Light3DPose: Real-Time Multi-Person 3D Pose Estimation from Multiple Views
Alessio Elmi,
Davide Mazzini,
Pietro Tortella

Auto-TLDR; 3D Pose Estimation of Multiple People from a Few calibrated Camera Views using Deep Learning
Similar papers
Exploring Severe Occlusion: Multi-Person 3D Pose Estimation with Gated Convolution
Renshu Gu, Gaoang Wang, Jenq-Neng Hwang
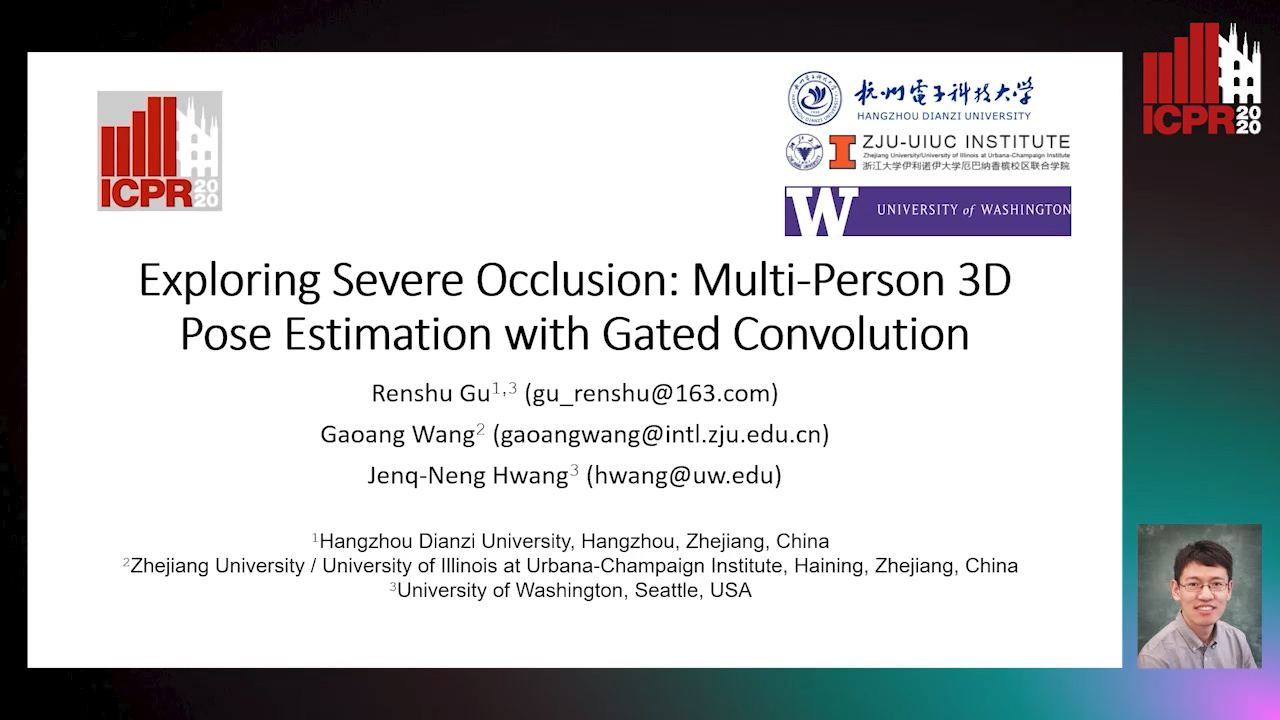
Auto-TLDR; 3D Human Pose Estimation for Multi-Human Videos with Occlusion
StrongPose: Bottom-up and Strong Keypoint Heat Map Based Pose Estimation
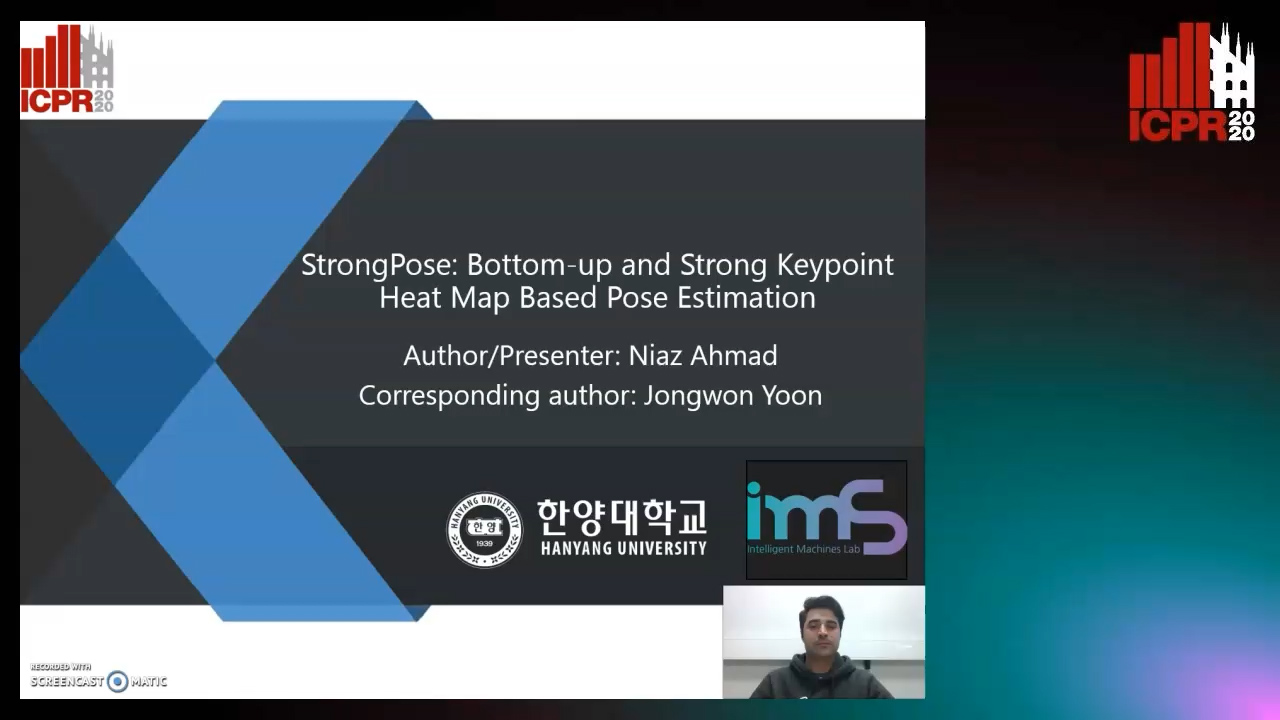
Auto-TLDR; StrongPose: A bottom-up box-free approach for human pose estimation and action recognition
Abstract Slides Poster Similar
RefiNet: 3D Human Pose Refinement with Depth Maps
Andrea D'Eusanio, Stefano Pini, Guido Borghi, Roberto Vezzani, Rita Cucchiara

Auto-TLDR; RefiNet: A Multi-stage Framework for 3D Human Pose Estimation
Simple Multi-Resolution Representation Learning for Human Pose Estimation
Trung Tran Quang, Van Giang Nguyen, Daeyoung Kim
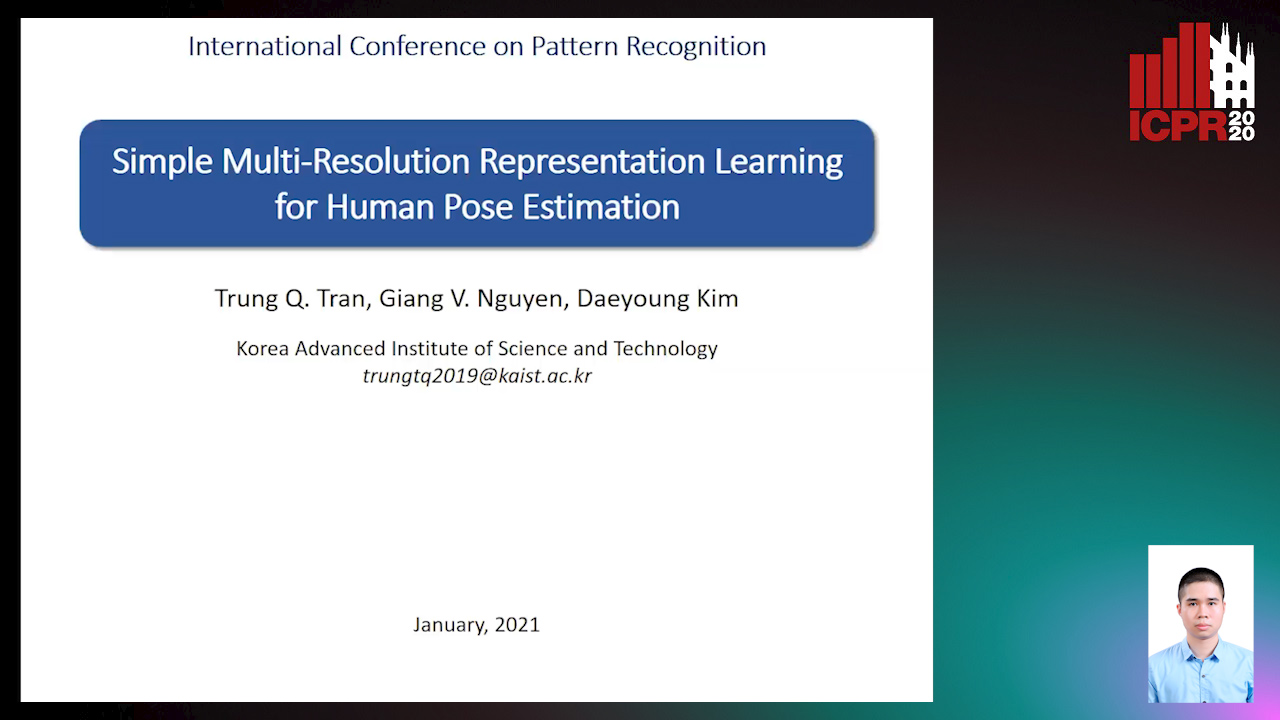
Auto-TLDR; Multi-resolution Heatmap Learning for Human Pose Estimation
Abstract Slides Poster Similar
Orthographic Projection Linear Regression for Single Image 3D Human Pose Estimation
Yahui Zhang, Shaodi You, Theo Gevers
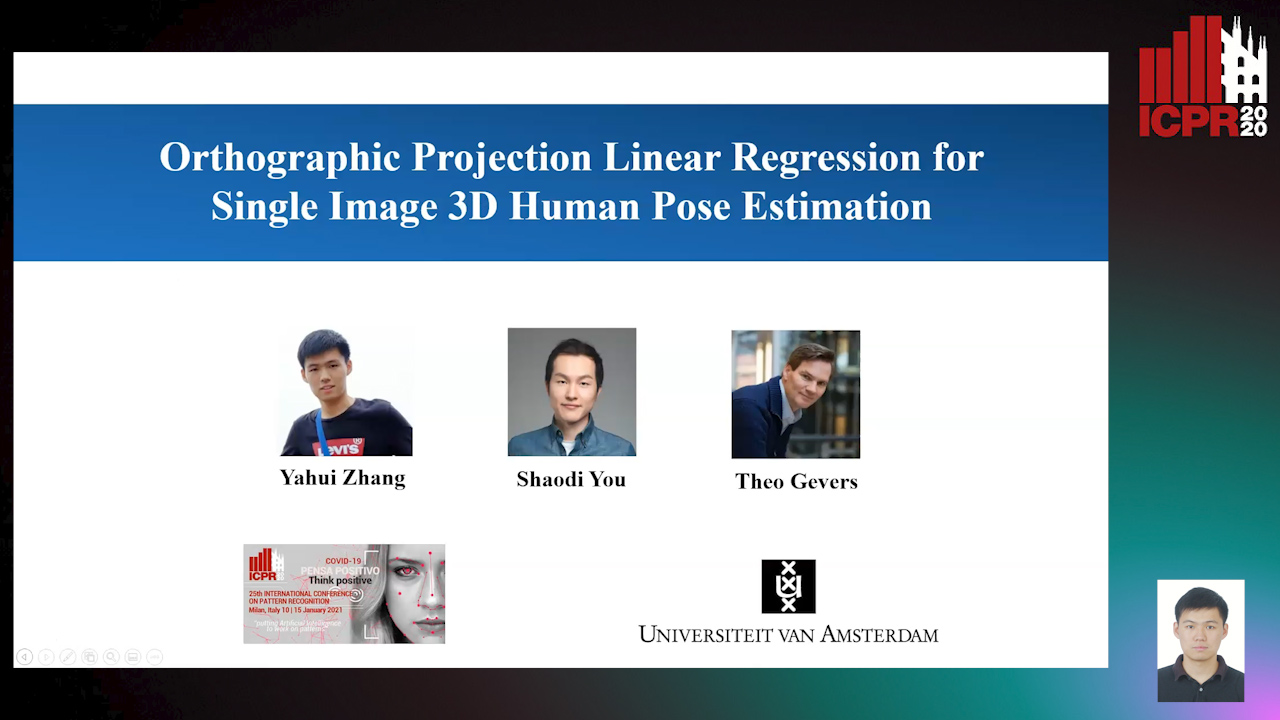
Auto-TLDR; A Deep Neural Network for 3D Human Pose Estimation from a Single 2D Image in the Wild
Abstract Slides Poster Similar
P2 Net: Augmented Parallel-Pyramid Net for Attention Guided Pose Estimation
Luanxuan Hou, Jie Cao, Yuan Zhao, Haifeng Shen, Jian Tang, Ran He
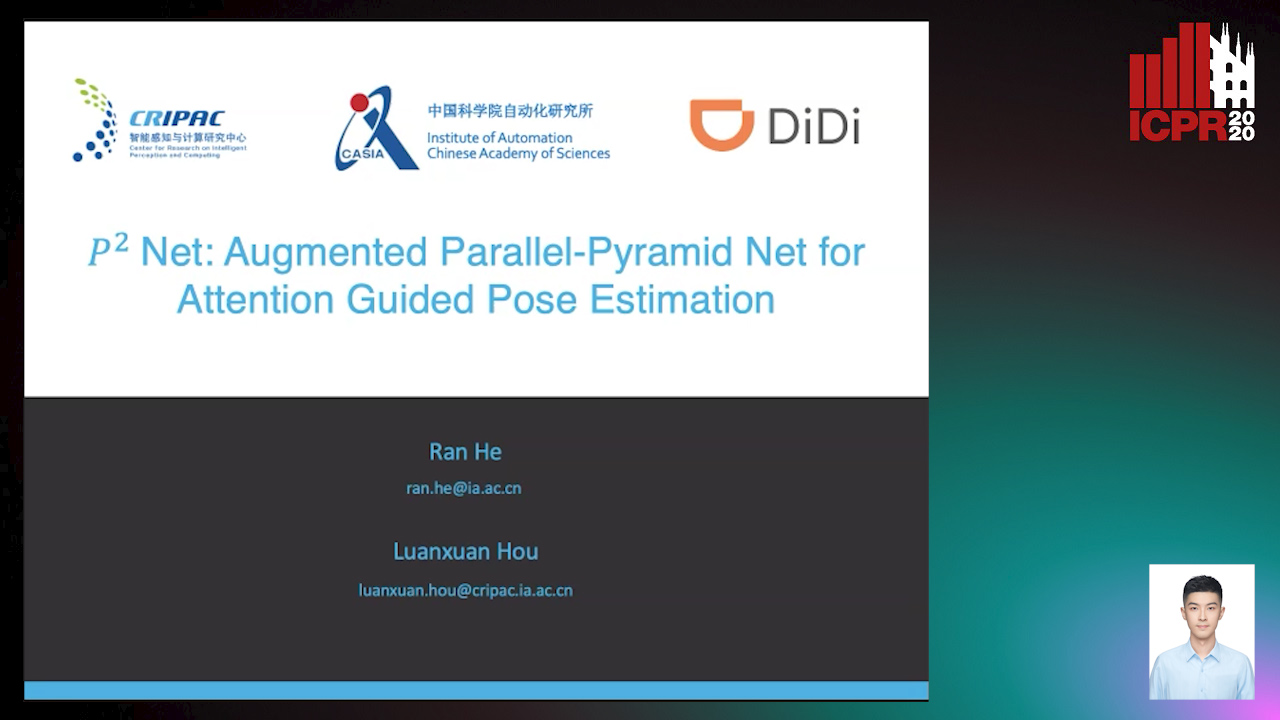
