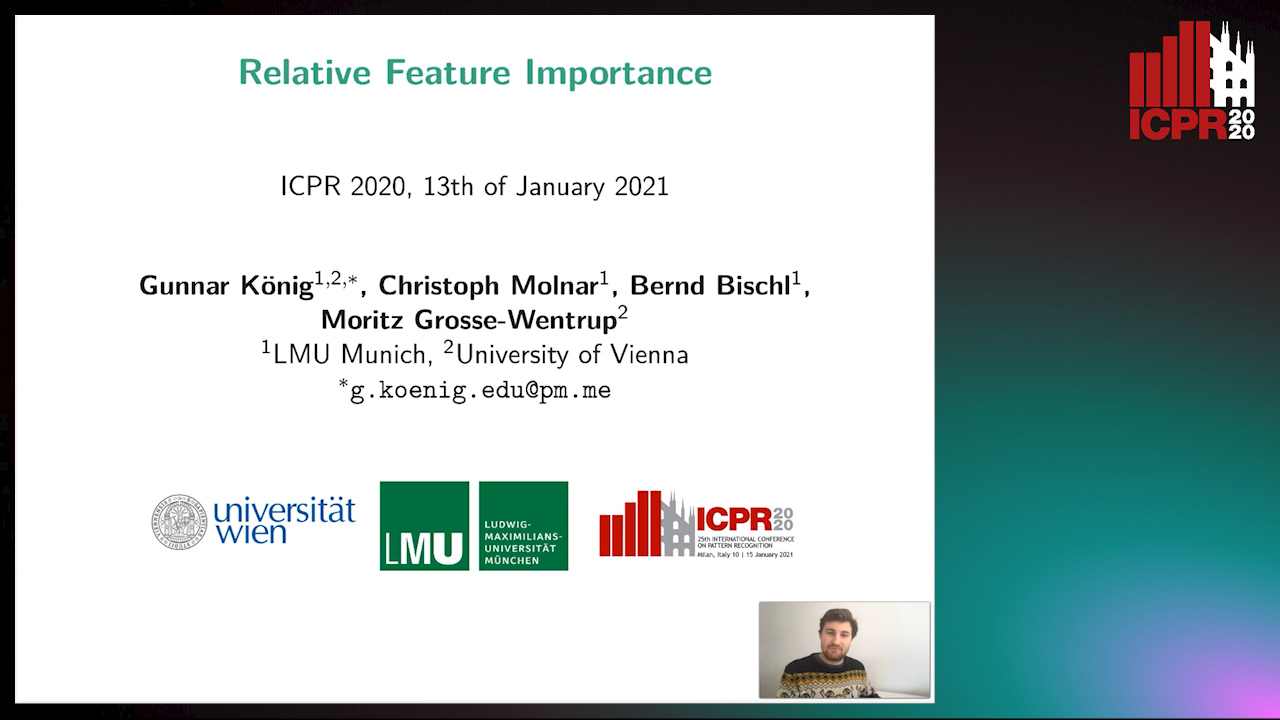
Auto-TLDR; Relative Feature Importance for Interpretable Machine Learning
Deep Learning on Active Sonar Data Using Bayesian Optimization for Hyperparameter Tuning
Henrik Berg, Karl Thomas Hjelmervik
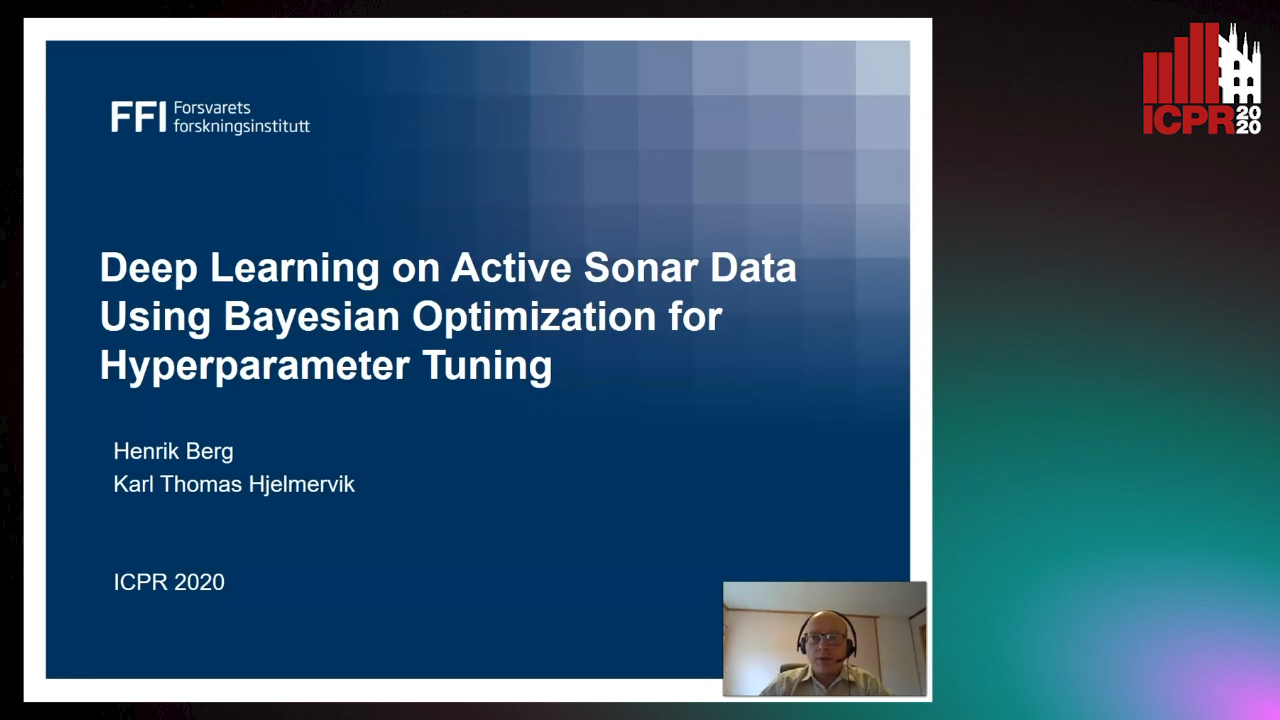