Auto-TLDR; Parallel-Pyramid Net with Partial Attention for Human Pose Estimation
Abstract Slides Poster Similar
HPERL: 3D Human Pose Estimastion from RGB and LiDAR
Michael Fürst, Shriya T.P. Gupta, René Schuster, Oliver Wasenmüler, Didier Stricker
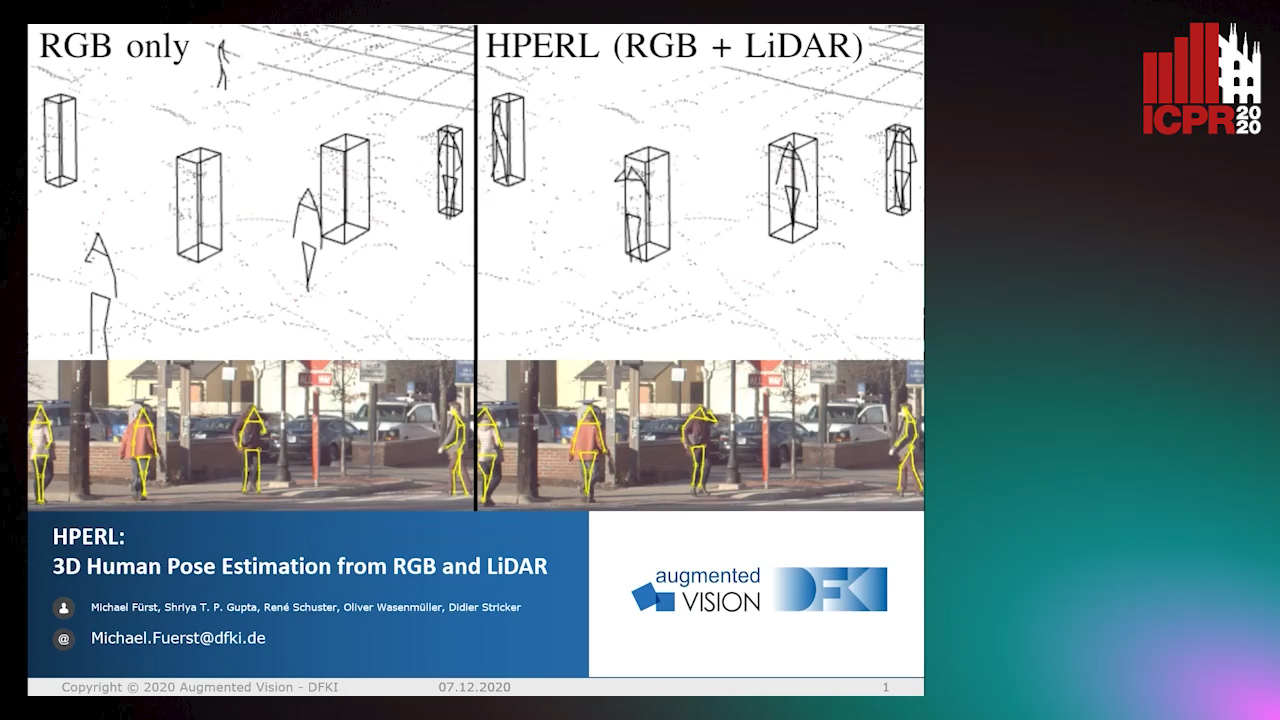
Auto-TLDR; 3D Human Pose Estimation Using RGB and LiDAR Using Weakly-Supervised Approach
Abstract Slides Poster Similar
Unsupervised 3D Human Pose Estimation in Multi-view-multi-pose Video
Cheng Sun, Diego Thomas, Hiroshi Kawasaki
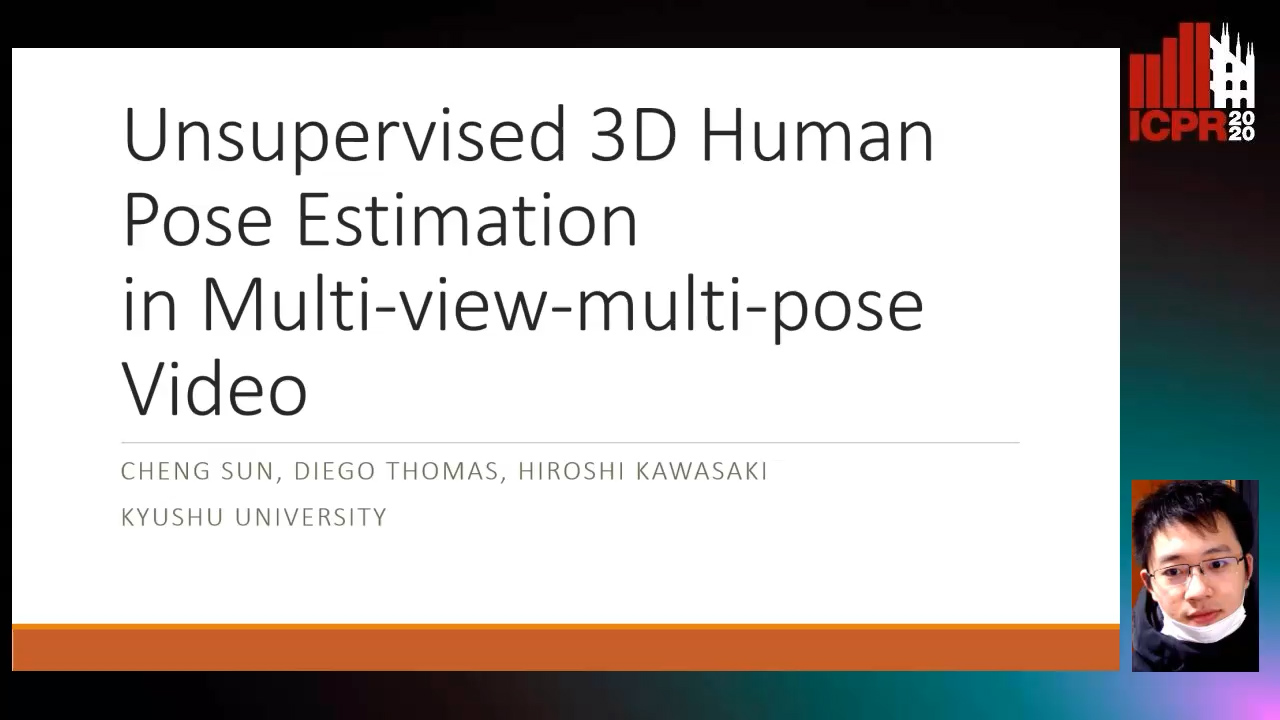
Auto-TLDR; Unsupervised 3D Human Pose Estimation from 2D Videos Using Generative Adversarial Network
Abstract Slides Poster Similar
Occlusion-Tolerant and Personalized 3D Human Pose Estimation in RGB Images
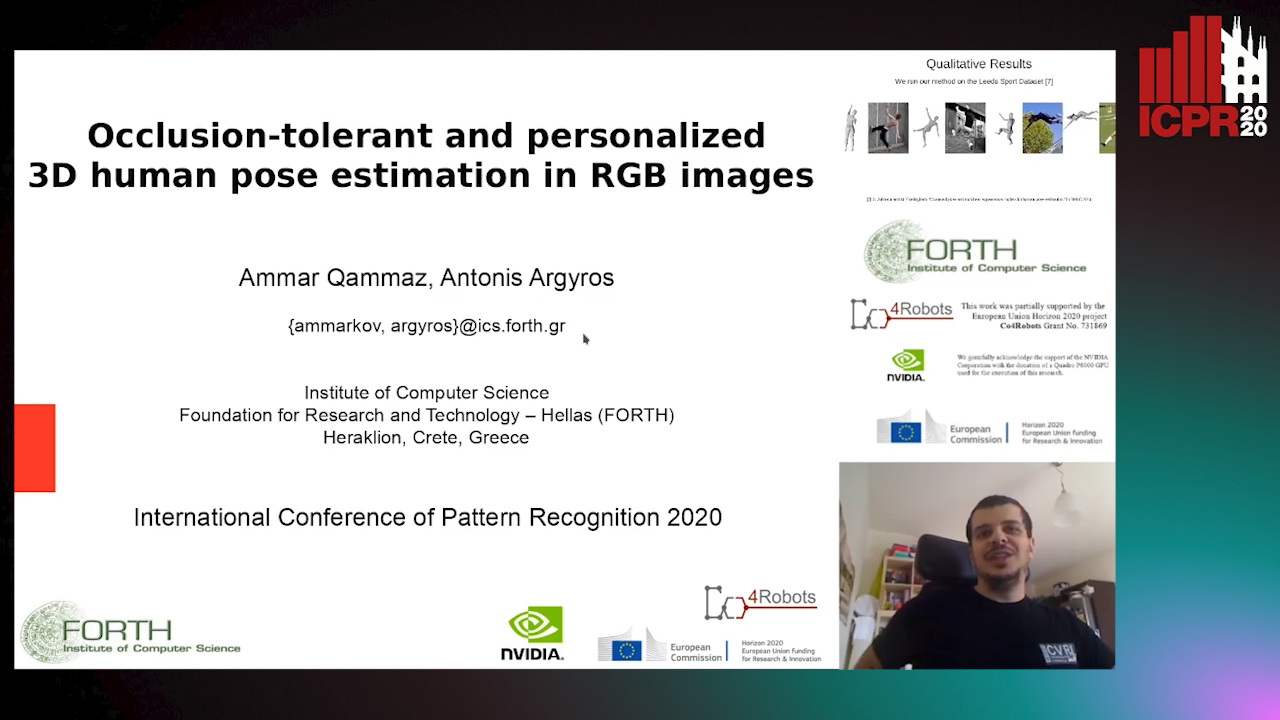
Auto-TLDR; Real-Time 3D Human Pose Estimation in BVH using Inverse Kinematics Solver and Neural Networks
PEAN: 3D Hand Pose Estimation Adversarial Network
Linhui Sun, Yifan Zhang, Jing Lu, Jian Cheng, Hanqing Lu
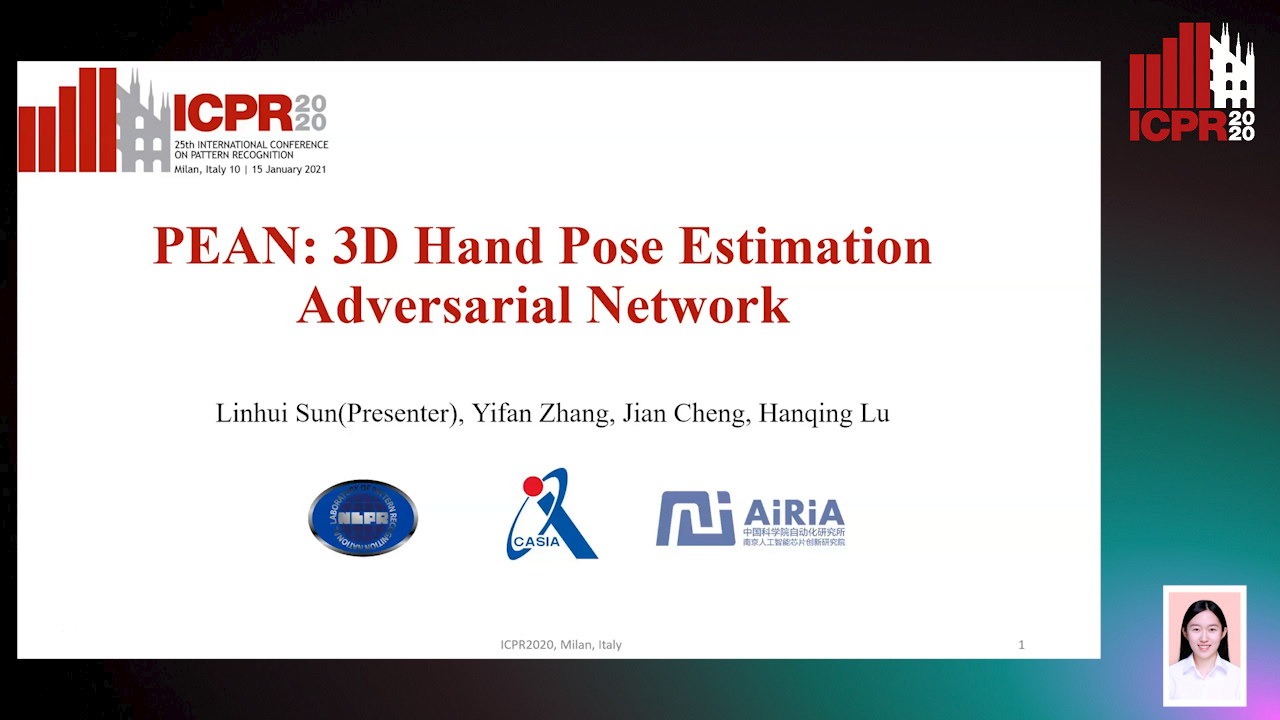
Auto-TLDR; PEAN: 3D Hand Pose Estimation with Adversarial Learning Framework
Abstract Slides Poster Similar
Better Prior Knowledge Improves Human-Pose-Based Extrinsic Camera Calibration
Olivier Moliner, Sangxia Huang, Kalle Åström
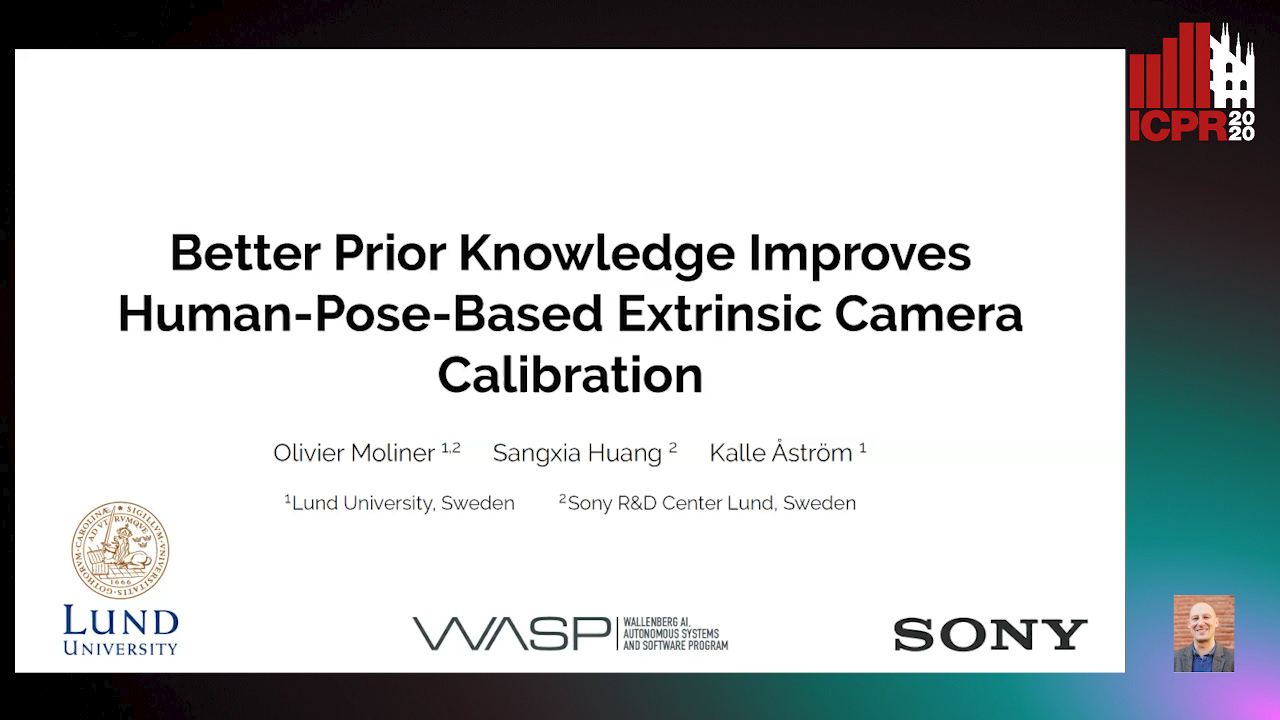
Auto-TLDR; Improving Human-pose-based Extrinsic Calibration for Multi-Camera Systems
Abstract Slides Poster Similar
Tilting at Windmills: Data Augmentation for Deeppose Estimation Does Not Help with Occlusions
Rafal Pytel, Osman Semih Kayhan, Jan Van Gemert
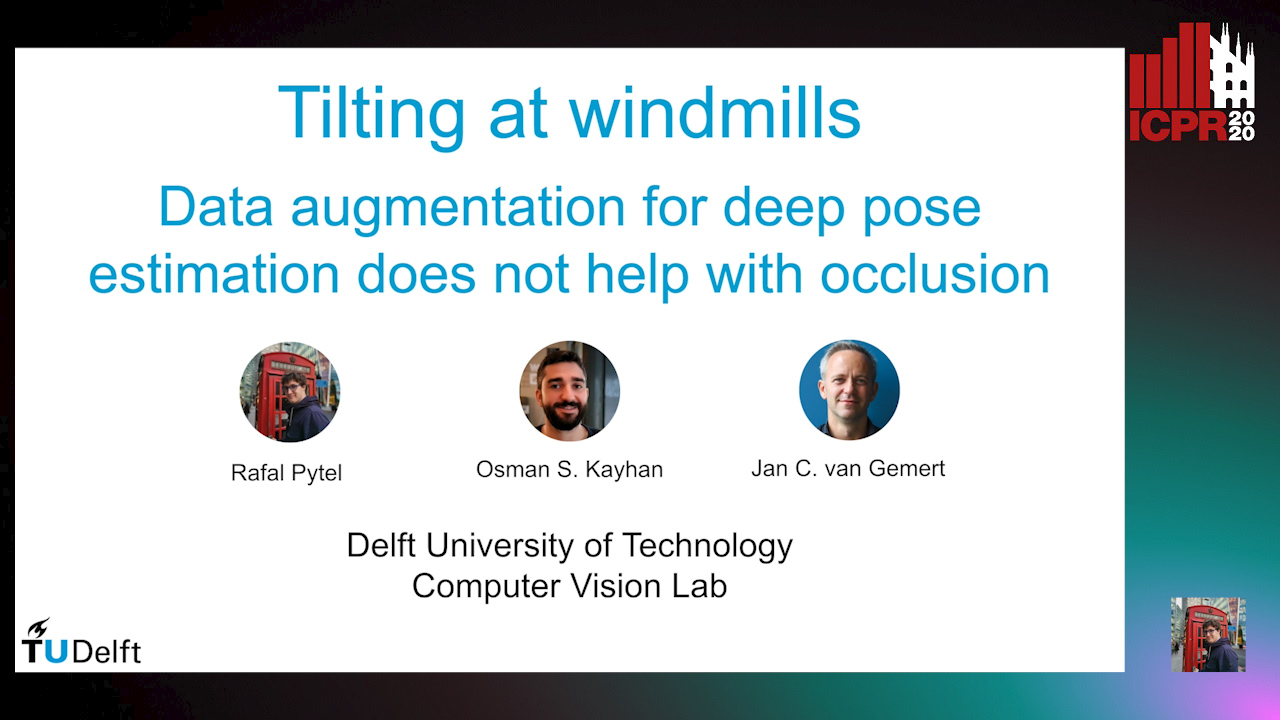
Auto-TLDR; Targeted Keypoint and Body Part Occlusion Attacks for Human Pose Estimation
Abstract Slides Poster Similar
JUMPS: Joints Upsampling Method for Pose Sequences
Lucas Mourot, Francois Le Clerc, Cédric Thébault, Pierre Hellier
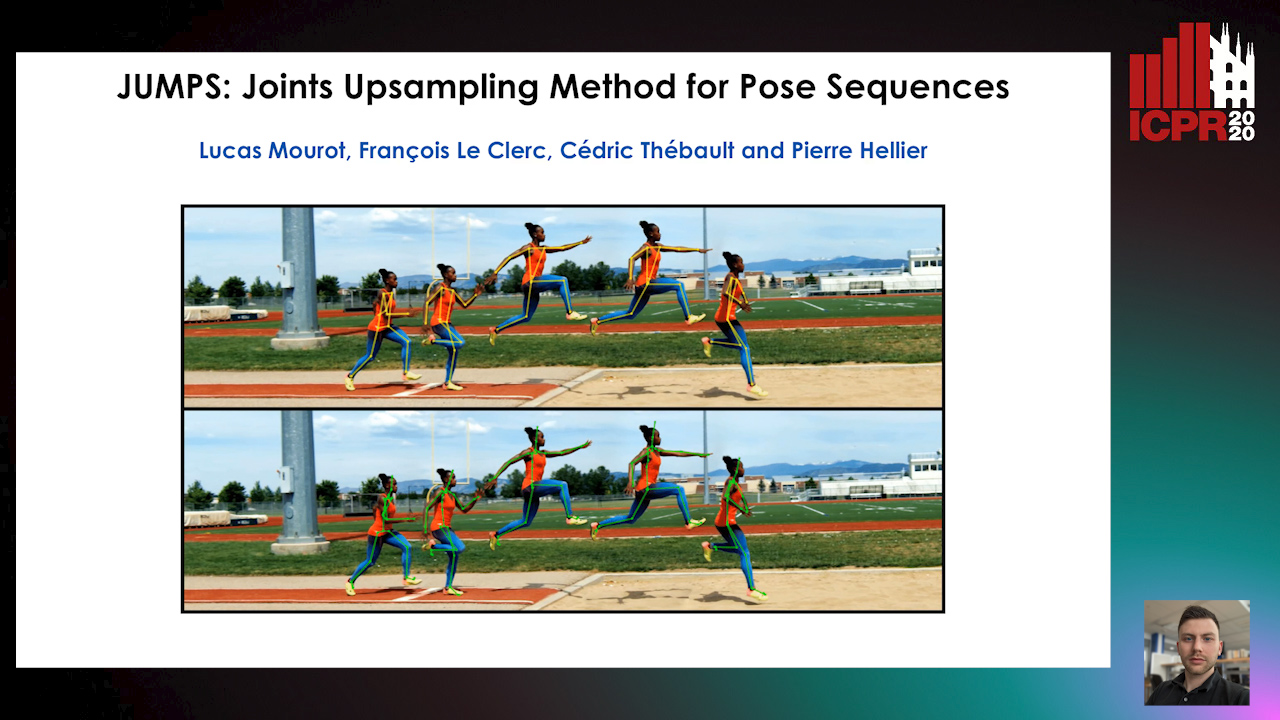
Auto-TLDR; JUMPS: Increasing the Number of Joints in 2D Pose Estimation and Recovering Occluded or Missing Joints
Abstract Slides Poster Similar
A Multi-Task Neural Network for Action Recognition with 3D Key-Points
Rongxiao Tang, Wang Luyang, Zhenhua Guo
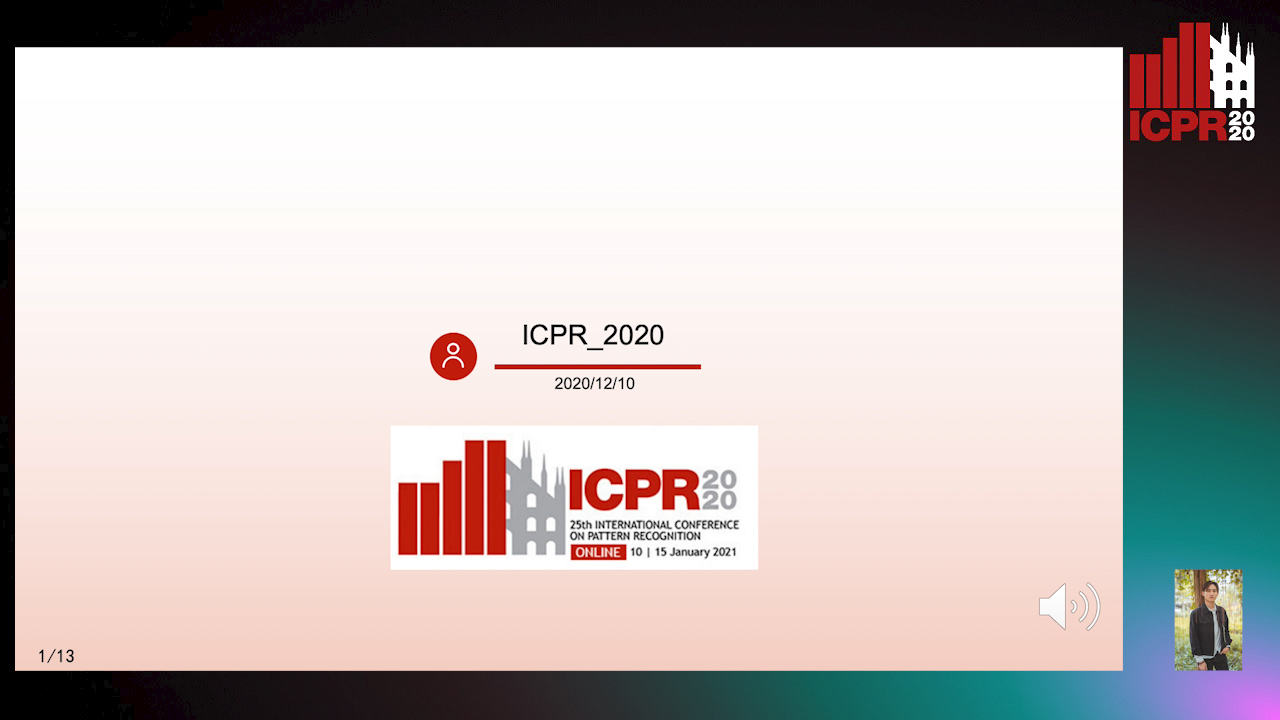
Auto-TLDR; Multi-task Neural Network for Action Recognition and 3D Human Pose Estimation
Abstract Slides Poster Similar
Efficient Grouping for Keypoint Detection
Alexey Sidnev, Ekaterina Krasikova, Maxim Kazakov
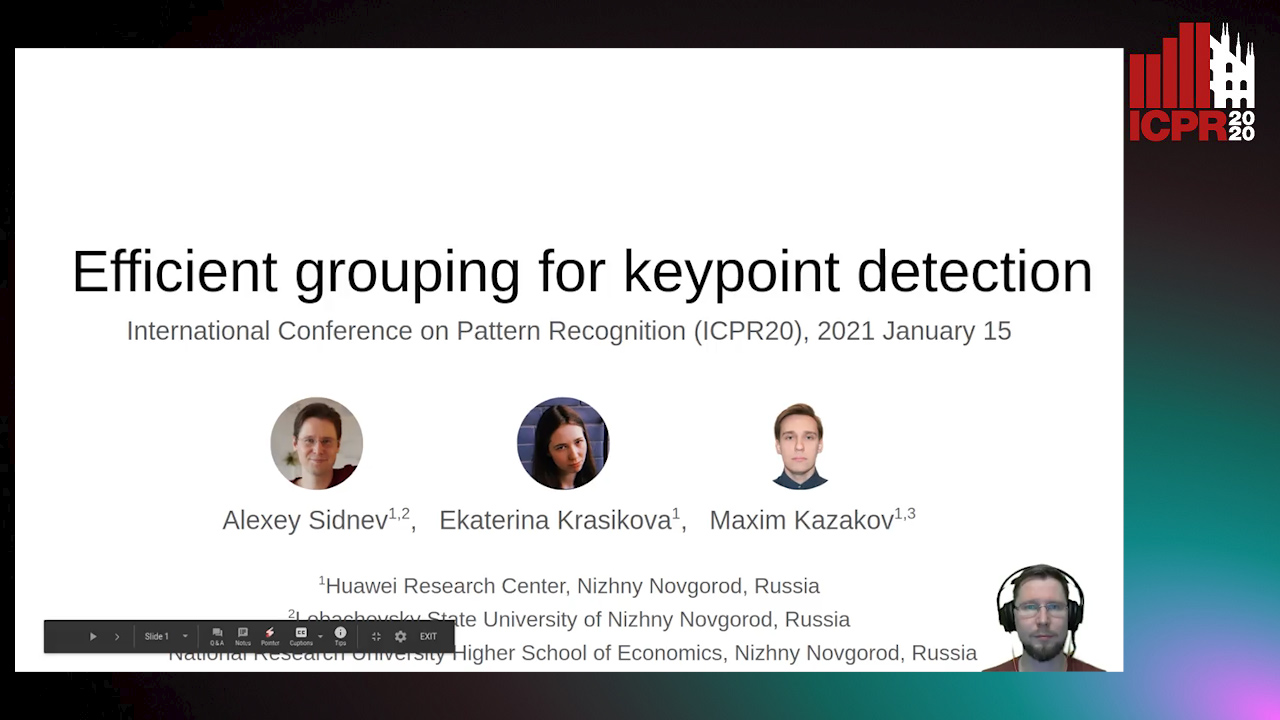
Auto-TLDR; Automatic Keypoint Grouping for DeepFashion2 Dataset
Abstract Slides Poster Similar
Rotational Adjoint Methods for Learning-Free 3D Human Pose Estimation from IMU Data
Caterina Emilia Agelide Buizza, Yiannis Demiris
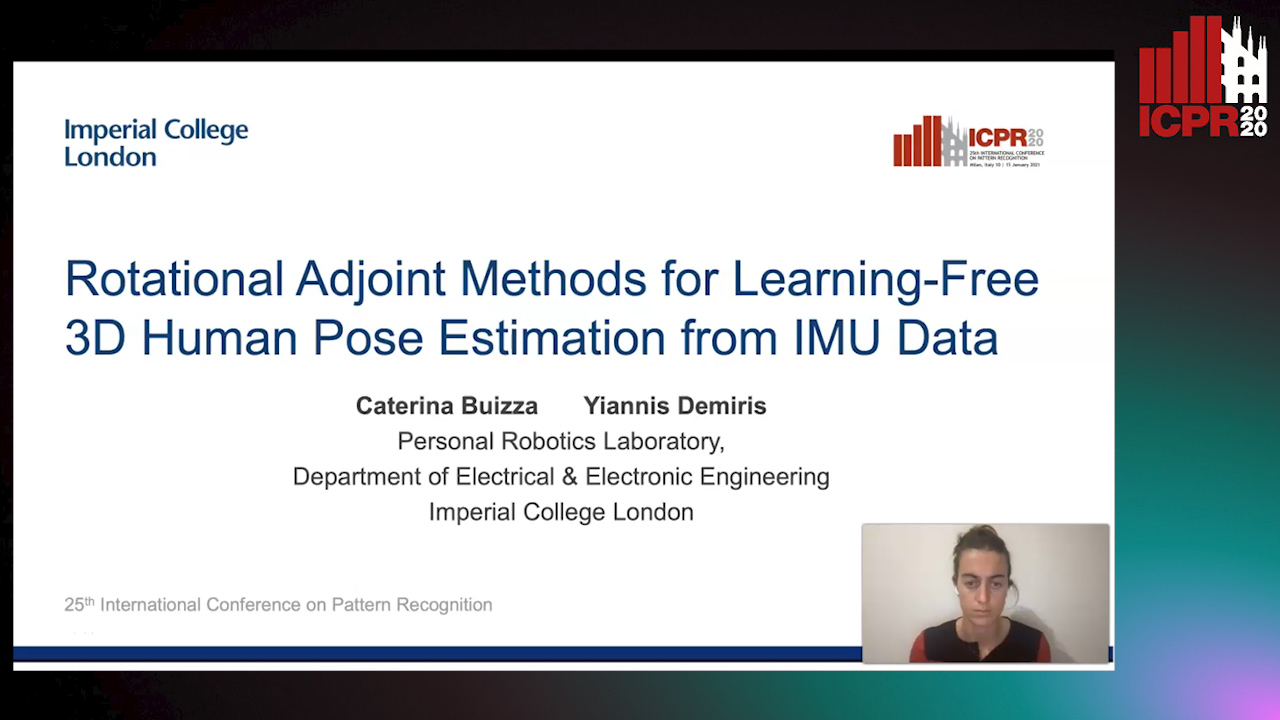
Auto-TLDR; Learning-free 3D Human Pose Estimation from Inertial Measurement Unit Data
Attention-Oriented Action Recognition for Real-Time Human-Robot Interaction
Ziyang Song, Ziyi Yin, Zejian Yuan, Chong Zhang, Wanchao Chi, Yonggen Ling, Shenghao Zhang
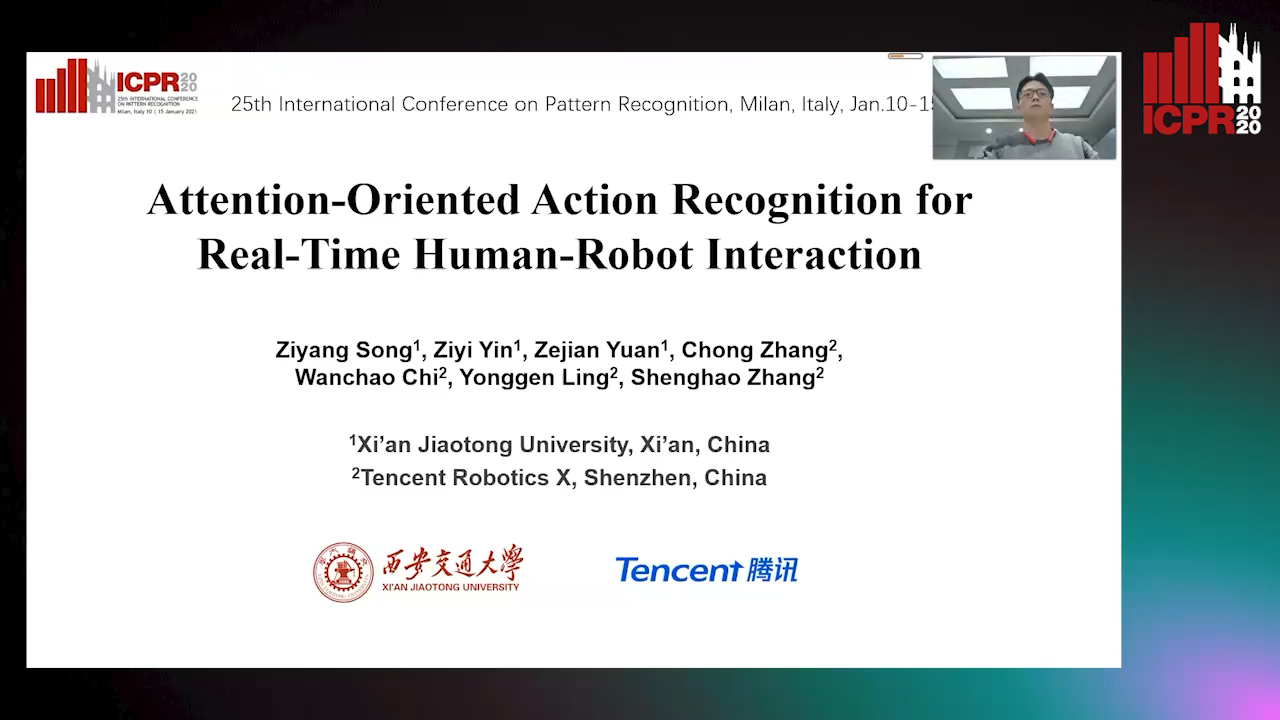
Auto-TLDR; Attention-Oriented Multi-Level Network for Action Recognition in Interaction Scenes
Abstract Slides Poster Similar
What and How? Jointly Forecasting Human Action and Pose
Yanjun Zhu, Yanxia Zhang, Qiong Liu, Andreas Girgensohn
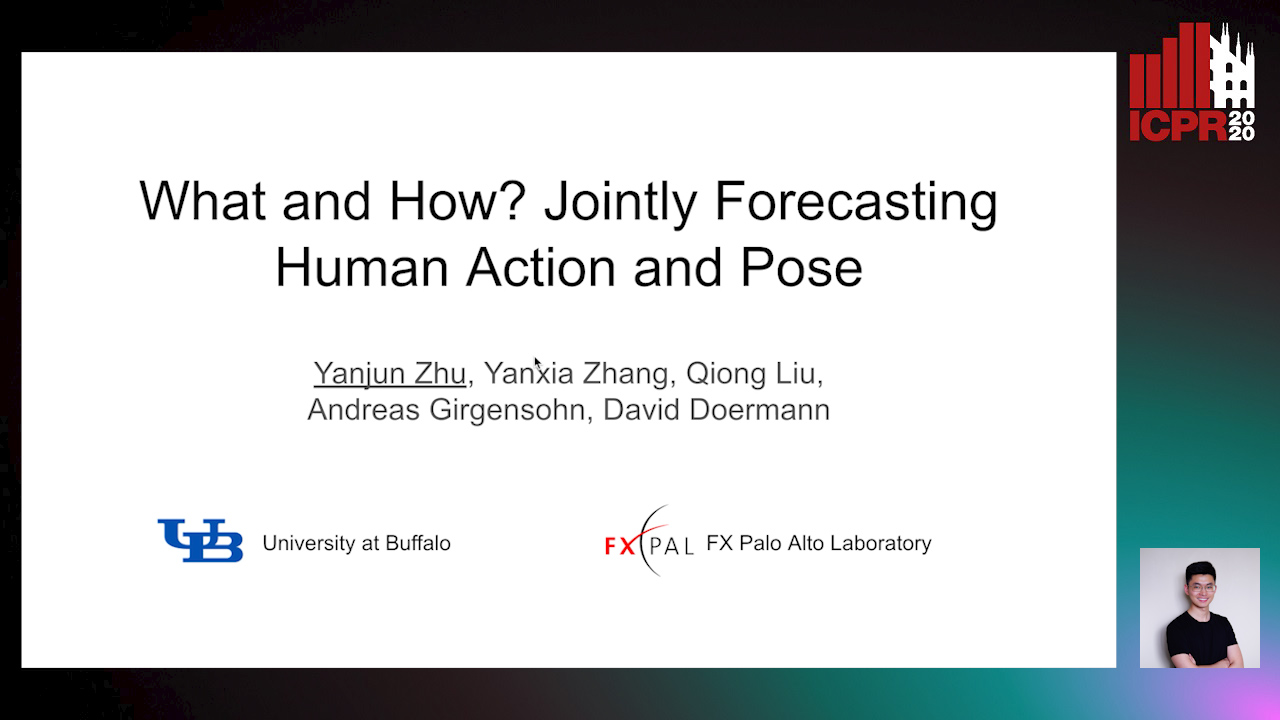
Auto-TLDR; Forecasting Human Actions and Motion Trajectories with Joint Action Classification and Pose Regression
Abstract Slides Poster Similar