Auto-TLDR; Bayesian Optimization for Sonar Operations in Littoral Environments
Abstract Slides Poster Similar
Proximity Isolation Forests
Antonella Mensi, Manuele Bicego, David Tax
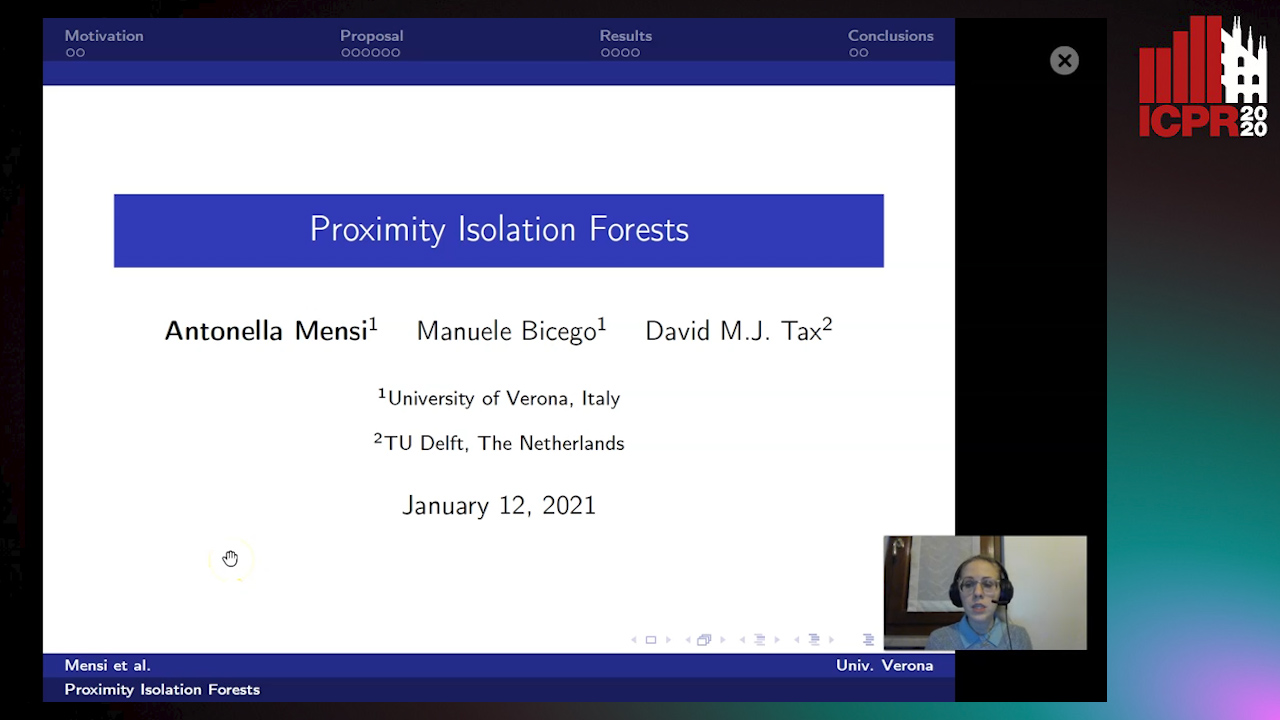
Auto-TLDR; Proximity Isolation Forests for Non-vectorial Data
Abstract Slides Poster Similar