Learning Group Activities from Skeletons without Individual Action Labels
Fabio Zappardino, Tiberio Uricchio, Lorenzo Seidenari, Alberto Del Bimbo
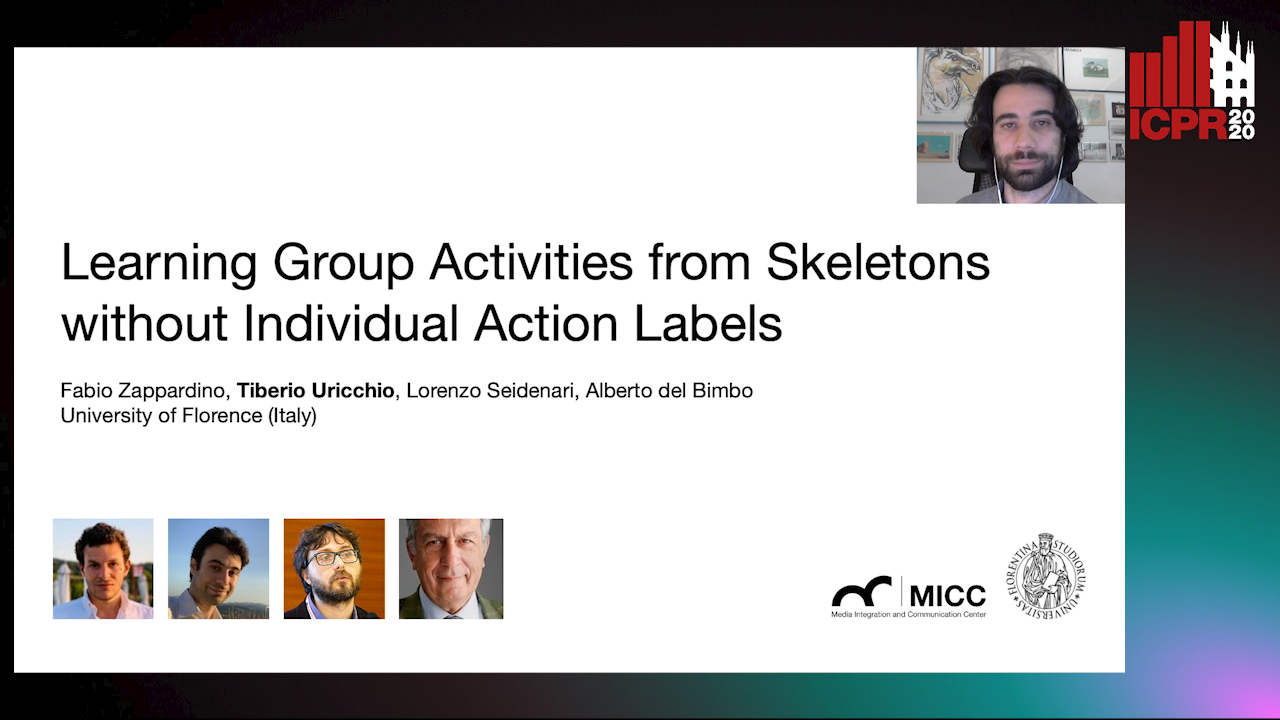
Auto-TLDR; Lean Pose Only for Group Activity Recognition
IPT: A Dataset for Identity Preserved Tracking in Closed Domains
Thomas Heitzinger, Martin Kampel
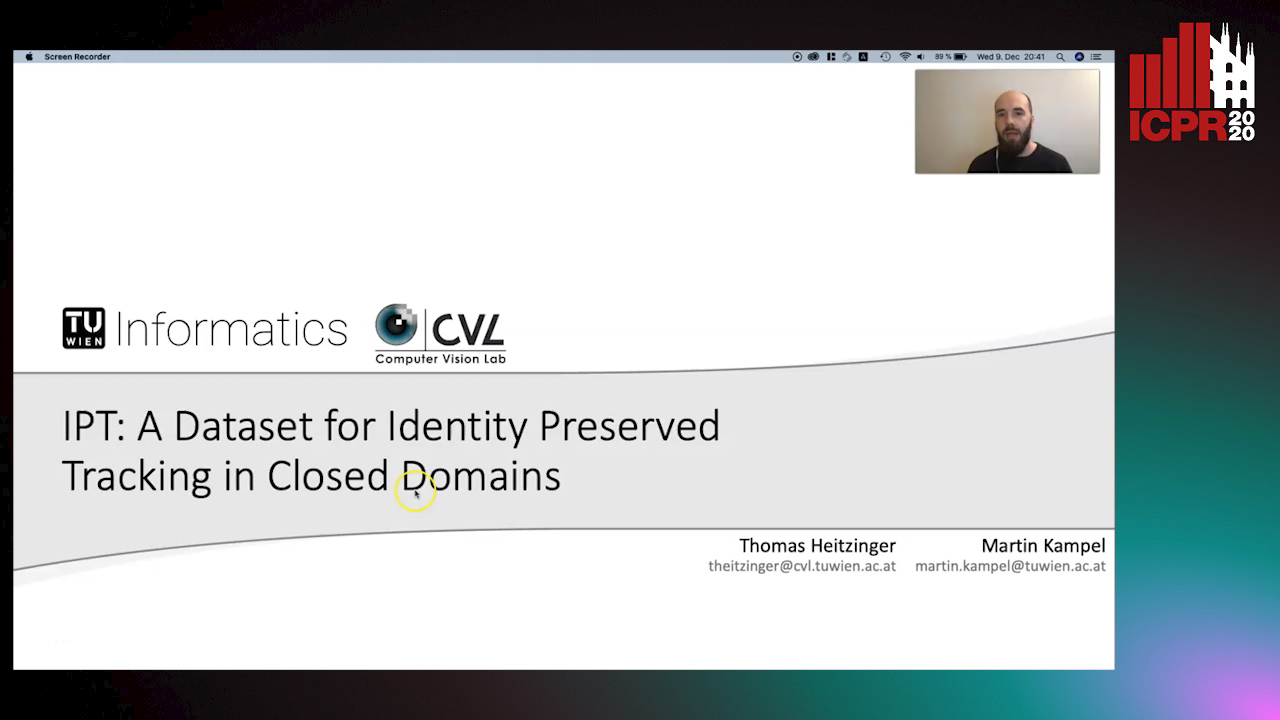
Auto-TLDR; Identity Preserved Tracking Using Depth Data for Privacy and Privacy
Abstract Slides Poster Similar
Learning to Implicitly Represent 3D Human Body from Multi-Scale Features and Multi-View Images
Zhongguo Li, Magnus Oskarsson, Anders Heyden
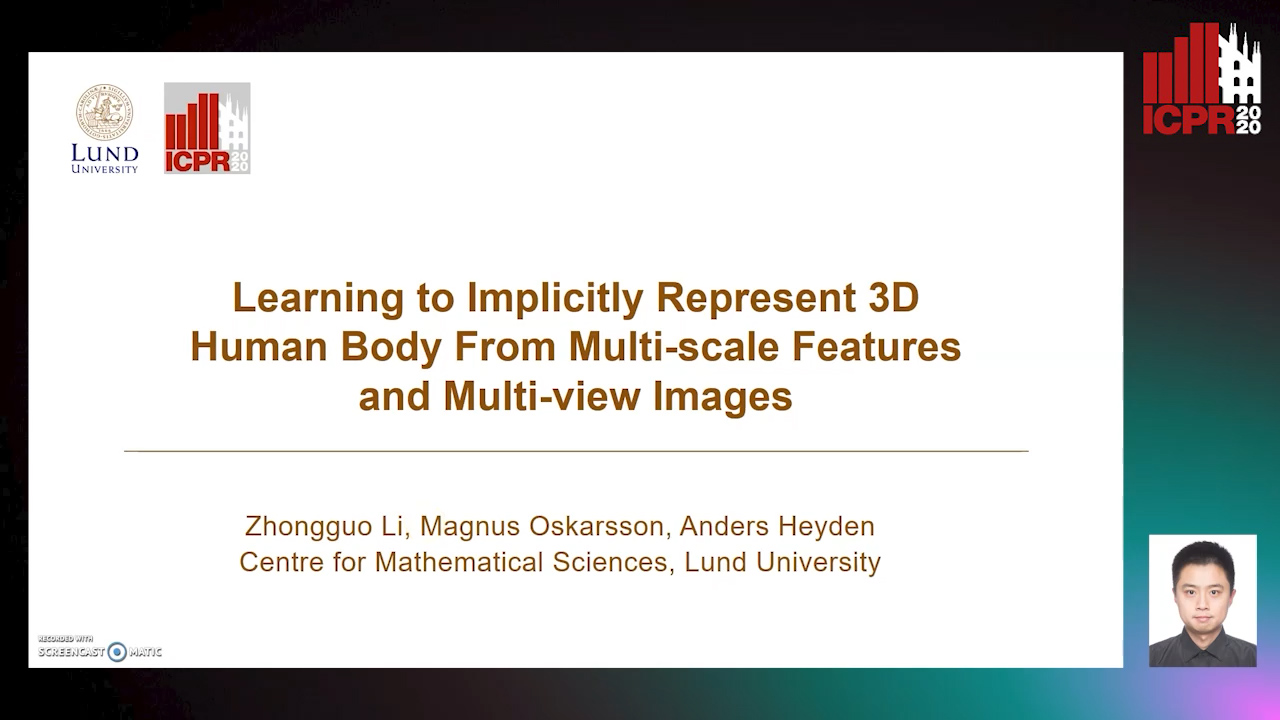
Auto-TLDR; Reconstruction of 3D human bodies from multi-view images using multi-stage end-to-end neural networks
Abstract Slides Poster Similar
A Grid-Based Representation for Human Action Recognition
Soufiane Lamghari, Guillaume-Alexandre Bilodeau, Nicolas Saunier
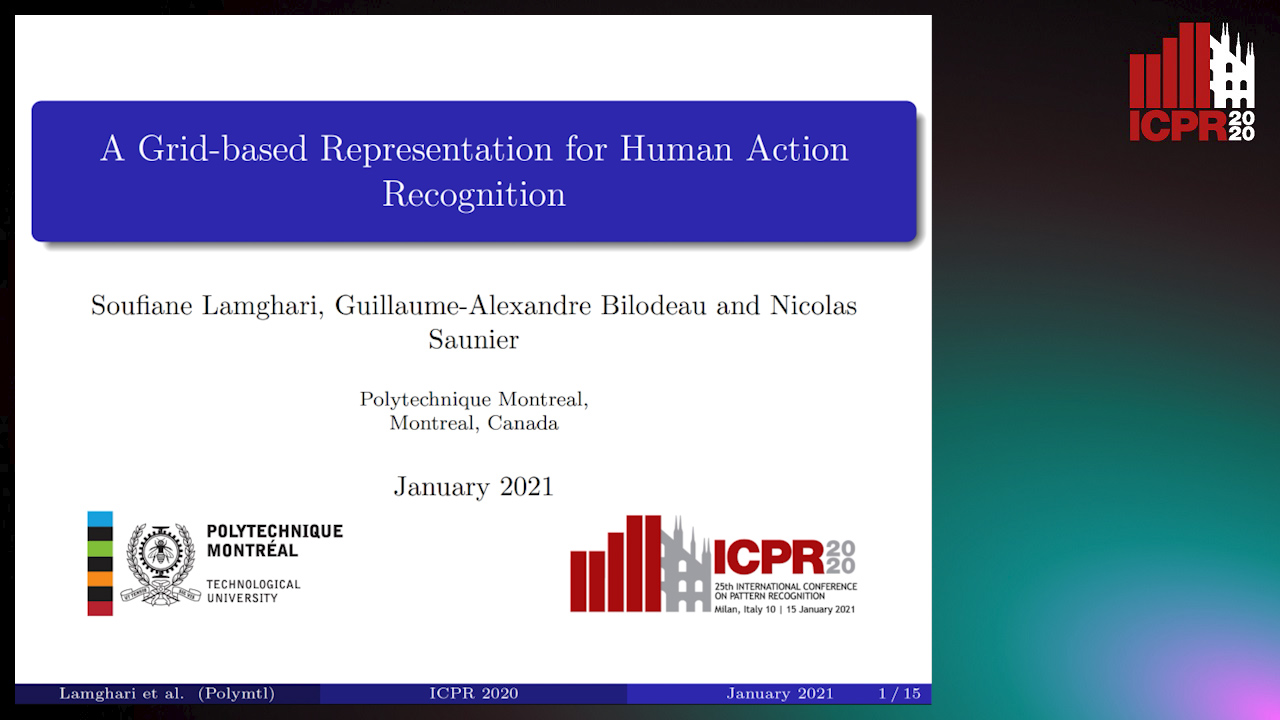
Auto-TLDR; GRAR: Grid-based Representation for Action Recognition in Videos
Abstract Slides Poster Similar
Shape Consistent 2D Keypoint Estimation under Domain Shift
Levi Vasconcelos, Massimiliano Mancini, Davide Boscaini, Barbara Caputo, Elisa Ricci
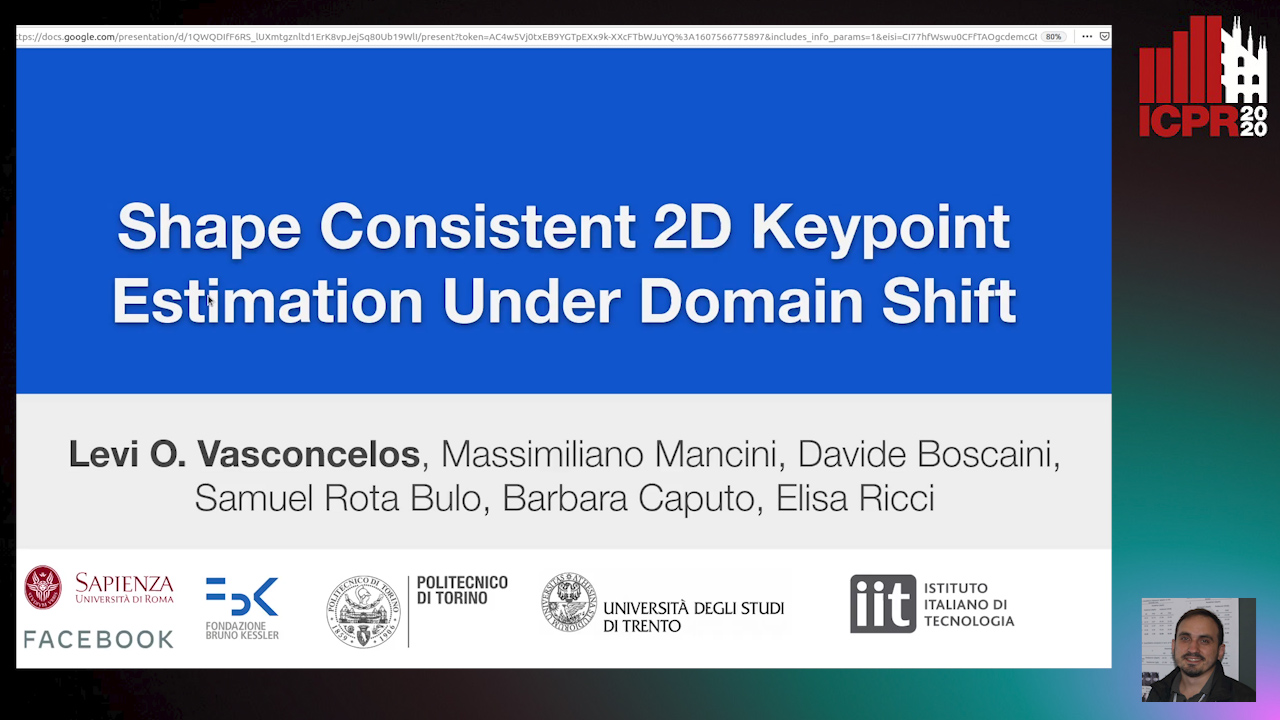
Auto-TLDR; Deep Adaptation for Keypoint Prediction under Domain Shift
Abstract Slides Poster Similar
On the Robustness of 3D Human Pose Estimation
Zerui Chen, Yan Huang, Liang Wang
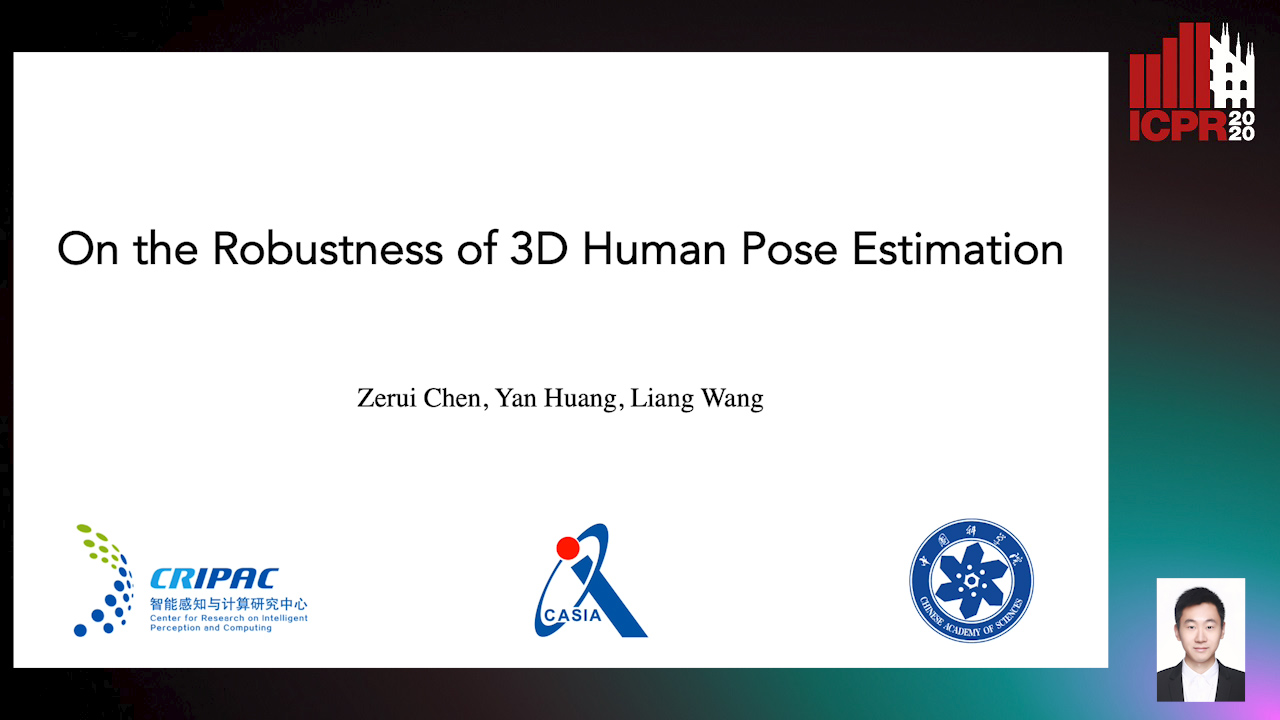
Auto-TLDR; Robustness of 3D Human Pose Estimation Methods to Adversarial Attacks
Efficient High-Resolution High-Level-Semantic Representation Learning for Human Pose Estimation
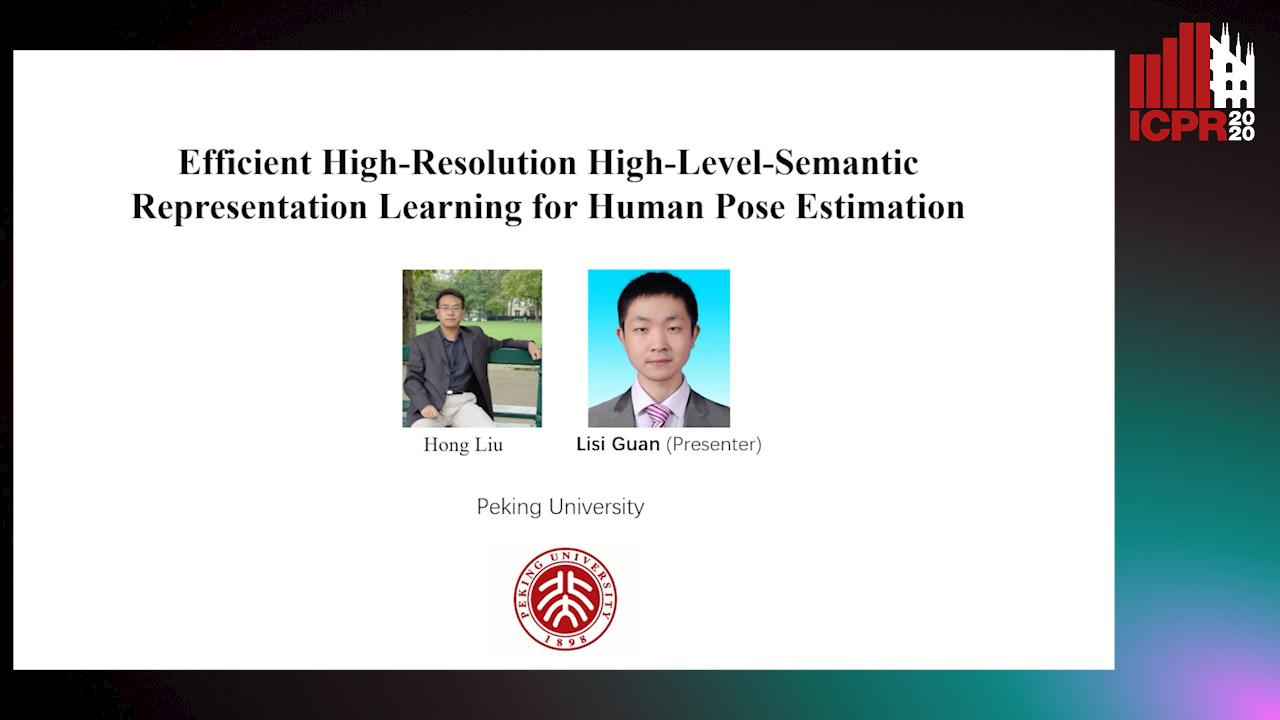
Auto-TLDR; Spatial enhanced separated temporal spatial convolutional neural network
Abstract Slides Poster Similar
Future Urban Scenes Generation through Vehicles Synthesis
Alessandro Simoni, Luca Bergamini, Andrea Palazzi, Simone Calderara, Rita Cucchiara
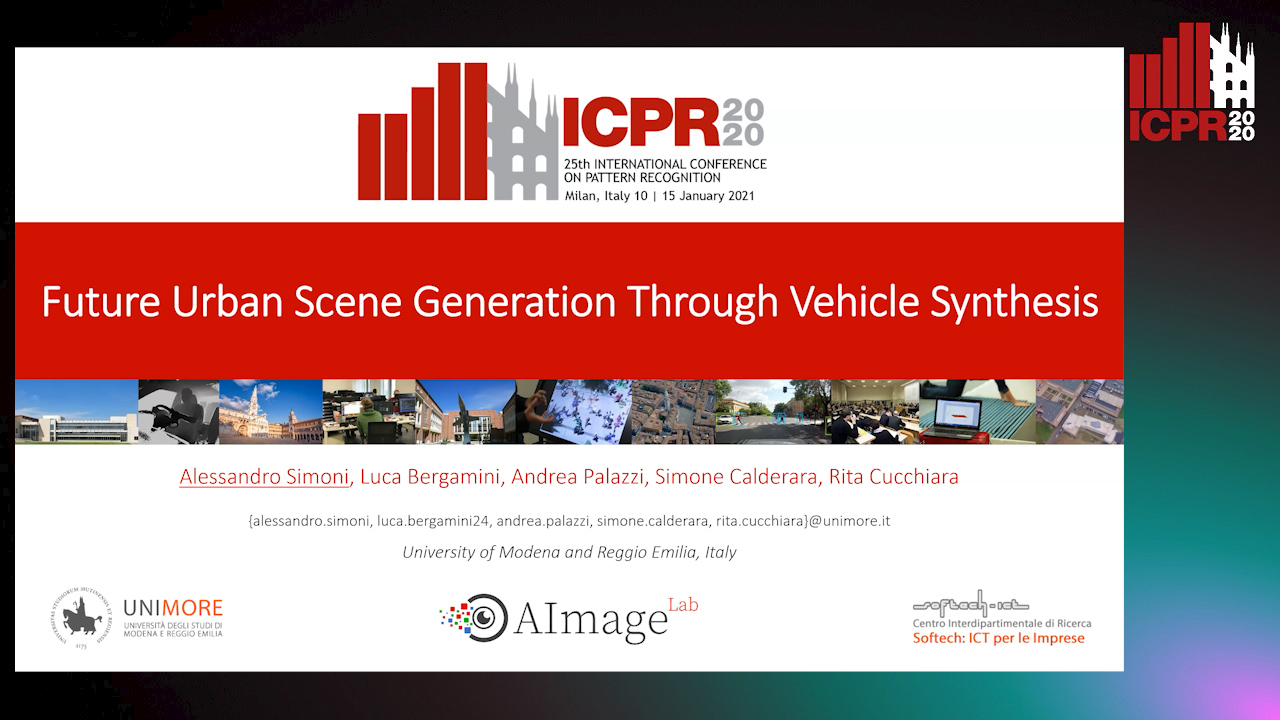
Auto-TLDR; Predicting the Future of an Urban Scene with a Novel View Synthesis Paradigm
Abstract Slides Poster Similar
Hybrid Approach for 3D Head Reconstruction: Using Neural Networks and Visual Geometry
Oussema Bouafif, Bogdan Khomutenko, Mohammed Daoudi
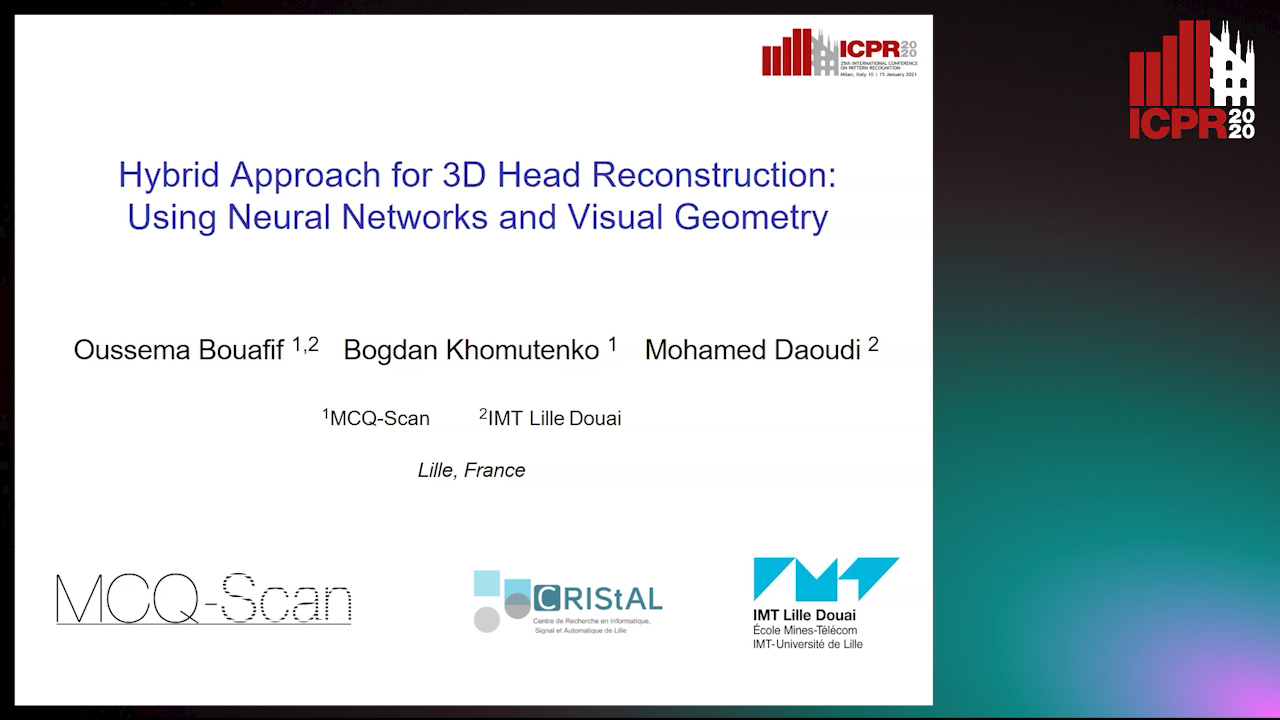
Auto-TLDR; Recovering 3D Head Geometry from a Single Image using Deep Learning and Geometric Techniques
Abstract Slides Poster Similar
Real-Time Monocular Depth Estimation with Extremely Light-Weight Neural Network
Mian Jhong Chiu, Wei-Chen Chiu, Hua-Tsung Chen, Jen-Hui Chuang
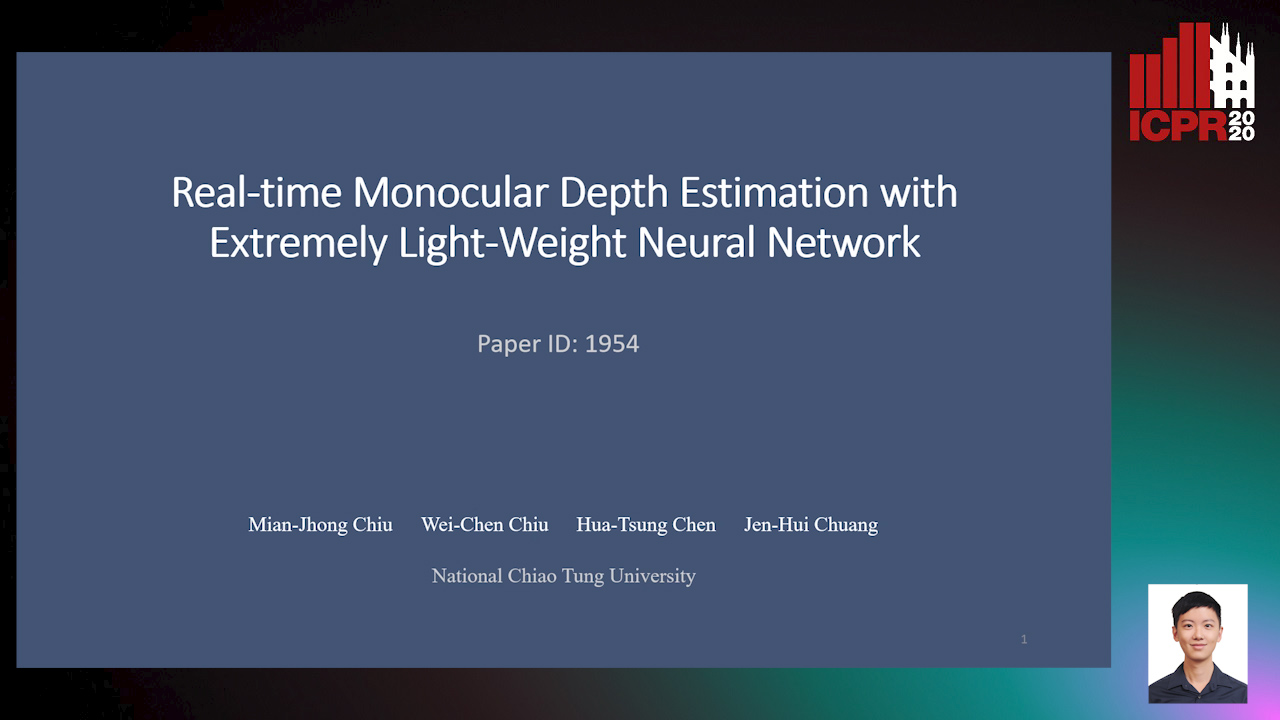
Auto-TLDR; Real-Time Light-Weight Depth Prediction for Obstacle Avoidance and Environment Sensing with Deep Learning-based CNN
Abstract Slides Poster Similar
Extending Single Beam Lidar to Full Resolution by Fusing with Single Image Depth Estimation
Yawen Lu, Yuxing Wang, Devarth Parikh, Guoyu Lu
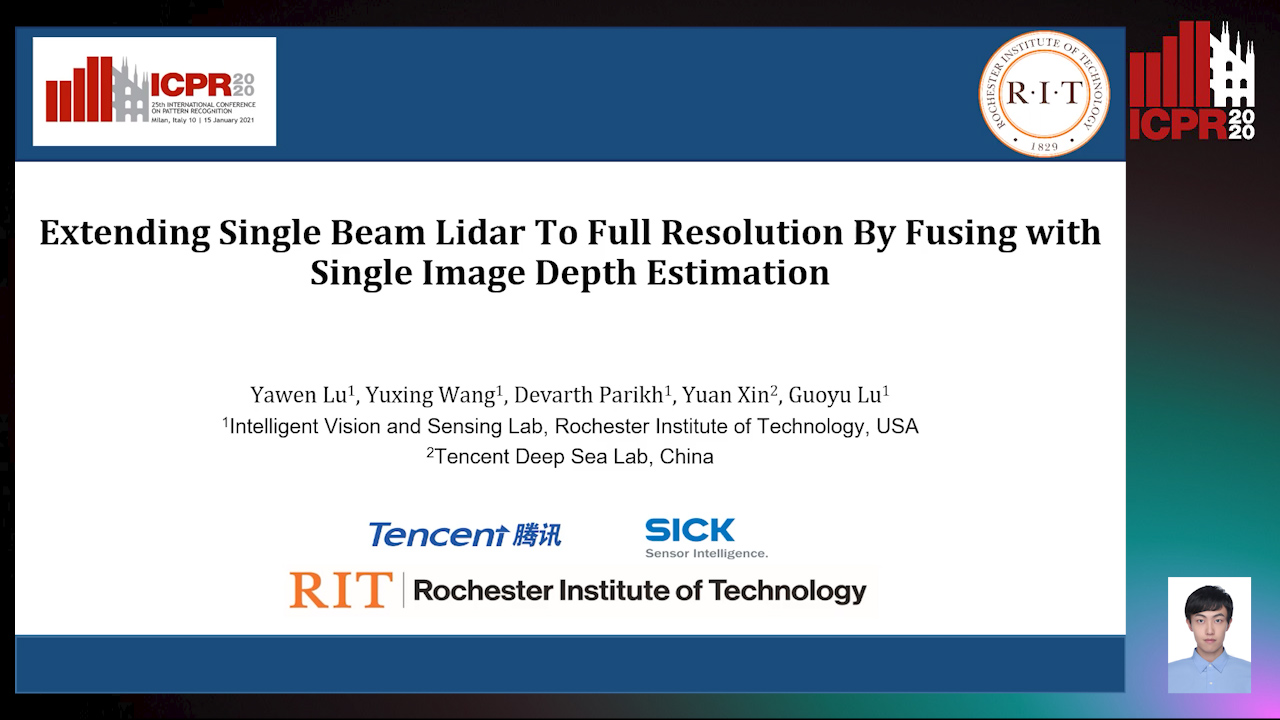
Auto-TLDR; Self-supervised LIDAR for Low-Cost Depth Estimation
P2D: A Self-Supervised Method for Depth Estimation from Polarimetry
Marc Blanchon, Desire Sidibe, Olivier Morel, Ralph Seulin, Daniel Braun, Fabrice Meriaudeau
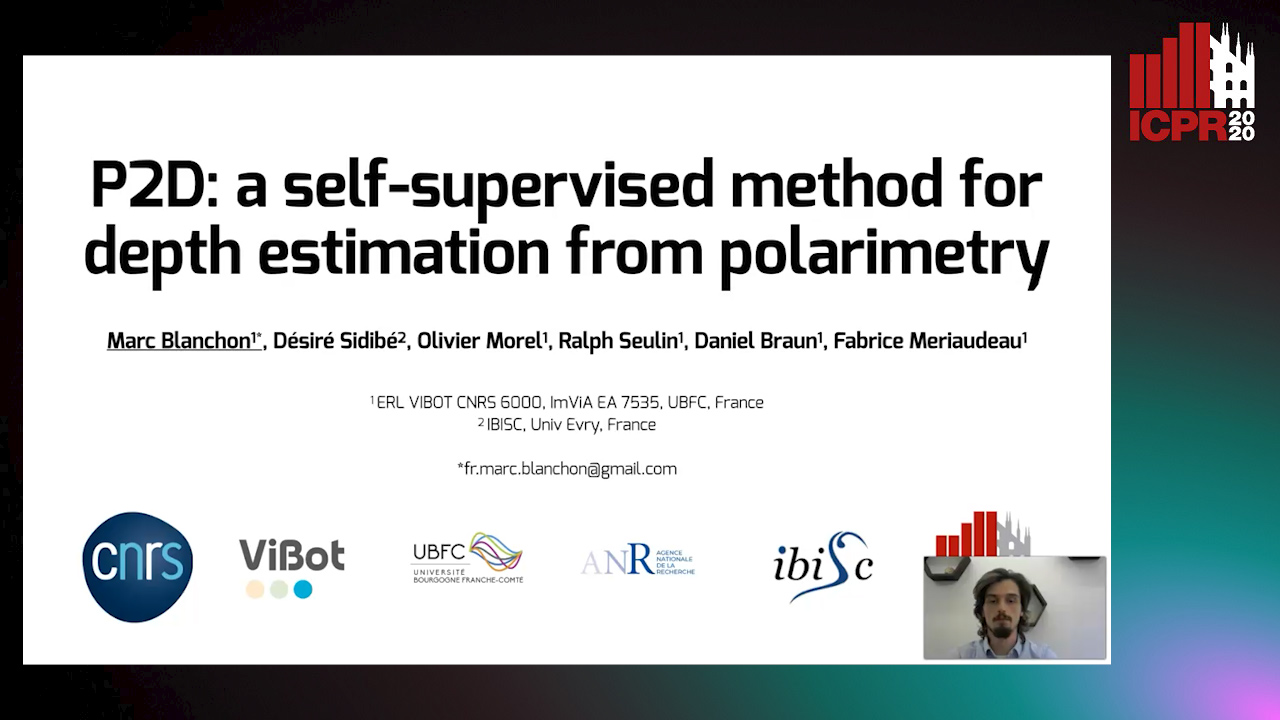
Auto-TLDR; Polarimetric Regularization for Monocular Depth Estimation
Abstract Slides Poster Similar
6D Pose Estimation with Correlation Fusion