Temporal Pattern Detection in Time-Varying Graphical Models
Federico Tomasi, Veronica Tozzo, Annalisa Barla
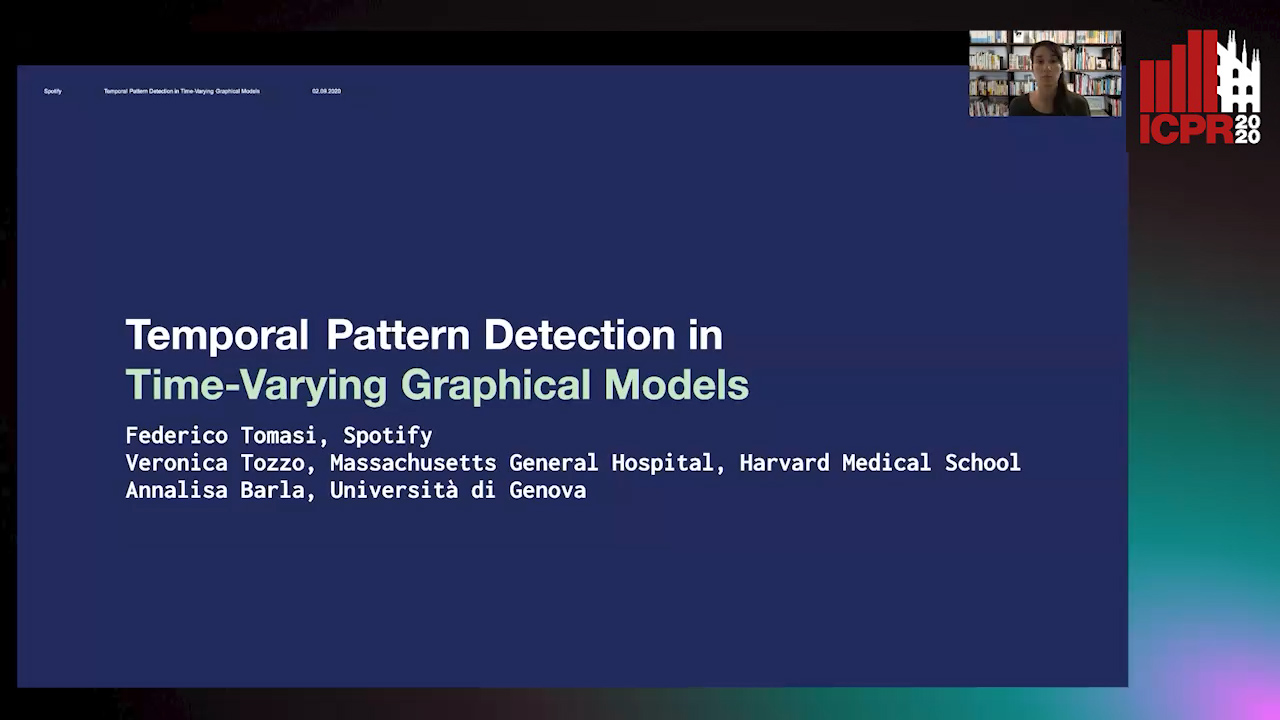
Auto-TLDR; A dynamical network inference model that leverages on kernels to consider general temporal patterns
Abstract Slides Poster Similar