Yi Cheng, Hongyuan Zhu, Ying Sun, Cihan Acar, Wei Jing, Yan Wu, Liyuan Li, Cheston Tan, Joo-Hwee Lim
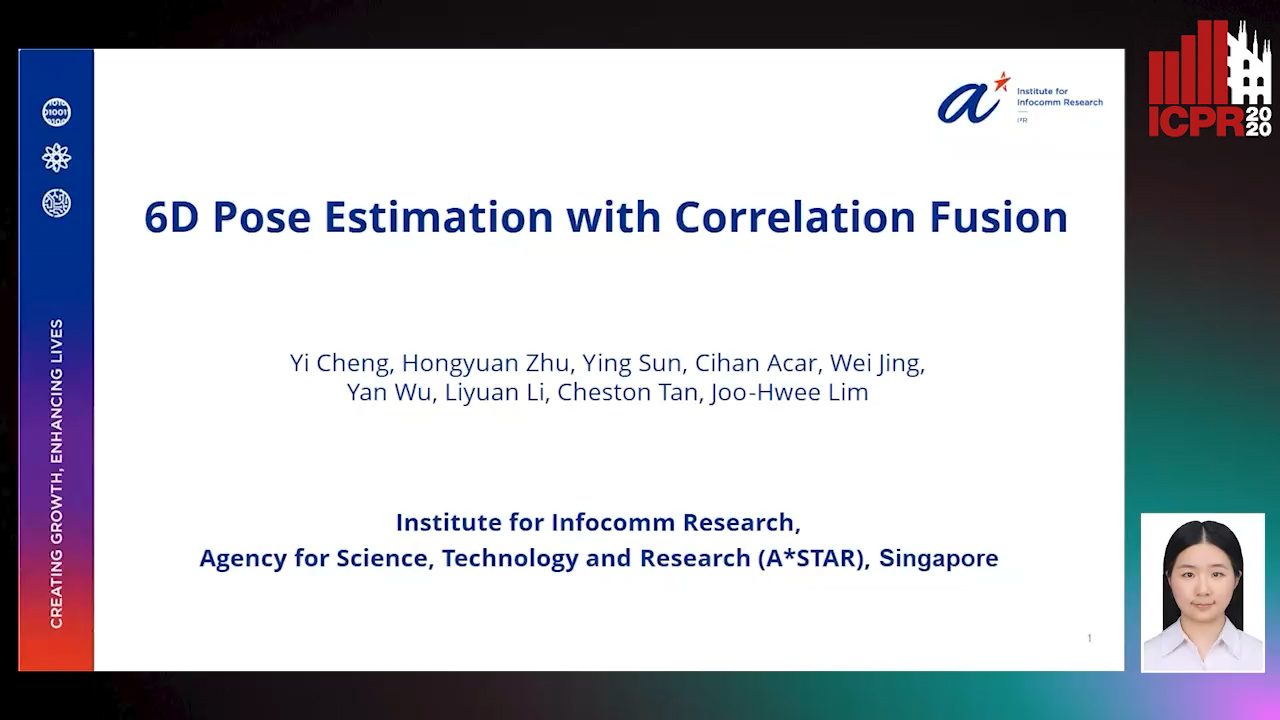
Auto-TLDR; Intra- and Inter-modality Fusion for 6D Object Pose Estimation with Attention Mechanism
Abstract Slides Poster Similar
DeepPear: Deep Pose Estimation and Action Recognition
Wen-Jiin Tsai, You-Ying Jhuang
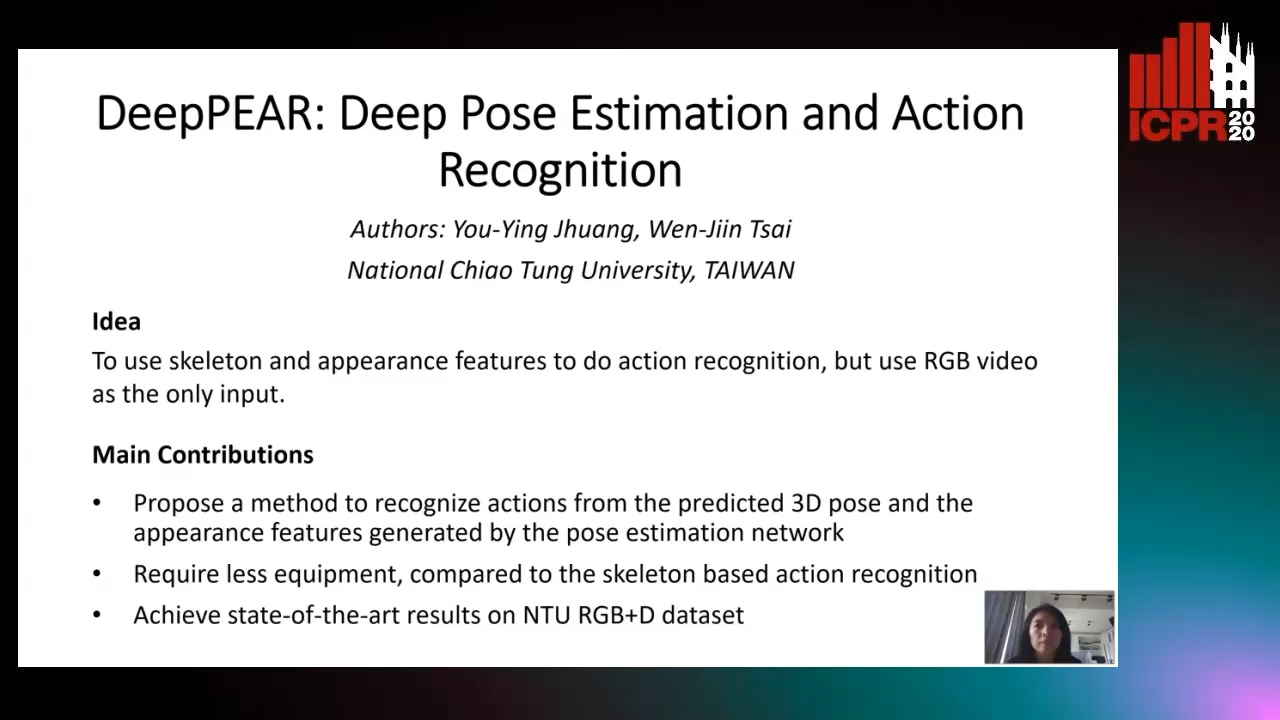
Auto-TLDR; Human Action Recognition Using RGB Video Using 3D Human Pose and Appearance Features
Abstract Slides Poster Similar
Temporal Attention-Augmented Graph Convolutional Network for Efficient Skeleton-Based Human Action Recognition
Negar Heidari, Alexandros Iosifidis
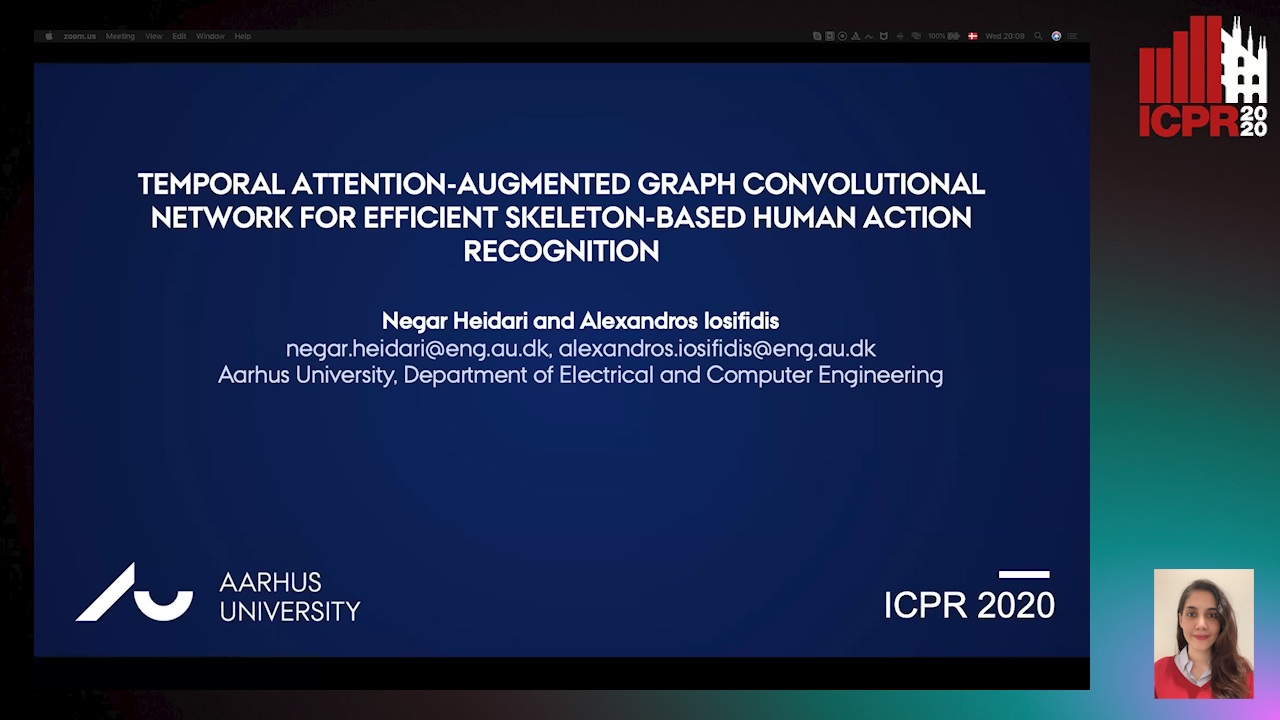
Auto-TLDR; Temporal Attention Module for Efficient Graph Convolutional Network-based Action Recognition
Abstract Slides Poster Similar
MixedFusion: 6D Object Pose Estimation from Decoupled RGB-Depth Features
Hangtao Feng, Lu Zhang, Xu Yang, Zhiyong Liu
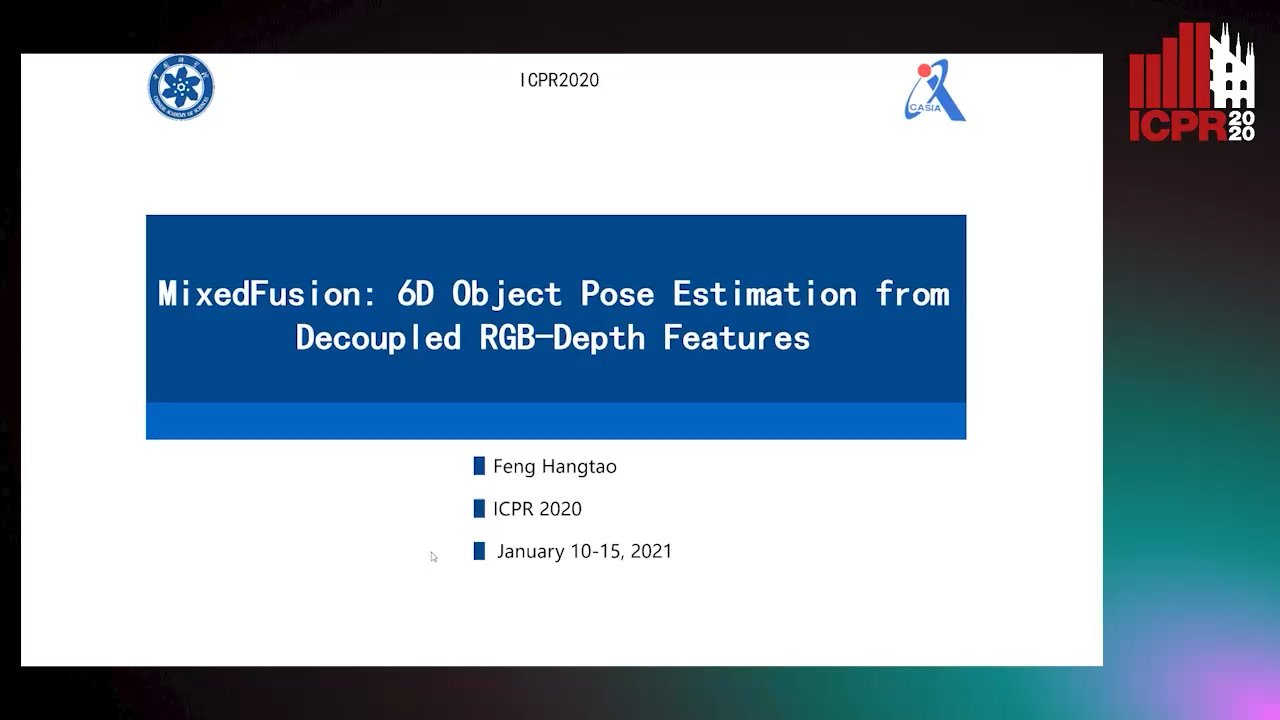
Auto-TLDR; MixedFusion: Combining Color and Point Clouds for 6D Pose Estimation
Abstract Slides Poster Similar
Self-Supervised Joint Encoding of Motion and Appearance for First Person Action Recognition
Mirco Planamente, Andrea Bottino, Barbara Caputo
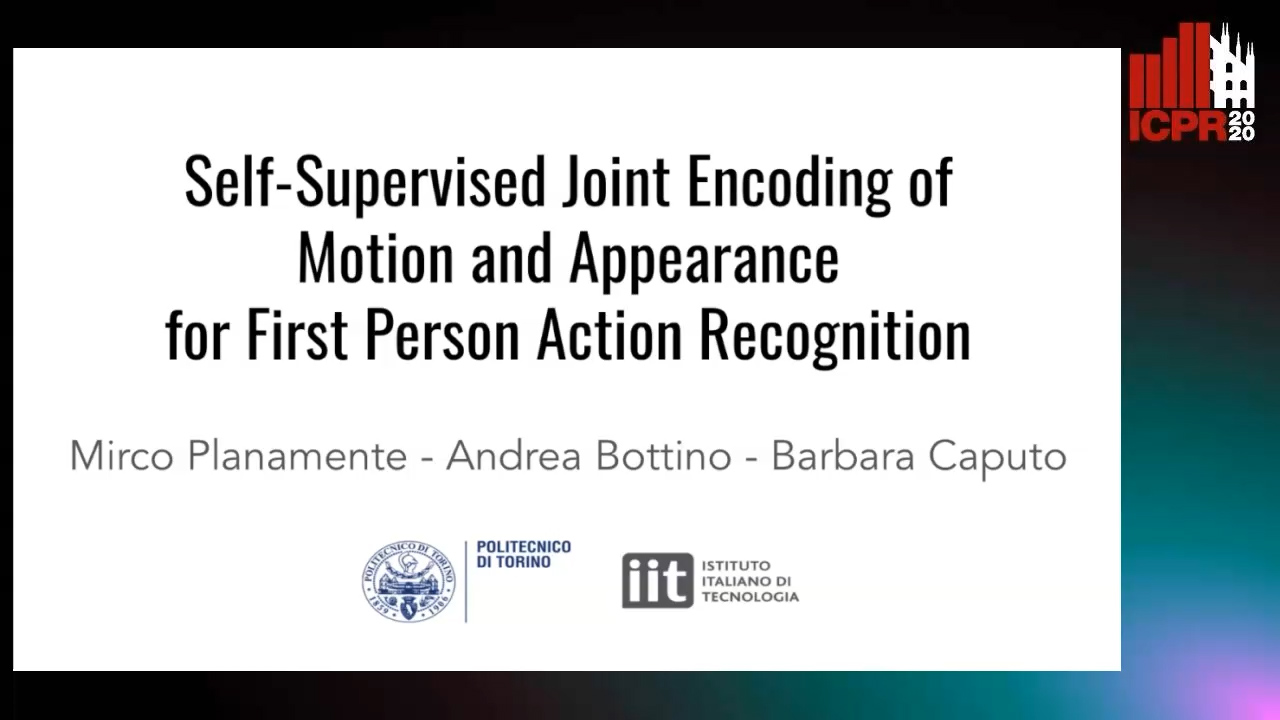
Auto-TLDR; A Single Stream Architecture for Egocentric Action Recognition from the First-Person Point of View
Abstract Slides Poster Similar
Movement-Induced Priors for Deep Stereo
Yuxin Hou, Muhammad Kamran Janjua, Juho Kannala, Arno Solin
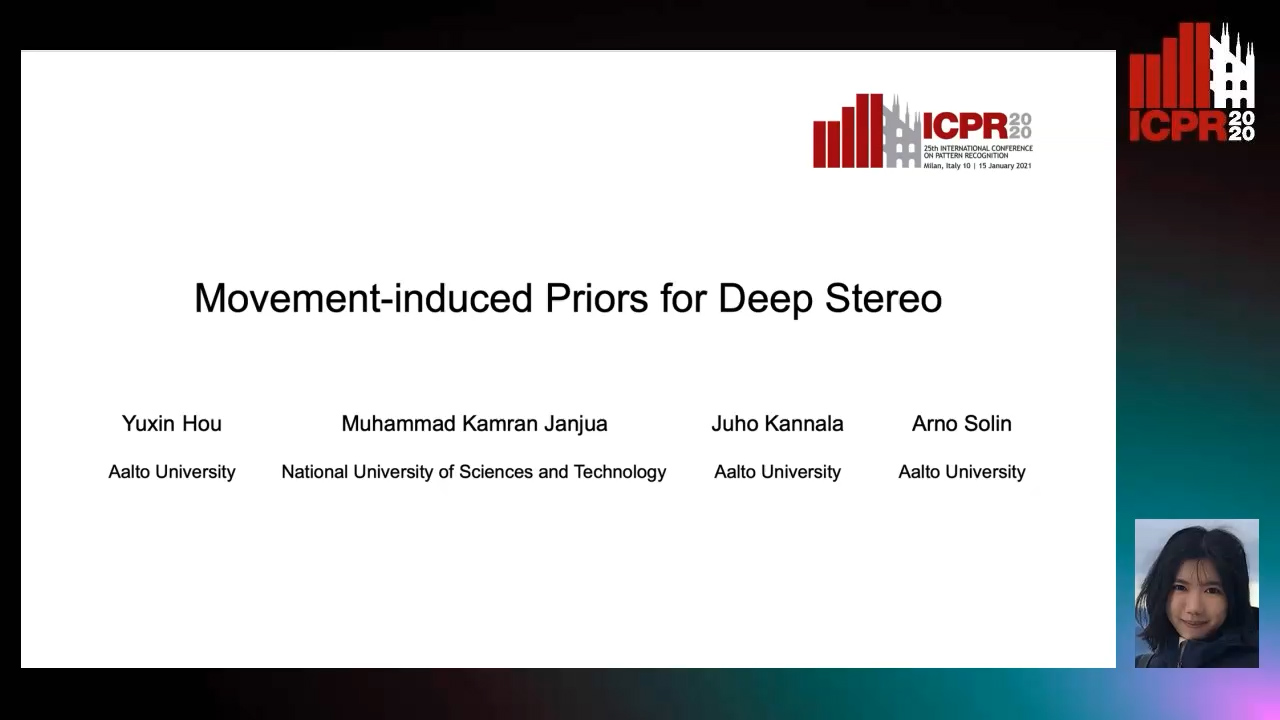
Auto-TLDR; Fusing Stereo Disparity Estimation with Movement-induced Prior Information
Abstract Slides Poster Similar
A Novel Region of Interest Extraction Layer for Instance Segmentation
Leonardo Rossi, Akbar Karimi, Andrea Prati
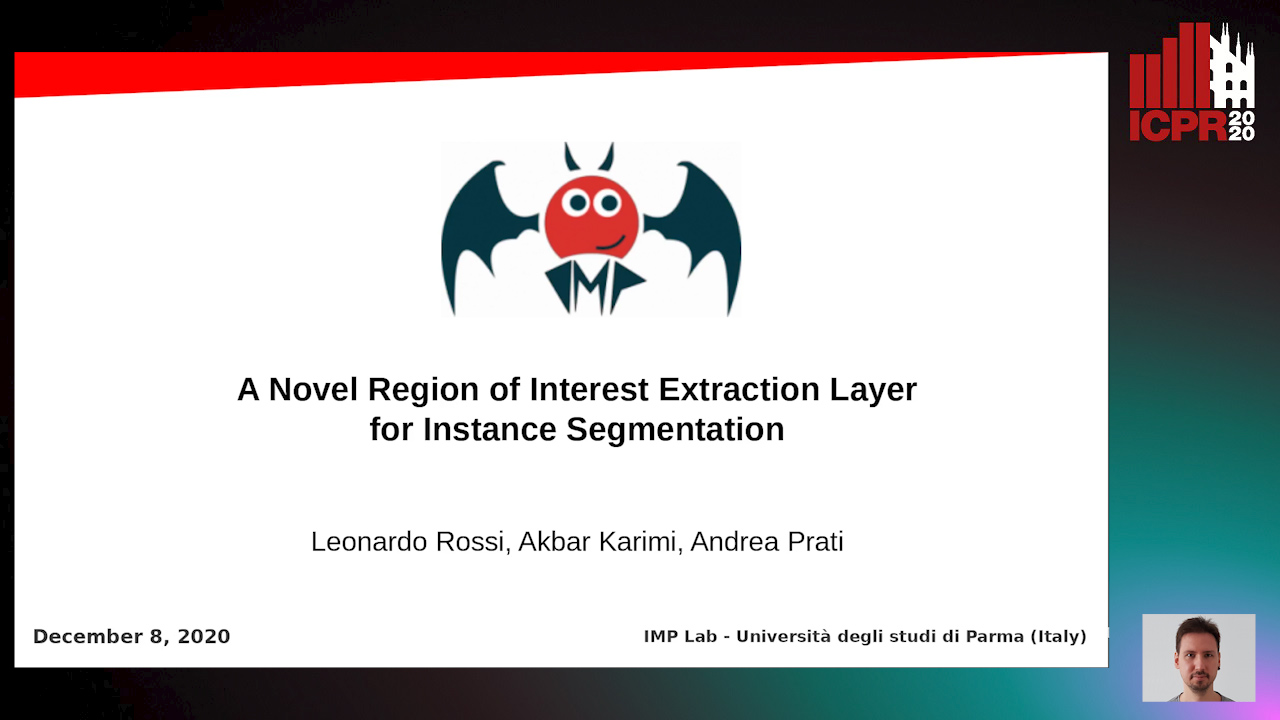
Auto-TLDR; Generic RoI Extractor for Two-Stage Neural Network for Instance Segmentation
Abstract Slides Poster Similar
Space-Time Domain Tensor Neural Networks: An Application on Human Pose Classification
Konstantinos Makantasis, Athanasios Voulodimos, Anastasios Doulamis, Nikolaos Doulamis, Nikolaos Bakalos
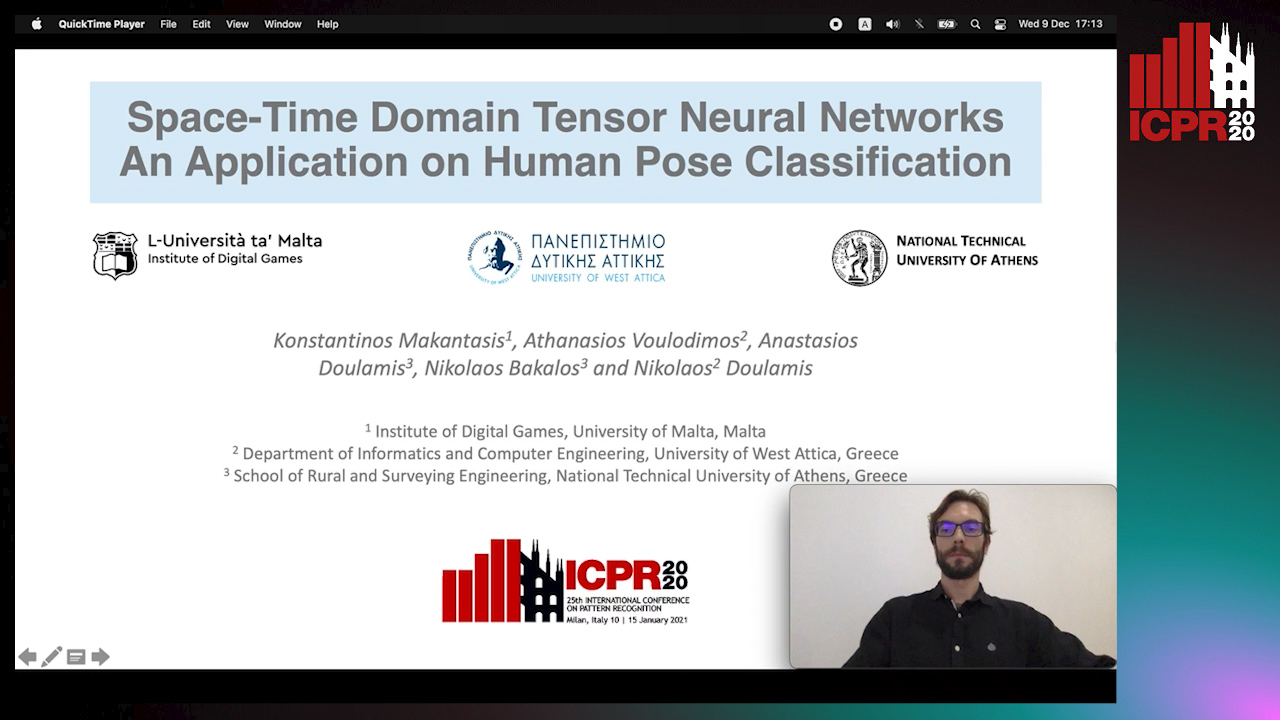
Auto-TLDR; Tensor-Based Neural Network for Spatiotemporal Pose Classifiaction using Three-Dimensional Skeleton Data
Abstract Slides Poster Similar
Joint Face Alignment and 3D Face Reconstruction with Efficient Convolution Neural Networks
Keqiang Li, Huaiyu Wu, Xiuqin Shang, Zhen Shen, Gang Xiong, Xisong Dong, Bin Hu, Fei-Yue Wang
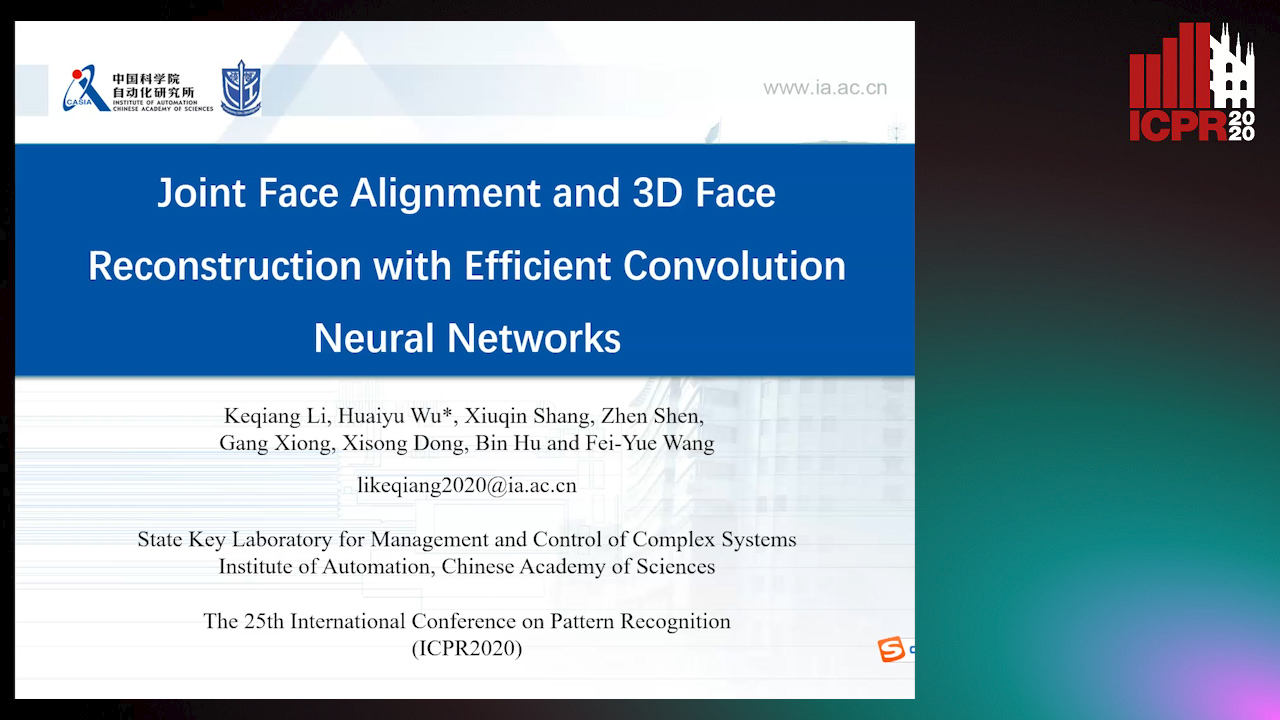
Auto-TLDR; Mobile-FRNet: Efficient 3D Morphable Model Alignment and 3D Face Reconstruction from a Single 2D Facial Image
Abstract Slides Poster Similar
Facetwise Mesh Refinement for Multi-View Stereo
Andrea Romanoni, Matteo Matteucci
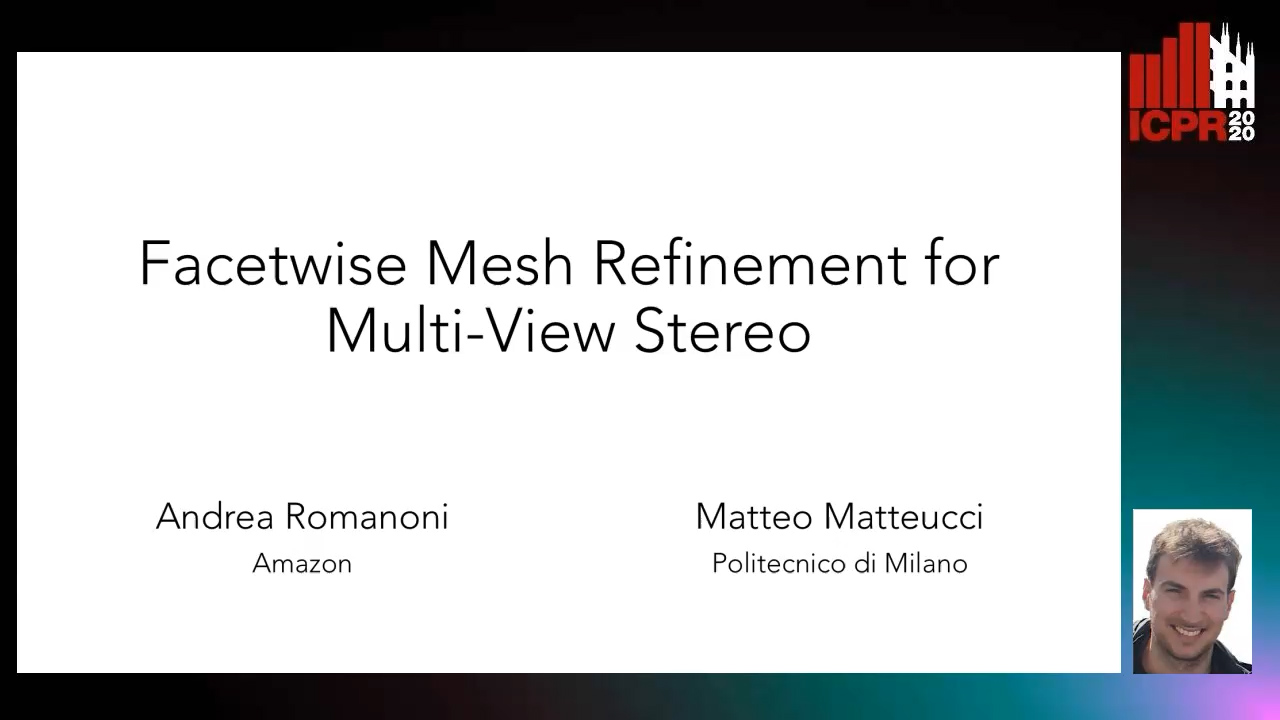
Auto-TLDR; Facetwise Refinement of Multi-View Stereo using Delaunay Triangulations
DmifNet:3D Shape Reconstruction Based on Dynamic Multi-Branch Information Fusion
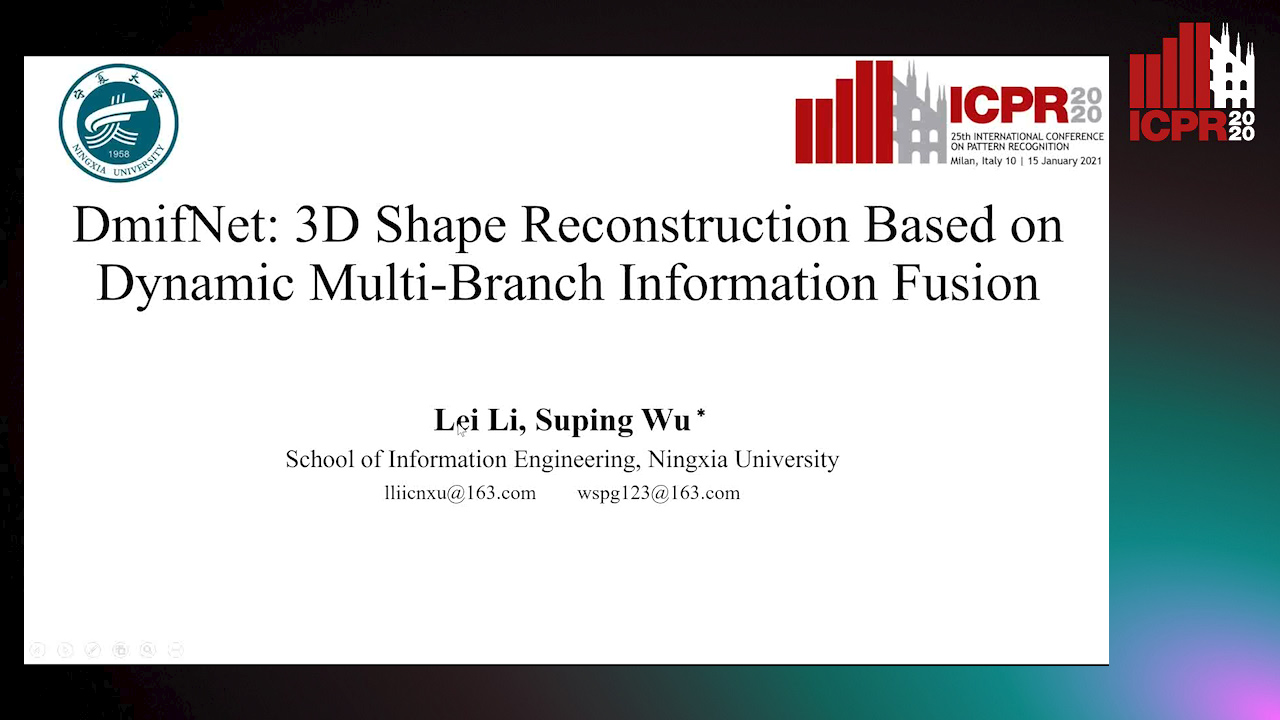
Auto-TLDR; DmifNet: Dynamic Multi-branch Information Fusion Network for 3D Shape Reconstruction from a Single-View Image
Enhancing Deep Semantic Segmentation of RGB-D Data with Entangled Forests
Matteo Terreran, Elia Bonetto, Stefano Ghidoni
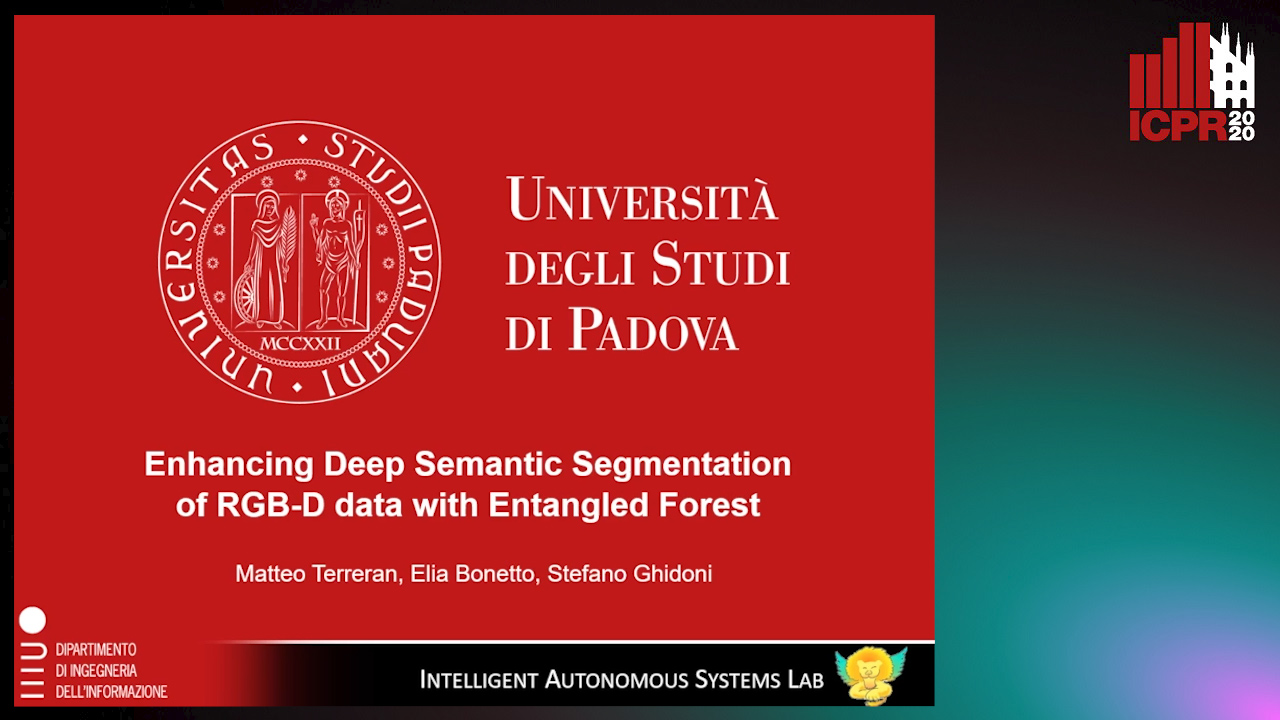
Auto-TLDR; FuseNet: A Lighter Deep Learning Model for Semantic Segmentation
Abstract Slides Poster Similar
PROPEL: Probabilistic Parametric Regression Loss for Convolutional Neural Networks
Muhammad Asad, Rilwan Basaru, S M Masudur Rahman Al Arif, Greg Slabaugh
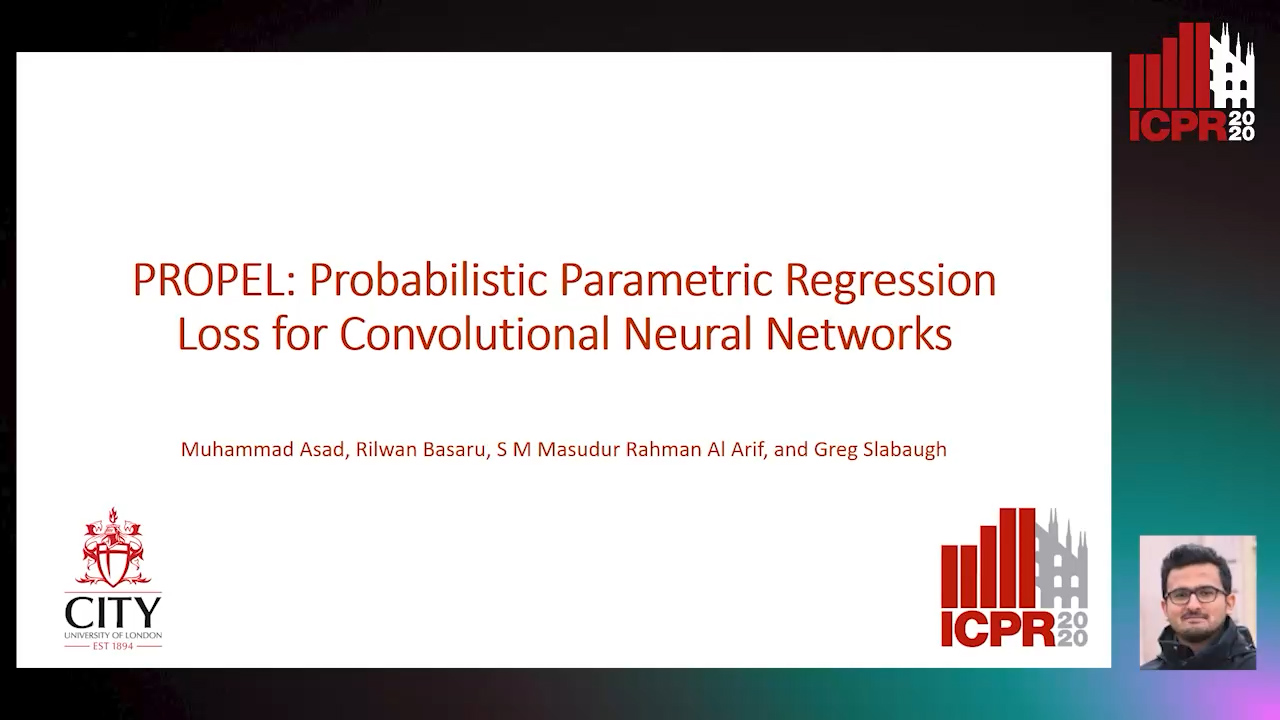
Auto-TLDR; PRObabilistic Parametric rEgression Loss for Probabilistic Regression Using Convolutional Neural Networks
Temporal Binary Representation for Event-Based Action Recognition
Simone Undri Innocenti, Federico Becattini, Federico Pernici, Alberto Del Bimbo
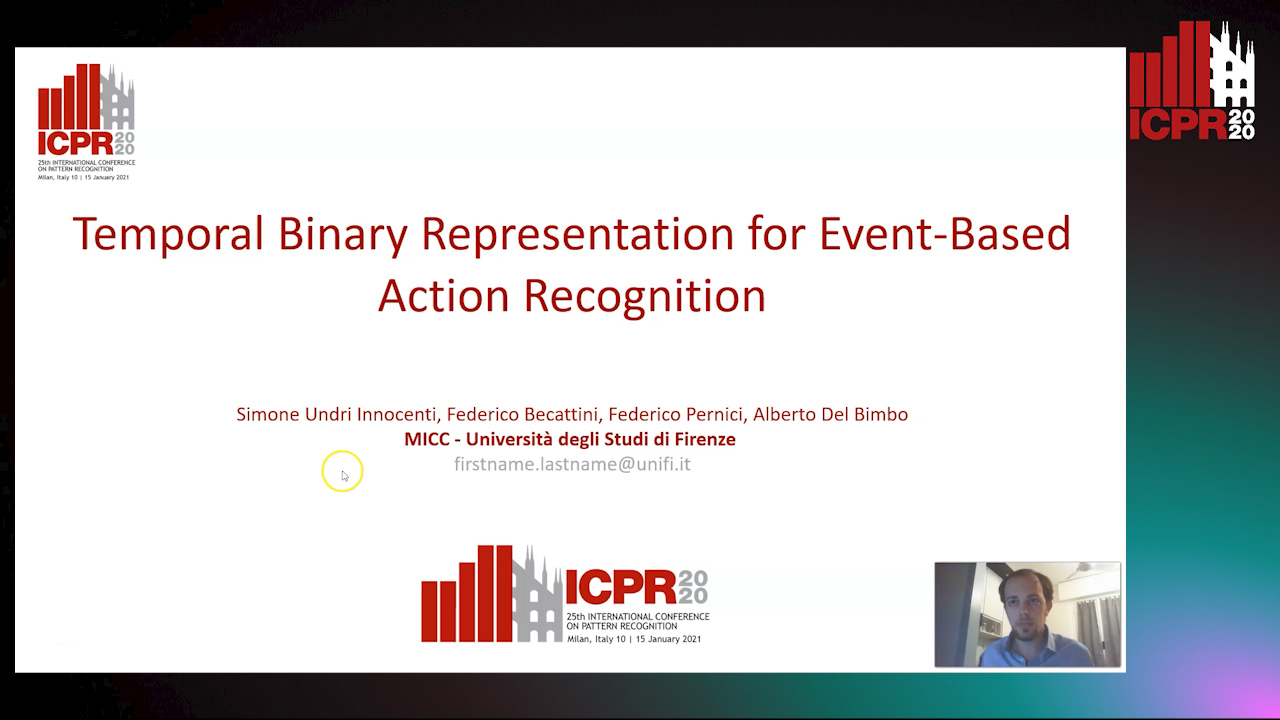
Auto-TLDR; Temporal Binary Representation for Gesture Recognition
Abstract Slides Poster Similar
Benchmarking Cameras for OpenVSLAM Indoors
Kevin Chappellet, Guillaume Caron, Fumio Kanehiro, Ken Sakurada, Abderrahmane Kheddar
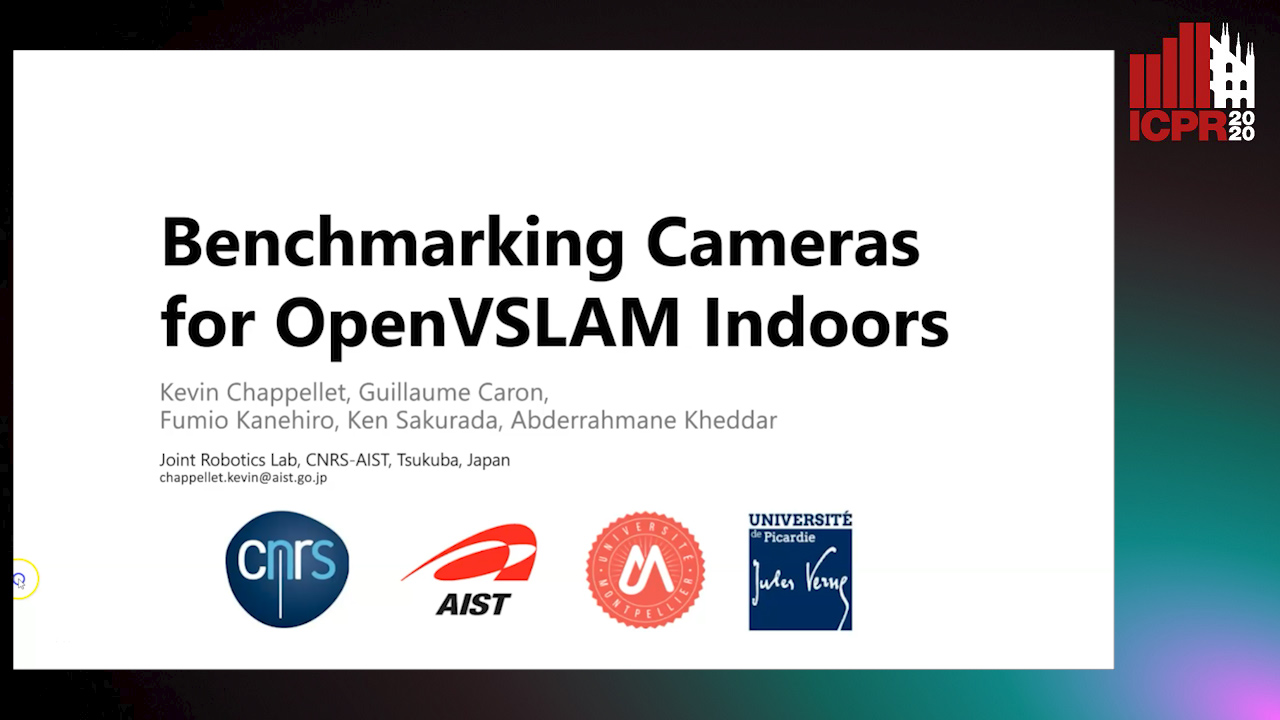
Auto-TLDR; OpenVSLAM: Benchmarking Camera Types for Visual Simultaneous Localization and Mapping
Abstract Slides Poster Similar
Real Time Fencing Move Classification and Detection at Touch Time During a Fencing Match
Cem Ekin Sunal, Chris G. Willcocks, Boguslaw Obara
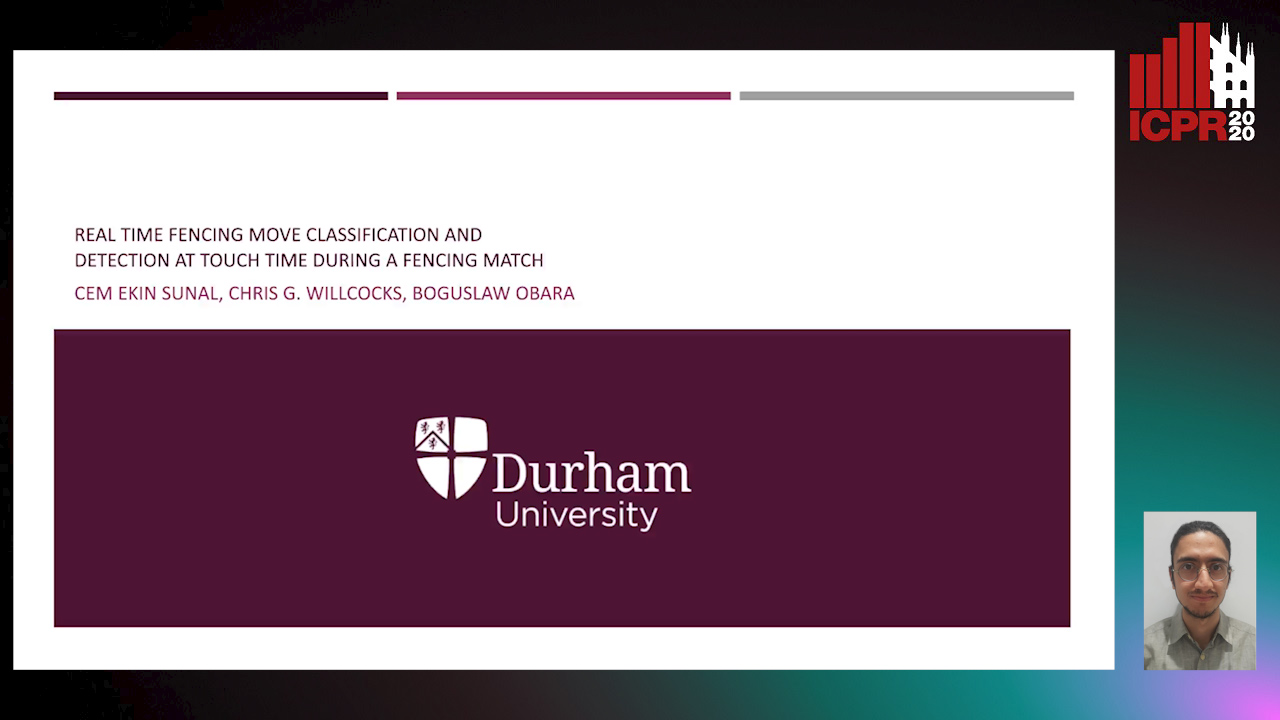
Auto-TLDR; Fencing Body Move Classification and Detection Using Deep Learning
Delivering Meaningful Representation for Monocular Depth Estimation
Doyeon Kim, Donggyu Joo, Junmo Kim
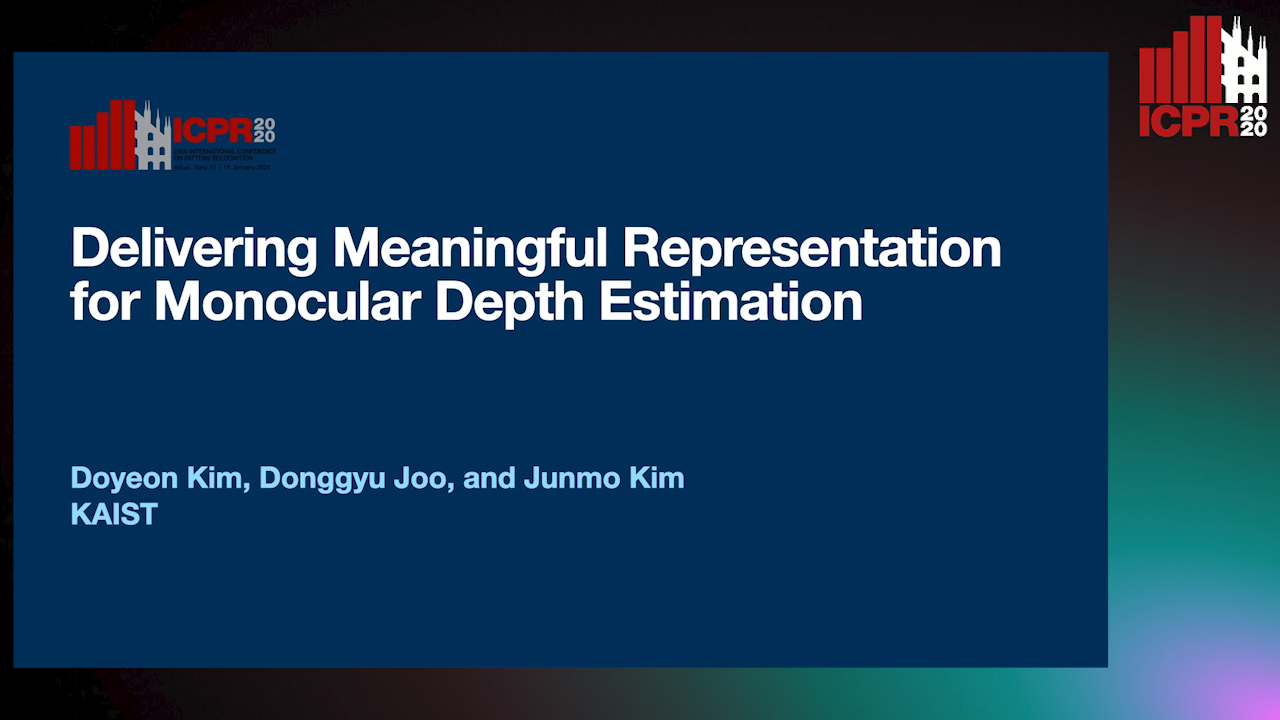
Auto-TLDR; Monocular Depth Estimation by Bridging the Context between Encoding and Decoding
Abstract Slides Poster Similar
Multi-Scale Residual Pyramid Attention Network for Monocular Depth Estimation
Jing Liu, Xiaona Zhang, Zhaoxin Li, Tianlu Mao
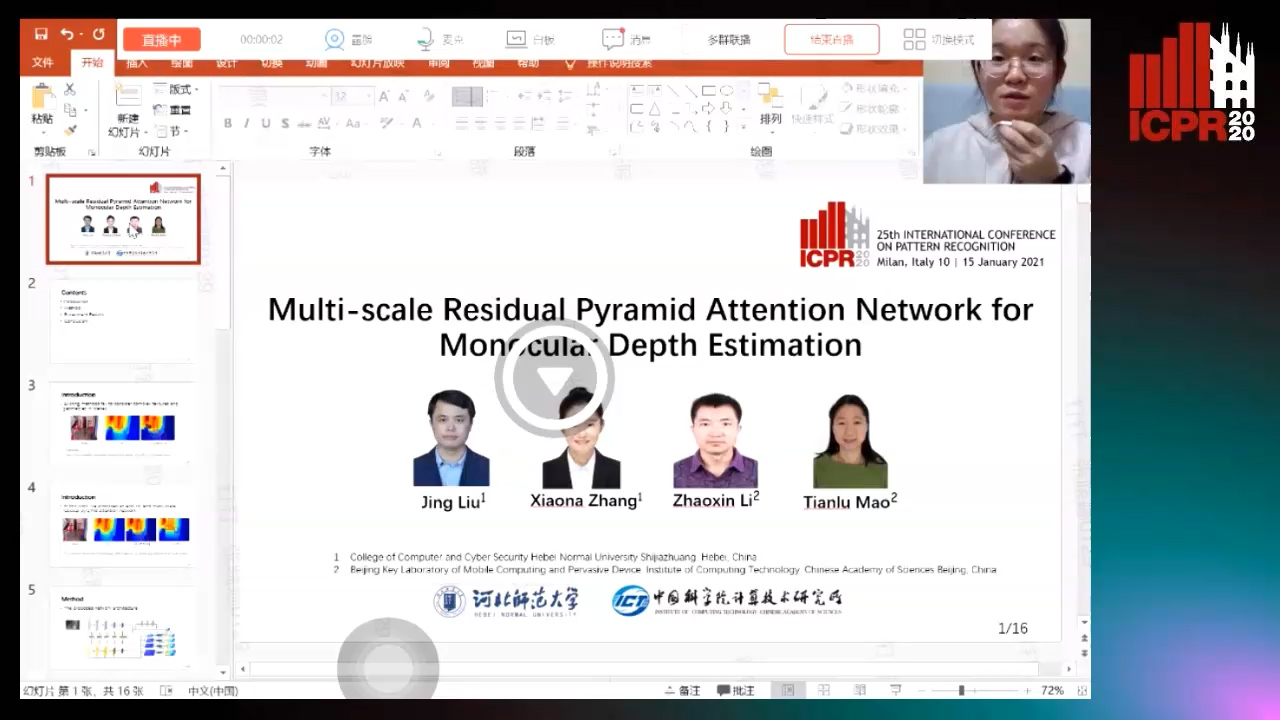
Auto-TLDR; Multi-scale Residual Pyramid Attention Network for Monocular Depth Estimation
Abstract Slides Poster Similar